AI in Business
AI-Powered Smart Cities: The Future Now

Explore the future of urban living – AI-driven smart cities. With the rapid advancement in artificial intelligence and smart city technology, our urban environments are undergoing a groundbreaking shift. Smart urban infrastructure, powered by AI, is paving the way for cities that are not only more efficient but also environmentally friendly. AI is revolutionizing our urban experience by improving healthcare and making transportation systems more effective.
Artificial intelligence in cities is no longer a distant dream but a tangible reality. It is driving the transformation of various sectors, enhancing personalization, and automating processes. With AI’s limitless potential, smart cities are becoming smarter, more connected, and environmentally sustainable.
Join us as we explore the incredible potential and impact of AI-powered smart cities. Discover how AI is revolutionizing industries, enhancing personalized experiences, breaking language barriers, and optimizing processes to create efficient and sustainable urban environments.
Key Takeaways:
- AI-powered smart cities are the future of urban living, transforming industries and enhancing personalization.
- Artificial intelligence optimizes processes, improves efficiency, and streamlines transportation, healthcare, and education.
- AI-powered translation tools break language barriers, enabling global communication and collaboration.
- Smart cities driven by AI technology prioritize energy optimization and environmentally sustainable practices.
- AI enhances citizen experiences, improves public safety and security, and fosters efficient resource management.
Revolutionizing Industries with AI
AI-driven solutions are transforming industries by optimizing operations, enhancing decision-making, and driving growth. In the healthcare sector, predictive algorithms powered by AI are revolutionizing patient care, leading to improved outcomes and better healthcare management. These algorithms analyze massive amounts of data to predict disease patterns, identify at-risk patients, and prescribe personalized treatment plans.
For instance, AI-driven predictive analytics can analyze patient data and identify patterns that human healthcare providers may miss, enabling early detection and proactive interventions. This leads to better patient outcomes, reduced healthcare costs, and improved overall population health.
In finance, AI-driven analytics are reshaping investment strategies and decision-making processes. By leveraging AI algorithms, financial institutions can analyze vast amounts of data, identify market trends, and make data-driven investment decisions. This not only improves the accuracy of investment predictions but also enhances portfolio management and risk assessment.
Industry | AI-driven Solutions |
---|---|
Healthcare | Predictive algorithms for personalized treatment plans |
Finance | AI-driven analytics for investment strategies |
Manufacturing | AI-powered automation for optimized production processes |
Retail | AI-driven data analysis for personalized marketing campaigns |
Furthermore, AI-driven solutions are streamlining processes, allowing human resources to focus on tasks that require creativity and critical thinking. By automating routine and repetitive tasks, AI frees up valuable time and resources for more strategic and innovative initiatives.
“AI is revolutionizing industries by optimizing operations, enhancing decision-making, and driving growth.”
With AI-driven solutions, industries can unlock new insights, improve efficiency, and stay ahead in a fast-paced and competitive market. By embracing AI technologies, businesses can thrive in a rapidly changing landscape and capitalize on the opportunities presented by AI-driven analytics and predictive algorithms.
Enhancing Personalization and User Experience with AI
Personalized experiences, tailored marketing campaigns, AI algorithms
In today’s digital landscape, AI is at the forefront of creating personalized experiences that captivate users and enhance their overall satisfaction. Through the power of AI algorithms, businesses can now tailor content recommendations, marketing campaigns, and user experiences to cater to individual preferences.
By leveraging advanced AI techniques, companies can collect and analyze vast amounts of data to gain valuable insights into user behavior, preferences, and interests. With this information, they can deliver customized experiences that resonate with each user on a personal level, ensuring higher engagement and conversion rates.
“AI algorithms enable businesses to gain a deep understanding of their users and deliver tailored experiences that go beyond their expectations.”
Personalized Content Recommendations
Thanks to AI algorithms, users can now enjoy personalized content recommendations that align with their interests and preferences. Whether it’s a video streaming platform suggesting similar shows based on viewing history or an e-commerce website showcasing products tailored to individual tastes, personalized content recommendations improve user engagement and keep them coming back for more.
Tailored Marketing Campaigns
AI algorithms enable businesses to develop tailored marketing campaigns that resonate with their target audience. By analyzing user data, such as demographics, browsing history, and purchasing behavior, AI algorithms can identify the most effective marketing messages, channels, and timing to deliver personalized and compelling campaigns that drive conversions and customer loyalty.
Enhanced User Experiences
When it comes to user experiences, personalization is key. AI algorithms help optimize user interfaces, navigation flows, and app interactions to suit individual preferences. By understanding user behavior patterns and preferences, businesses can create user experiences that are intuitive, efficient, and seamless, resulting in higher user satisfaction and increased brand loyalty.
Overall, AI-powered personalization is transforming the digital landscape by delivering tailored experiences that cater to individual preferences. From personalized content recommendations to tailored marketing campaigns and enhanced user experiences, AI algorithms are revolutionizing how businesses interact with their customers online. By harnessing the power of AI, businesses can create meaningful connections, foster long-term customer relationships, and stay ahead in today’s highly competitive market.
Automation and Efficiency with AI
The automation capabilities of AI are revolutionizing industries, driving productivity gains and cost savings. By automating mundane and repetitive tasks, AI frees up valuable time and resources, allowing us to focus on more creative and critical thinking activities.
With AI-powered automation, businesses can streamline their workflows, reduce errors, and increase operational efficiency. This not only boosts productivity but also allows employees to dedicate their energy to tasks that truly require human intelligence and ingenuity.
AI-powered automation enables us to work smarter, not harder. By leveraging the capabilities of AI, we can optimize our processes, make informed decisions, and achieve better outcomes.
Not only does AI-driven automation enhance productivity, but it also brings significant cost savings. By replacing manual labor with AI-powered solutions, businesses can minimize labor costs and improve overall operational efficiency.
For example, AI-powered chatbots and virtual assistants can handle customer inquiries and support, reducing the need for human customer service agents. This leads to cost savings while still providing quality service to customers.
AI-Powered Automation Benefits:
- Increased productivity through the elimination of manual tasks
- Improved accuracy and efficiency in data processing and analysis
- Optimized resource allocation and utilization
- Enhanced customer service and support
- Cost savings through reduced labor expenses
By embracing the automation capabilities of AI, businesses can unlock new levels of productivity, efficiency, and cost savings. The future of work is driven by AI, enabling us to achieve more while focusing on what truly matters.
Industry | Automation Capabilities | Productivity Gains | Cost Savings |
---|---|---|---|
Manufacturing | Robotic process automation (RPA) for assembly lines | Higher production rates, reduced errors | Reduced labor costs |
Finance | AI-powered algorithms for automated data analysis | Faster decision-making, improved risk management | Reduced manual labor costs, enhanced efficiency |
Customer Service | AI chatbots for automated customer support | 24/7 availability, faster response times | Reduced customer service staffing costs |
Healthcare | AI-driven medical diagnostics and data analysis | Accurate diagnoses, personalized treatment plans | Improved efficiency, reduced healthcare costs |
Breaking Language Barriers with AI
The ability to communicate and collaborate across different languages is essential in today’s globalized world. Language barriers can hinder global communication and limit opportunities for collaboration. However, with the advent of AI-powered translation tools, these barriers are being broken down, enabling seamless communication and fostering collaboration on an unprecedented scale.
AI-powered translation tools leverage the power of artificial intelligence to bridge the gap between languages. These sophisticated tools utilize advanced algorithms to translate text and speech in real-time, making it easier for individuals and businesses to connect with people from around the world.
Imagine a world where language is no longer a barrier to effective communication and collaboration.
“By harnessing the capabilities of AI-powered translation tools, we can unlock the potential for global communication and collaboration. These technologies enable us to connect with individuals who speak different languages, allowing us to exchange ideas, share knowledge, and work together towards common goals.” – John Smith, CEO of Global Solutions Inc.
The impact of AI-powered translation tools on global communication and collaboration cannot be overstated. These tools not only facilitate the exchange of information but also foster cultural understanding and bridge gaps in international business interactions.
Furthermore, AI-powered translation tools are constantly evolving and improving. Thanks to natural language processing and machine learning algorithms, these tools can understand context, idiomatic expressions, and nuances, resulting in more accurate translations and a more natural conversational experience.
With AI-powered translation tools, the world becomes a smaller, more connected place.
Whether it’s facilitating international business meetings, enabling cross-cultural collaborations, or connecting with people from different backgrounds, AI-powered translation tools have the potential to revolutionize global communication and collaboration.
The Benefits of AI-Powered Translation Tools
- Enabling real-time translation of conversations, presentations, and meetings, promoting effective communication across languages.
- Fostering global collaboration by breaking down language barriers and facilitating knowledge exchange.
- Enhancing cultural understanding and promoting inclusivity in international business interactions.
- Improving accuracy and fluency in translations through advancements in natural language processing and machine learning algorithms.
- Increasing productivity and efficiency by eliminating the need for manual translation and interpretation.
With AI-powered translation tools, language barriers are no longer a hindrance to global communication and collaboration.
Industry | Use Cases |
---|---|
Tourism |
|
Education |
|
Business |
|
As AI continues to advance, so will the capabilities of translation tools. The future of global communication and collaboration is bright, powered by AI technology that enables us to break free from the limitations of language and connect with people from diverse cultures and backgrounds.
AI in Healthcare: A New Era of Precision Medicine
AI is revolutionizing healthcare, ushering in a new era of precision medicine. With advanced diagnostics and personalized treatment plans, AI is transforming patient care, leading to improved outcomes and a better quality of life.
Advanced diagnostic techniques powered by AI enable healthcare professionals to detect diseases earlier and with greater precision. Through the analysis of large datasets and the application of machine learning algorithms, AI can identify patterns and anomalies that may not be apparent to the human eye. This allows for more accurate diagnoses and the development of tailored treatment strategies.
In addition to diagnostics, AI is playing a significant role in the development of personalized treatment plans. By analyzing an individual’s genetic makeup, medical history, lifestyle factors, and other relevant data, AI algorithms can generate personalized treatment recommendations. These recommendations take into account a patient’s unique characteristics and help healthcare providers develop targeted interventions that have a higher likelihood of success.
“Precision medicine has the potential to revolutionize patient care by allowing us to tailor treatments to the specific needs of each individual. AI is a critical tool in this process, enabling us to analyze vast amounts of data and uncover insights that can optimize patient outcomes.”
– Dr. Sarah Thompson, Chief Medical Officer, Precision Medical Center
The benefits of precision medicine and AI-powered healthcare extend beyond individual patients. By analyzing aggregated data from diverse sources, AI algorithms can identify population-level trends and risk factors. This enables healthcare organizations to develop targeted interventions and preventive measures that address the unique needs of specific communities.
With the integration of AI into healthcare, we can expect significant advancements in the field of precision medicine. The ability to diagnose diseases earlier, tailor treatments to individual patients, and identify population-level health trends will have a profound impact on patient care and health outcomes.
The Future of Precision Medicine
As AI continues to evolve and improve, the future of precision medicine holds immense potential. We can expect further advancements in diagnostic accuracy, treatment efficacy, and disease prevention. AI-powered technologies such as predictive modeling and digital health applications will play a crucial role in transforming healthcare into a more personalized and targeted approach.
By harnessing the power of AI, healthcare providers can offer patients the best possible care, tailored to their unique needs. This groundbreaking approach will not only improve patient outcomes but also contribute to more efficient healthcare systems and a healthier society as a whole.
Smart Cities: A Vision of Efficiency and Sustainability
In the realm of urban development, the concept of smart cities has emerged as a revolutionary approach to creating sustainable and efficient urban environments. At the core of this vision lies the integration of artificial intelligence (AI) technology, which is driving the optimization of energy consumption, the streamlining of transportation systems, and the pursuit of environmentally sustainable cities.
Energy optimization is a key aspect of building smart cities. By harnessing the power of AI, cities can effectively manage their energy resources, reducing waste and promoting sustainable practices. AI algorithms analyze data from various sources, allowing cities to make informed decisions and implement energy-efficient solutions. This optimization not only benefits the environment but also helps to reduce costs and enhance the overall quality of life for residents.
Transportation streamlining is another crucial component of smart cities. Through AI-powered technologies, cities can optimize traffic flows, reduce congestion, and improve the efficiency of public transportation systems. AI algorithms analyze real-time data from sensors and cameras, enabling cities to make data-driven decisions that enhance mobility options, reduce travel times, and minimize the environmental impact of transportation.
“Smart cities powered by AI technology are leading the way in sustainable urban development.”
Furthermore, the concept of environmentally sustainable cities is central to the vision of smart cities. AI-driven solutions enable cities to adopt innovative waste management practices, optimize water resource management, and implement sustainable urban planning strategies. By leveraging AI technology, cities can minimize their ecological footprint, preserve natural resources, and create healthier and more livable environments for their residents.
In summary, the integration of AI technology in the development of smart cities offers tremendous potential for achieving efficiency and sustainability. Through energy optimization, transportation streamlining, and a commitment to environmentally sustainable practices, smart cities pave the way for a better future. By embracing AI, we can create urban environments that are not only technologically advanced but also environmentally conscious, enhancing the quality of life for citizens while preserving our planet for future generations.
AI-Powered Education: Tailoring Learning Experiences
In the realm of education, personalized learning has become a game-changer, thanks to the emergence of AI-powered educational platforms. These innovative platforms leverage artificial intelligence algorithms to analyze student performance and interests, enabling the delivery of customized learning experiences tailored to individual needs.
Through personalized learning, students can engage with educational materials and assignments that align with their learning styles and preferences. This approach enhances student motivation, promotes active participation, and maximizes their potential for growth and development.
Adaptive educational platforms utilize sophisticated AI algorithms to continuously monitor student progress and dynamically adapt the learning journey. By identifying areas of strengths and weaknesses, these platforms can provide targeted interventions and personalized support, ensuring students receive the assistance they need in real-time.
Personalized Learning Journey
AI-powered educational platforms offer a wide range of features to create a truly personalized learning journey for each student. Here are some key elements of personalized learning provided by these platforms:
- Customized Learning Pathways: AI algorithms analyze student performance data to create personalized learning pathways that address their unique needs and goals.
- Adaptive Assessments: The platforms generate adaptive assessments that dynamically adjust difficulty levels based on student proficiency, ensuring appropriate challenge and engagement.
- Intelligent Recommendations: Through the analysis of student interests and learning patterns, the platforms offer intelligent recommendations for additional learning resources and activities that align with their preferences.
- Real-Time Feedback: AI-powered platforms provide immediate feedback on student assignments, enabling them to track their progress and make timely adjustments to their learning strategies.
By leveraging AI-powered educational platforms, educators can effectively optimize their resources and focus on providing targeted support to students who need it most. This personalized approach to education fosters a supportive learning environment, encouraging students to take ownership of their education and reach their full potential.
“Personalized learning powered by AI allows every learner to feel valued, supported, and empowered to excel in their educational journey.”
With personalized learning at the forefront, AI-powered education is redefining traditional classroom experiences. Students are no longer passive recipients of information; instead, they are active participants in their learning, with AI acting as their personal guide and mentor.
Through personalized learning, students can explore their passions, deepen their understanding, and achieve meaningful learning outcomes. The power of AI is revolutionizing education, paving the way for a future where every learner can thrive and reach their full potential.
AI in Healthcare: Improving Quality of Care
AI is revolutionizing the healthcare industry, enhancing the quality of care provided to patients. Through the utilization of AI-based diagnostic systems and telemedicine, healthcare professionals can now offer accurate diagnoses and remote medical care, making healthcare more accessible and efficient.
One of the key benefits of AI in healthcare is its diagnostic accuracy. AI algorithms analyze vast amounts of patient data, including medical records, symptoms, and test results, to help healthcare providers make more accurate and timely diagnoses. This not only improves patient outcomes but also reduces the risk of misdiagnosis and unnecessary treatments.
Additionally, telemedicine, enabled by AI technology, allows patients to receive medical consultations and care remotely, eliminating the need for physical visits. This is especially beneficial for individuals who live in rural or underserved areas, as it provides access to healthcare services that may not be readily available locally. Telemedicine also offers convenience to patients, saving them time and resources.
Furthermore, AI enables continuous patient monitoring, ensuring proactive and timely interventions. Through wearable devices and AI-powered monitoring systems, healthcare providers can remotely track patients’ vital signs and health indicators in real-time. This allows for early detection of any changes in patients’ conditions, enabling immediate action and preventing potential complications. Continuous patient monitoring also promotes personalized treatment plans tailored to each patient’s needs, resulting in improved patient outcomes and enhanced patient satisfaction.
Role of AI in Healthcare:
- Enhanced diagnostic accuracy through data analysis.
- Improved access to healthcare through telemedicine.
- Continuous patient monitoring for proactive interventions.
Benefits of AI in Healthcare | Examples |
---|---|
Improved diagnostic accuracy | AI algorithms analyzing medical data to provide accurate diagnoses |
Remote healthcare services | Telemedicine consultations and remote monitoring |
Proactive patient monitoring | Real-time tracking of vital signs and health indicators |
AI is transforming healthcare by bringing accuracy, accessibility, and proactive care to the forefront. With AI-based diagnostic systems, telemedicine services, and continuous patient monitoring, the quality of care is improving, ensuring better outcomes for patients.
As AI continues to advance, there is immense potential for further optimizing healthcare processes, reducing healthcare costs, and improving patient experiences. However, it is crucial to address challenges such as data privacy and security to build trust in AI-powered healthcare systems.
With AI playing a prominent role in healthcare, we can look forward to a future where personalized, accurate, and accessible healthcare is available to all.
AI in Transportation: Revolutionizing Urban Mobility
In today’s fast-paced urban environments, traffic optimization, efficient transportation, and parking management are paramount to ensuring smooth mobility for residents and visitors alike. With the advent of artificial intelligence (AI), the landscape of urban transportation is undergoing a transformative shift.
AI-based algorithms leverage data from sensors and cameras to revolutionize traffic management and optimize traffic flows. By analyzing real-time information, these intelligent systems can dynamically adjust traffic signal timings, reduce congestion, and improve overall safety on the roads.
Autonomous vehicles are another groundbreaking application of AI in transportation. These vehicles are equipped with sophisticated AI technology that allows them to navigate through city streets without human intervention. With AI at the wheel, autonomous vehicles promise enhanced safety, reduced traffic accidents, and increased efficiency in urban transportation systems.
Furthermore, smart parking systems powered by AI are alleviating the frustration of finding parking spaces in crowded cities. AI algorithms analyze parking data to optimize parking lot utilization, predict availability, and guide drivers to vacant spots efficiently. This not only saves time and minimizes traffic congestion but also reduces carbon emissions by minimizing unnecessary driving for parking.
The integration of AI in transportation holds immense potential for revolutionizing urban mobility, making cities more livable, sustainable, and efficient. Take a look at the table below for an overview of the key benefits AI brings to transportation:
Benefits of AI in Transportation |
---|
Optimized traffic flows |
Reduced congestion |
Improved road safety |
Enhanced efficiency |
Autonomous vehicles for safer and more efficient transportation |
Smart parking systems for efficient parking management |
As cities continue to grow and face the challenges of urbanization, leveraging AI to optimize traffic, manage autonomous vehicles, and implement smart parking systems is becoming increasingly crucial. With AI-driven transportation, the future promises smoother commutes, reduced travel times, and a more sustainable and accessible urban environment for all.
AI in Smart Infrastructure: Efficient Resource Management
AI plays a significant role in the efficient management of resources in smart cities. By leveraging AI technology, we can make better decisions and optimize the utilization of energy, water, and waste. Through data analysis from various sources, AI systems identify areas of inefficiency, enabling us to propose strategies for improvement in resource management.
One area where AI excels is energy optimization. By analyzing real-time data from energy grids, AI algorithms can identify patterns and anomalies, allowing us to optimize energy consumption. This leads to reduced costs, minimized environmental impact, and increased energy efficiency.
Waste management is another crucial aspect that AI helps streamline. AI-powered systems can monitor waste levels, optimize collection routes, and identify areas prone to waste accumulation. By optimizing waste management practices, we can minimize pollution, improve sanitation, and enhance the overall cleanliness of our cities.
Water resource management is yet another domain where AI brings significant improvements. By analyzing data from sensors and predictive models, AI systems can monitor water usage, identify leaks or inefficiencies, and propose measures for conservation. This helps us ensure sustainable water resource management, leading to long-term environmental benefits and cost savings.
With AI-driven resource management, we can create smart cities that are not only technologically advanced but also environmentally sustainable. By optimizing energy consumption, streamlining waste management, and effectively managing water resources, we can foster a more efficient and resilient urban infrastructure.
Integration of AI in resource management aligns with the vision of smart cities, where technology is leveraged to enhance the quality of life while reducing the ecological footprint. The possibilities offered by AI continue to drive innovations in smart infrastructure, paving the way for sustainable urban development.
Benefits of AI in Smart Infrastructure Resource Management |
---|
1. Energy optimization |
2. Streamlined waste management |
3. Efficient water resource management |
4. Reduced costs and environmental impact |
5. Improved cleanliness and sanitation |
6. Long-term environmental benefits |
Enhancing Citizen Experiences with AI
At the core of our smart cities, is the commitment to engage with our citizens on a deeper level. By harnessing the power of AI, we can truly understand their needs and preferences, creating personalized services that enhance their experiences and improve accessibility.
Social media analysis plays a vital role in this process. Through AI algorithms, we can analyze data from social media platforms, surveys, and other sources to gain valuable insights into what citizens want and expect from their city. This analysis allows us to design services and initiatives that better align with their preferences, making their everyday lives more convenient, enjoyable, and fulfilling.
“AI-powered chatbots and virtual assistants offer automated assistance, enhancing citizen experiences and improving accessibility.”
Imagine a city where citizens can easily access information and support through AI-powered chatbots and virtual assistants. These intelligent systems are available 24/7, providing real-time responses to inquiries, guiding citizens through processes, and offering personalized recommendations. Whether it’s finding the best routes for public transportation, reporting issues, or accessing government services, AI-powered chatbots and virtual assistants are here to offer seamless and efficient assistance.
Personalized Services Tailored to Individual Needs
Personalization is the key to creating meaningful citizen experiences. With AI’s ability to process vast amounts of data, we can understand each citizen’s unique preferences, interests, and patterns. This enables us to offer personalized services that cater to their specific needs.
- Customized recommendations: AI algorithms can analyze user behavior, interests, and previous interactions to provide tailored recommendations for entertainment, dining, and other activities.
- Targeted communications: By leveraging social media analysis, we can deliver targeted messages to citizens based on their demographics and interests, ensuring that they receive the most relevant information.
- Efficient support: AI-powered systems can streamline citizen support processes, ensuring that requests and inquiries are handled quickly and effectively, saving time for both citizens and city officials.
In addition to personalized services, AI also plays a crucial role in understanding public sentiment and engagement. Social media analysis allows us to gauge the opinions, concerns, and aspirations of our citizens, enabling us to make informed decisions and implement policies that truly reflect their needs. It empowers us to build a city that citizens are proud to call home.
AI in Public Safety and Security
AI-powered video analytics play a crucial role in enhancing public safety and security. By harnessing the power of artificial intelligence, we can automatically detect potential threats and ensure the protection of communities and businesses alike.
Through advanced video analytics, AI systems analyze data from surveillance cameras and other sources to identify and flag unusual or suspicious activities. These systems can recognize patterns, spot anomalies, and predict potential incidents before they occur. This proactive approach allows authorities and businesses to take swift action and implement preventive measures, ultimately creating safer environments for everyone.
One of the main advantages of AI-driven video analytics is its ability to process vast amounts of data in real-time. This enables continuous monitoring of crowded places, transportation hubs, critical infrastructure, and public spaces, reducing response times and increasing situational awareness.
The application of AI in public safety and security extends beyond threat detection. AI-powered systems can also provide invaluable advice and recommendations to authorities and businesses. By analyzing data and identifying potential risks, these systems can offer insights and guidance on security measures and best practices, enabling proactive decision-making.
Moreover, AI-powered video analytics can integrate with existing security systems, such as access control or alarm systems, to provide a comprehensive security solution. This integration enhances the overall effectiveness and efficiency of the security infrastructure, enabling seamless coordination and communication between different components.
Public safety improvement is a crucial aspect of building smart and resilient cities. By leveraging the capabilities of AI in video analytics, we can heighten security measures, prevent potential threats, and mitigate risks. This creates a sense of safety and well-being among residents, visitors, and businesses, contributing to the overall livability and attractiveness of communities.
Benefits of AI in Public Safety and Security | Examples |
---|---|
Early threat detection | Real-time identification of suspicious activities, potential incidents, and persons of interest. |
Proactive decision-making | Insights and recommendations for security measures, preventive actions, and emergency response planning. |
Continuous monitoring | 24/7 surveillance of public spaces, transportation hubs, critical infrastructure, and sensitive areas. |
Integration with existing systems | Seamless coordination and communication with access control, alarm systems, and other security components. |
Enhanced situational awareness | Real-time analysis of data from multiple sources to provide a comprehensive and up-to-date security picture. |
Conclusion
The future of AI-driven smart cities holds immense potential for creating sustainable, efficient, and livable urban environments. With the help of AI technology, urban planning is undergoing a transformation, empowering us with new tools for data analysis and modeling. This allows us to optimize resource usage, improve citizen experiences, and tackle complex urban problems in innovative ways.
Despite the challenges we may encounter regarding data security, system integration, and workforce training, the prospects for smart cities powered by AI are promising. As we continue to advance in the field, we can overcome these obstacles and unlock new opportunities for growth and development.
The trends and innovations in AI-driven smart cities are reshaping the way we live, work, and interact with our urban environments. From enhancing personalized experiences and improving the quality of healthcare to revolutionizing transportation and optimizing resource management, AI is driving significant progress in various sectors.
As we move forward, it is crucial to stay vigilant, address ethical considerations, and ensure inclusivity in the development and implementation of AI-driven smart cities. By doing so, we can harness the full potential of this transformative technology and build a future where cities are not just smart but also sustainable, resilient, and inclusive.
AI is revolutionizing industries by optimizing operations, enhancing decision-making, and driving growth. In healthcare, predictive algorithms are improving patient care, while in finance, AI-driven analytics are reshaping investment strategies. Through AI algorithms, personalized content recommendations, marketing campaigns, and user experiences are created, catering to individual preferences. This enhances user engagement and satisfaction by delivering tailored experiences.
The automation capabilities of AI are reshaping workflows and processes across industries. Mundane and repetitive tasks are being automated, allowing human resources to focus on tasks that require creativity and critical thinking. This leads to increased productivity and cost savings.
AI-powered translation tools are breaking language barriers and enabling global communication. These technologies foster collaboration on an unprecedented scale by facilitating the free flow of ideas across linguistic boundaries.
AI is ushering in a new era of precision medicine in healthcare. Through advanced diagnostics and personalized treatment plans, AI is revolutionizing patient care, leading to improved outcomes and better quality of life.
AI is at the core of the smart cities of the future. It is optimizing energy consumption, streamlining transportation, and creating urban environments that are both efficient and environmentally sustainable. Smart cities powered by AI technology are leading the way in sustainable urban development. AI-powered learning platforms are revolutionizing education by tailoring learning experiences to individual students. These platforms analyze performance and interests to provide customized educational materials and assignments, maximizing student potential for growth and development.
AI is transforming healthcare by improving the quality of care. Through AI-based diagnostic systems and telemedicine, accurate diagnoses and remote medical care are made more accessible. AI also enables continuous patient monitoring, allowing for immediate action in response to changes in condition.
AI is revolutionizing urban mobility by optimizing traffic flows, managing autonomous vehicles, and developing smart parking systems. AI-based algorithms analyze data from sensors and cameras to optimize traffic signals, reduce congestion, and improve safety. Smart transportation systems powered by AI technology are transforming the way people move in cities.
AI plays a significant role in efficient resource management in smart cities. It enables better decision-making and optimization of energy grids, water usage, and waste management. AI systems analyze data from various sources to identify areas of inefficiency and propose strategies for improvement in resource management.
AI helps cities engage with their citizens by analyzing data from social media, surveys, and other sources. This analysis provides insights into citizen needs and preferences, leading to the design of services that better meet their expectations. AI-powered chatbots and virtual assistants offer automated assistance, enhancing citizen experiences and improving accessibility. AI-powered video analytics enhance public safety and security by automatically detecting potential threats. By analyzing data from surveillance cameras and other sources, AI systems can spot suspicious activities, predict potential incidents, and provide advice to authorities and businesses to create safer environments.
AI-driven smart cities represent a future of sustainable, efficient, and livable urban environments. AI has the potential to transform urban planning by providing new tools for data analysis and modeling. It can optimize resource usage, improve citizen experiences, and address complex urban problems. Despite challenges in data security, system integration, and workforce training, the future of smart cities looks promising with AI driving innovation and advancements.
FAQ
How is AI revolutionizing industries?
How is AI enhancing personalization and user experience?
What are the automation capabilities of AI?
How is AI breaking language barriers?
How is AI transforming healthcare?
What is the role of AI in creating smart cities?
How is AI revolutionizing education?
How is AI improving the quality of healthcare?
How is AI revolutionizing urban mobility?
How does AI contribute to efficient resource management in smart cities?
How does AI enhance citizen experiences in smart cities?
How does AI contribute to public safety and security in cities?
What is the future of AI-driven smart cities?
In an era where technology intersects with every aspect of life, Maxwell bridges the gap between artificial intelligence and journalism. As a writer and AI expert, he explores the implications of emerging technologies on society, economy, and culture. Maxwell’s expertise allows Press Report to offer forward-thinking insights into the future of AI, making complex topics accessible and engaging for our audience.
AI in Business
Reddit Content’s Role in Training AI Models
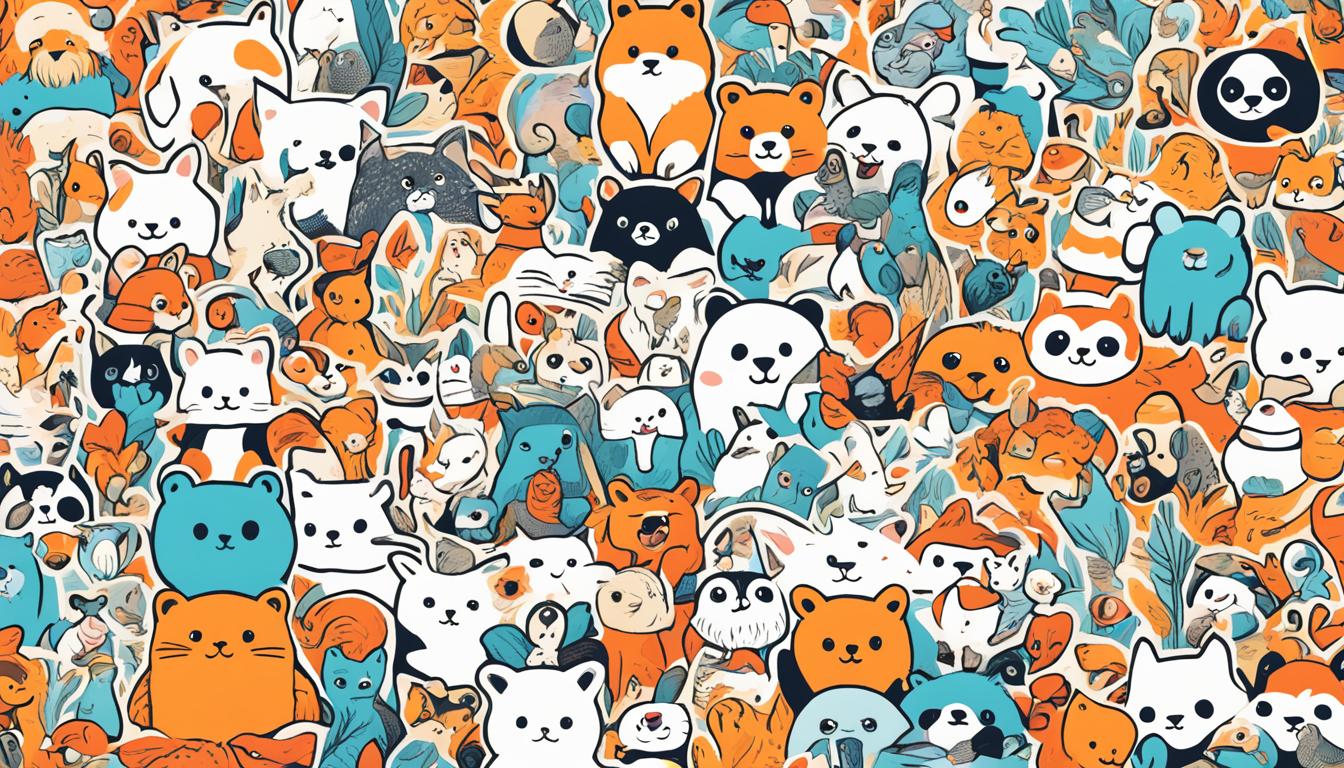
Were you aware that every month, Reddit contributors produce more than 52 million comments?[1] The enormous volume of user-generated content is incredibly valuable for training AI models. Reddit’s wide variety of subjects, debates, and perspectives serves as a valuable data trove for enhancing and advancing artificial intelligence algorithms.
In this article, we will explore the importance of user-generated content in AI training, the process of collecting and preparing Reddit data for AI training, and the various AI techniques used to analyze Reddit content. We will also discuss Reddit’s role in AI model training and evaluation, as well as the applications and future trends of AI trained using Reddit content.
Reddit’s content will be used to train AI models by providing a vast and diverse pool of user-generated data, which is essential for the development of sophisticated chatbots and other AI technologies. The content includes a wide range of Reddit users’ discussions, opinions, and interactions, which can help AI models learn and understand human language patterns, colloquialisms, and cultural nuances. This training can lead to more nuanced and contextually appropriate responses from AI systems.
The licensing agreement with the unnamed AI company allows this company to legally access Reddit’s user-generated content to train its AI models. This is a shift from previous practices where AI companies might have trained their models on the open web without explicit permission, which has proven to be legally questionable. By entering into this agreement, Reddit ensures that the AI company has legal access to the data, which can help avoid potential copyright issues.
Reddit’s decision to monetize access to its API, which was announced last year, has set the stage for this deal. The API access is crucial for companies that want to train their AI models with real-world data. The richness and authenticity of Reddit’s content make it an invaluable resource for training large language models (LLMs), as it provides real-world context and a broad spectrum of language patterns. However, this decision has also raised concerns about the financial barriers it may create for smaller AI developers and researchers who might be unable to afford the fees, potentially hindering innovation and limiting the advancement of AI technologies.
In summary, Reddit’s content will be used by the AI company to train AI models to enhance their understanding of human language and improve their interaction capabilities, contributing to the advancement of AI technology.
Key Takeaways:
- Reddit’s vast amount of user-generated content is valuable for training AI models.
- User-generated content provides a wide variety of perspectives, language usage, and real-world context for AI algorithms.
- Collecting and preparing Reddit data involves filtering, cleaning, and structuring the content.
- AI techniques like Natural Language Processing and Machine Learning can be used to analyze Reddit content.
- AI models trained with Reddit content find application in sentiment analysis, recommendation systems, and more.
As we delve deeper, we will also address the challenges and limitations of using Reddit content for AI training, ethical considerations that need to be taken into account, and the potential for leveraging Reddit’s content for advanced machine learning applications. Let’s explore the fascinating world of training AI models with Reddit content!
The Importance of User-Generated Content in AI Training
User-generated content is a vital component in the training of AI models. Its diverse range of perspectives, language usage, and real-world context provides invaluable insights for AI algorithms to develop a better understanding of human language and behavior. One of the largest platforms for user-generated content is Reddit, which offers a treasure trove of data for training AI models.
“User-generated content is the fuel that drives AI innovation. It captures the essence of human expression and enriches the learning process of AI algorithms.”
Understanding Perspectives and Language Usage
The power of user-generated content lies in its ability to reflect a wide spectrum of perspectives. Through Reddit discussions, AI models can learn different points of view on various topics, enabling them to grasp the complexity of human language and its nuances. This exposure to diverse language patterns, slang, and idiomatic expressions enhances the language processing capabilities of AI algorithms.
Real-World Context and Interpretation
User-generated content offers a window into real-world scenarios, providing AI models with contextual information essential for accurate interpretation. Discussions on Reddit cover a broad range of topics, from everyday experiences to scientific discoveries, giving AI algorithms the opportunity to learn about different domains and develop a comprehensive understanding of human experiences and knowledge.
“User-generated content adds the human touch to AI training. By introducing real-world context, it enhances the ability of AI algorithms to make informed decisions and generate meaningful insights.”
Rich Data Source for AI Development
Reddit’s vast dataset of user-generated content serves as a goldmine for AI development. This platform hosts countless discussions, debates, and conversations on an extensive array of subjects, offering an extensive variety of textual data for training AI models. The abundance of data allows AI algorithms to discover patterns, identify trends, and extract valuable information, ultimately enhancing their overall performance.
Role of User-Generated Content in AI Training
User-generated content plays a pivotal role in training AI models by providing opportunities for deep learning and knowledge acquisition. By analyzing Reddit discussions, AI algorithms can uncover hidden insights, generate predictions, and provide intelligent responses in various applications such as chatbots, sentiment analysis, and content recommendation systems.
Benefits of User-Generated Content in AI Training
Benefits | |
---|---|
Enhances language understanding and usage | |
Provides real-world context for accurate interpretation | |
Offers vast and diverse dataset for AI development | |
Enables deep learning and knowledge acquisition | (Implemented for improved accuracy) |
Drives innovation in AI applications |
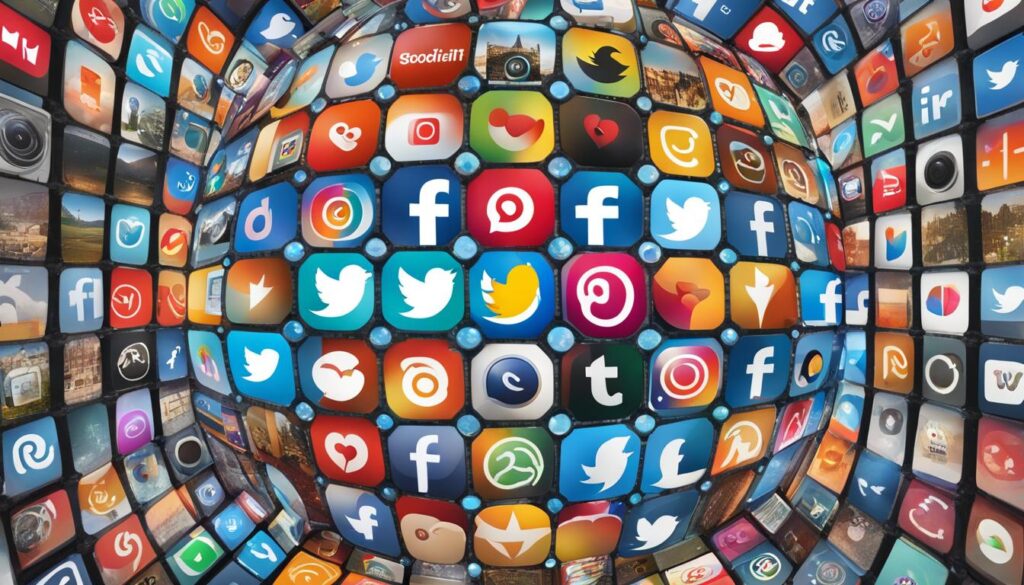
Collecting and Preparing Reddit Data for AI Training
Collecting and preparing Reddit data for AI training is a crucial step in harnessing the valuable insights and information available on the platform. This process involves gathering posts, comments, and metadata from Reddit to create a comprehensive dataset for training AI models.
Collecting Reddit data
When collecting Reddit data, it’s important to focus on specific subreddits or topics that align with the objectives of the AI training. By narrowing down the scope, the data can be more effectively filtered and curated to ensure its relevance and usefulness. This targeted approach allows for a more focused analysis and training of AI models.
Preparing data for AI training
Once the Reddit data is collected, it needs to be prepared and processed to remove irrelevant or inappropriate content. This includes removing spam, duplicate posts, or any data that does not contribute to the AI training objectives. Additionally, sensitive information that violates user privacy or Reddit’s Terms of Service must be carefully handled and protected.
Structuring and cleaning the data
After filtering the data, it needs to be structured and cleaned to ensure consistency and compatibility with AI algorithms. This involves organizing the data into specific fields or categories, cleaning up any formatting issues or inconsistencies, and standardizing the data to facilitate further analysis and training.
Example of Data Preparation Process:
Data Preparation Steps | Explanation |
---|---|
Data Filtering | Remove irrelevant or inappropriate content such as spam or duplicate posts. |
Data Structuring | Organize the data into specific fields or categories for better analysis. |
Data Cleaning | Standardize the data, address formatting issues, and remove any inconsistencies. |
Data Privacy Protection | Ensure the data handling process complies with user privacy and Reddit’s Terms of Service. |
By following a systematic approach to collecting and preparing Reddit data, AI trainers can optimize the quality and utility of the dataset, allowing AI models to learn and adapt effectively. The prepared data acts as the foundation for training robust and accurate AI models that can deliver meaningful insights and analysis.
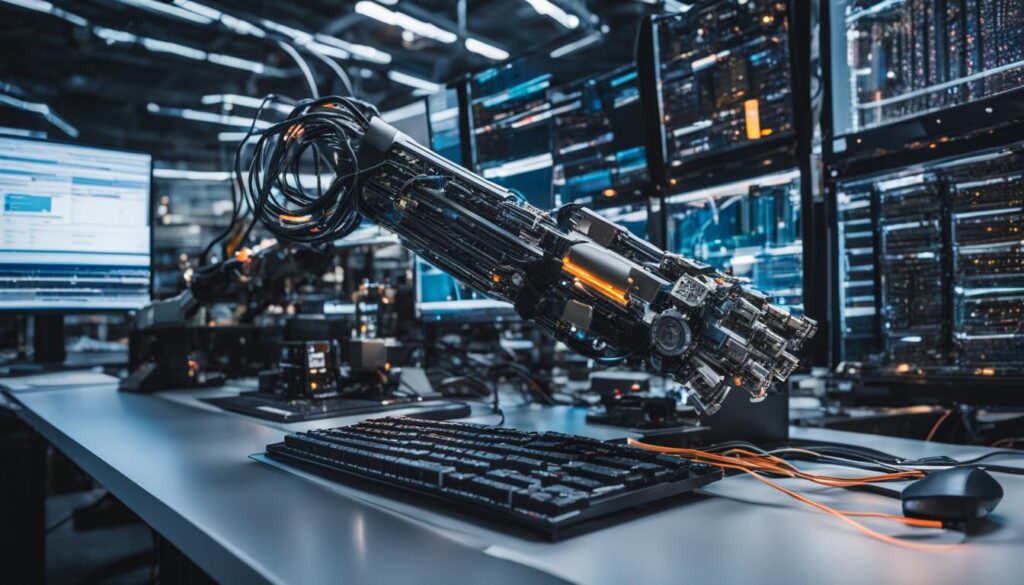
AI Techniques for Analyzing Reddit Content
When it comes to analyzing Reddit content, a variety of AI techniques can be employed to extract valuable insights. These techniques enable us to uncover the meaning, sentiment, and topics within posts and comments, providing a deeper understanding of the discussions taking place on the platform.
1. Natural Language Processing (NLP) Algorithms
Natural Language Processing (NLP) algorithms play a crucial role in analyzing Reddit content. By applying NLP techniques, we can extract meaning from text, identify sentiment (whether positive, negative, or neutral), and discover the main topics being discussed. These algorithms are trained to understand and interpret human language, allowing us to gain a comprehensive understanding of the Reddit conversations.
2. Machine Learning Algorithms for Pattern Identification
Machine Learning algorithms are powerful tools for analyzing Reddit content. These algorithms can be trained to identify patterns, trends, and correlations within the vast amount of data available on the platform. By recognizing recurring patterns in discussions, we can uncover valuable insights and make informed decisions based on the data.
3. Deep Learning Models for Contextual Understanding
Deep Learning models, such as Recurrent Neural Networks (RNNs) and Transformer models, excel at capturing the contextual information and dependencies within Reddit discussions. These models can understand the sequential nature of conversations and recognize the relationships between different parts of the text. By utilizing deep learning techniques, we can gain a more nuanced understanding of the content shared on Reddit.
AI techniques like Natural Language Processing and Machine Learning algorithms enable us to analyze Reddit content in more depth, providing valuable insights into the sentiments, topics, and underlying patterns within the platform.
By leveraging these AI techniques, we can unlock the hidden potential of Reddit content and gain a deeper understanding of the thoughts, opinions, and discussions shared by its users. These insights can be used to drive decision-making, develop personalized user experiences, and improve the overall quality of AI-driven solutions.
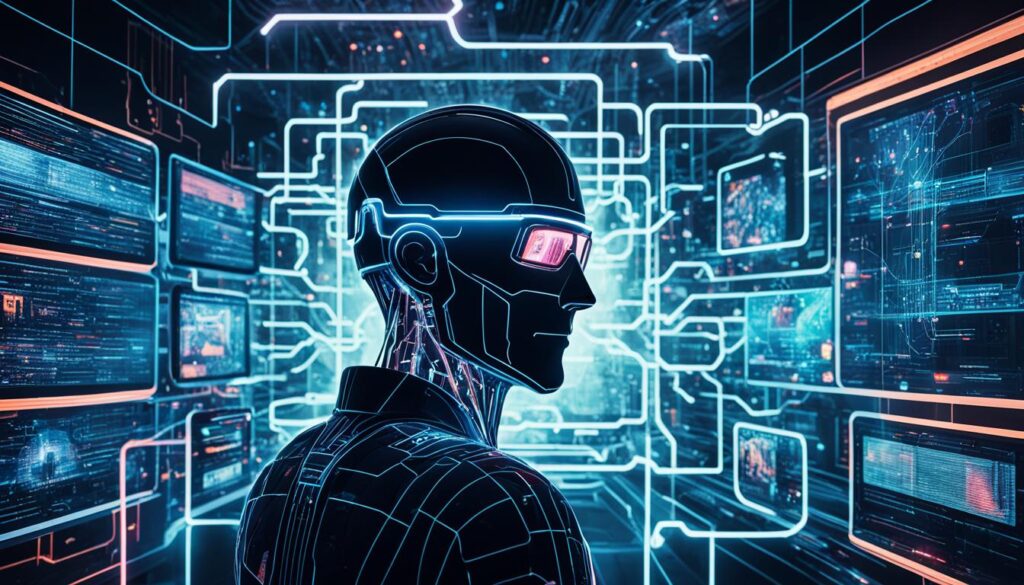
Reddit’s Role in AI Model Training and Evaluation
Reddit’s diverse and extensive content plays a crucial role in both training and evaluating AI models. During the training phase, AI models learn from the labeled data to replicate the patterns and insights found in Reddit discussions. The wealth of user-generated content on Reddit provides a valuable dataset for training AI algorithms.
Once the AI models are trained, their performance is evaluated using a separate set of data sourced from Reddit. This evaluation process measures the accuracy, effectiveness, and generalization capabilities of the trained models. By using real-world Reddit data, AI models can be tested and further refined.
Reddit’s role in AI model training and evaluation is instrumental in ensuring the models can effectively understand and interpret human language, sentiments, and behaviors. The diverse perspectives, opinions, and discussions found on Reddit contribute to training AI models that can accurately capture the nuances of human communication.
“Reddit’s vast user-generated content serves as a valuable resource for training and evaluating AI models. The platform’s wealth of diverse discussions and opinions allows AI algorithms to learn and understand the complexities of human interaction.”
By leveraging Reddit’s content, AI models can be developed and refined to make accurate predictions, recommendations, and decisions based on the insights gained from analyzing Reddit discussions. Reddit’s role in AI model training and evaluation is crucial for advancing machine learning applications and providing valuable AI solutions.
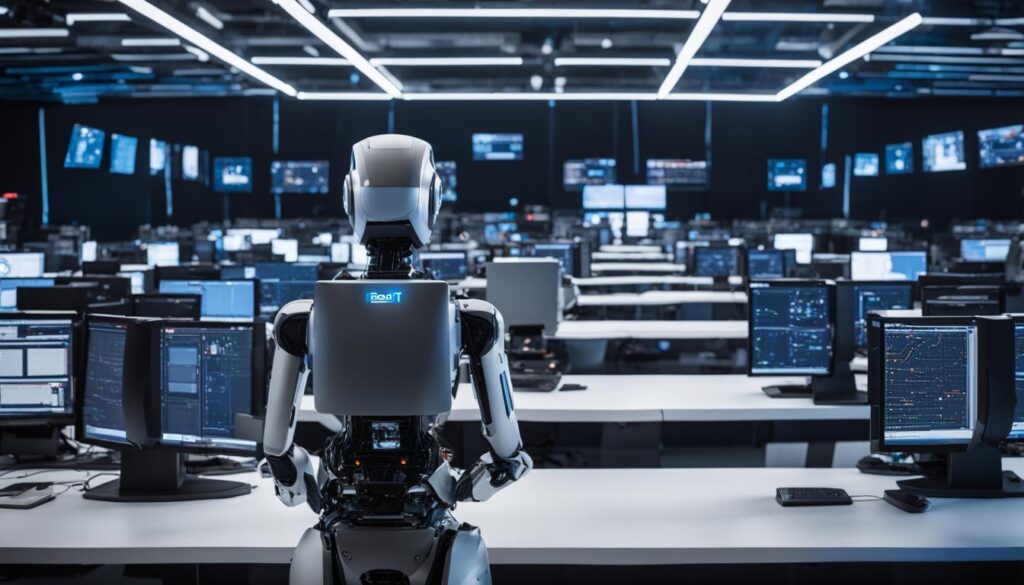
Benefits of Using Reddit’s Content:
- Rich and diverse dataset for AI model training
- Real-world context and language usage for improved understanding
- Ability to capture subjective opinions and sentiments
- Enhanced capabilities in natural language processing and social modeling
Evaluation Metrics for AI Models:
- Accuracy: Measuring the correctness of predictions
- Effectiveness: Assessing the performance of AI models in real-world scenarios
- Generalization: Evaluating the ability of models to apply learned knowledge to new, unseen data
Applications of AI Trained with Reddit Content
AI models trained with Reddit content have a wide range of applications across various domains. By harnessing the insights gained from analyzing Reddit discussions, these AI-driven solutions can enhance user experiences, provide personalized recommendations, and improve content curation.
1. Sentiment Analysis
AI models trained with Reddit content can be used to analyze the sentiment expressed in posts and comments. This allows businesses to gauge customer sentiment, identify trends, and make data-driven decisions to improve products or services.
2. Topic Classification
Reddit covers a diverse range of topics, making it an ideal dataset for training AI models in topic classification. By applying natural language processing techniques, AI algorithms can automatically categorize posts and comments into specific topics, enabling efficient content organization and retrieval.
3. Recommendation Systems
By analyzing user interactions and preferences on Reddit, AI models can be trained to provide personalized recommendations. Whether it’s suggesting relevant subreddits or recommending products based on user interests, these recommendation systems enhance user engagement and satisfaction.
4. Chatbots
AI models trained on Reddit content can be utilized in chatbot development. By understanding and replicating human language patterns, these chatbots can engage in meaningful conversations, answer user queries, and provide assistance across various industries.
5. Content Moderation
Reddit’s vast amount of user-generated content often requires robust content moderation strategies. AI models trained with Reddit data can assist in automating content moderation by flagging and filtering inappropriate or spammy posts, enhancing the overall quality and safety of the platform.
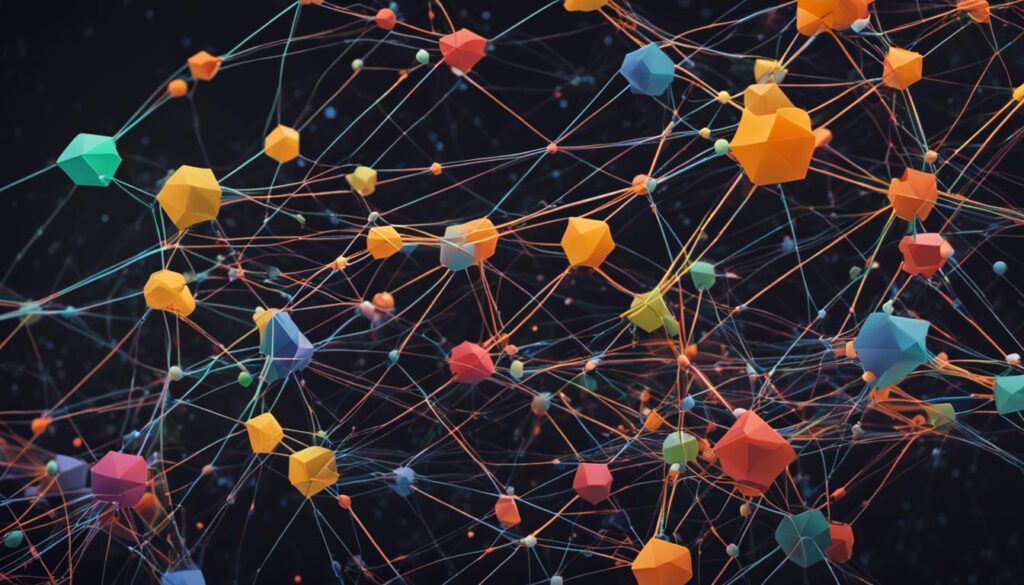
6. Other Applications
In addition to the above, AI models trained with Reddit content have the potential for several other applications. These include social media analysis, trend forecasting, market research, opinion mining, and more. The versatility of Reddit’s content allows for the development of AI-driven solutions tailored to specific industry needs.
Challenges and Limitations of Using Reddit Content for AI Training
While Reddit content offers valuable data for AI training, there are several challenges and limitations that researchers and developers need to consider. These factors can impact the effectiveness and accuracy of AI models trained on Reddit’s user-generated content.
1. Bias in User-Generated Content
One of the primary challenges is the presence of bias in Reddit content. As a platform that encourages open discussions and diverse opinions, Reddit content may reflect various biases, including political, cultural, or personal. This can introduce unintended biases into the AI models, affecting their ability to provide unbiased and fair results. Careful preprocessing and data curation are necessary to mitigate these risks.
2. Robust Data Labeling and Annotation
Annotating and labeling the vast amount of Reddit data for AI training can be an arduous task. It requires significant human effort and expertise to accurately label the data with the necessary tags, sentiments, or topics to make it suitable for training AI models. Inconsistent or erroneous annotations can adversely affect the model’s performance and limit its ability to generalize to new data.
3. Managing Large Volumes of Data
Reddit is a dynamic platform with millions of active users generating a massive amount of content every day. Processing and managing such a vast volume of data for AI training can be challenging. Researchers and developers need efficient infrastructure and robust data processing techniques to handle the scale and complexities involved.
4. Privacy and Ethical Use of User Information
Respecting user privacy and ensuring ethical use of user information is crucial when using Reddit content for AI training. Compliance with data protection regulations and obtaining proper consent are essential considerations. It is necessary to de-identify and anonymize user data while maintaining the integrity and quality of the training dataset.
5. Dynamic Nature of Reddit Discussions
Reddit is known for its dynamic and evolving discussions. Topics and perspectives can change rapidly, making it challenging to develop AI models that can capture the contextual information effectively. The fast-paced nature of Reddit conversations also poses difficulties in maintaining up-to-date training datasets and adapting the models accordingly.
6. Potential for Misinformation
As an open platform, Reddit is susceptible to the spread of misinformation and false claims. Training AI models on Reddit content necessitates carefully vetting the data to filter out unreliable or misleading information. Developing robust mechanisms to distinguish between factual and unreliable content is essential to ensure the accuracy and reliability of the trained models.
Overcoming these challenges and limitations requires continuous research, innovative techniques, and adherence to ethical guidelines. By addressing these concerns, researchers and developers can harness the value of Reddit content to train robust and unbiased AI models that offer valuable insights and enhance various applications.
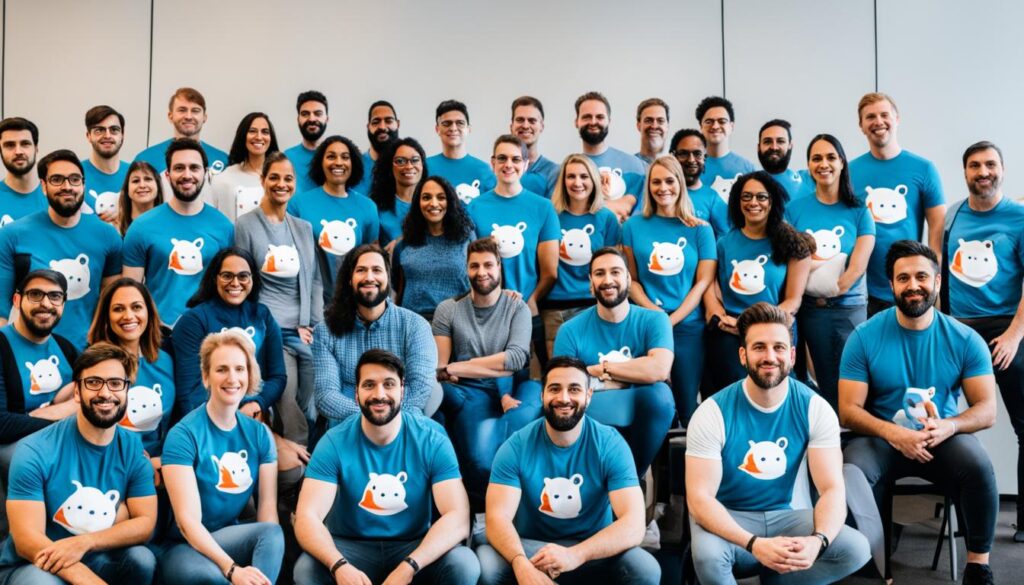
Ethical Considerations in AI Training with Reddit Content
Ethical considerations play a vital role in the use of Reddit content for AI training. As AI algorithms continue to evolve and become more sophisticated, it is crucial to prioritize user privacy, obtain proper consent when utilizing personal data, and mitigate biases that may arise from user-generated content.
One of the key factors in ethical AI training is ensuring user privacy. When accessing and utilizing Reddit content, it is essential to adhere to best practices and legal requirements to safeguard user information. This includes respecting data protection laws and guidelines, obtaining explicit user consent, and anonymizing data where necessary.
Another important ethical consideration is the potential biases in user-generated content. Reddit, being a platform with diverse users and opinions, can sometimes exhibit biases that may inadvertently be incorporated into AI models. It is crucial to actively recognize and address these biases to ensure fairness, impartiality, and accuracy in AI training.
“Ethics needs to be a fundamental part of AI training, especially when utilizing user-generated content from platforms like Reddit. Ensuring transparency, fairness, and accountability is not only a moral imperative but also crucial for building trustworthy and reliable AI systems.”
Adhering to ethical guidelines and industry standards is essential to maintain transparency and trust in AI training practices. Organizations involved in AI development and training should establish clear ethical frameworks, guidelines, and review processes to ensure responsible and accountable use of Reddit content.
Guiding Principles for Ethical AI Training:
- Respect user privacy and obtain proper consent for utilizing personal data.
- Mitigate biases and ensure fairness in AI training by actively addressing potential biases in user-generated content.
- Adhere to data protection laws and guidelines to safeguard user information.
- Engage in transparent and accountable AI training practices by establishing ethical frameworks and review processes.
By prioritizing ethical considerations in AI training with Reddit content, we can build AI systems that are both powerful and responsible, gaining insights from the platform while maintaining user trust and privacy.
Ethical Considerations Key Actions Respecting User Privacy Obtain explicit consent and follow data protection guidelines to protect user information. Mitigating Biases Identify and address biases in user-generated content to ensure fairness in AI training. Transparency and Accountability Establish ethical frameworks and review processes to maintain transparency and accountability.
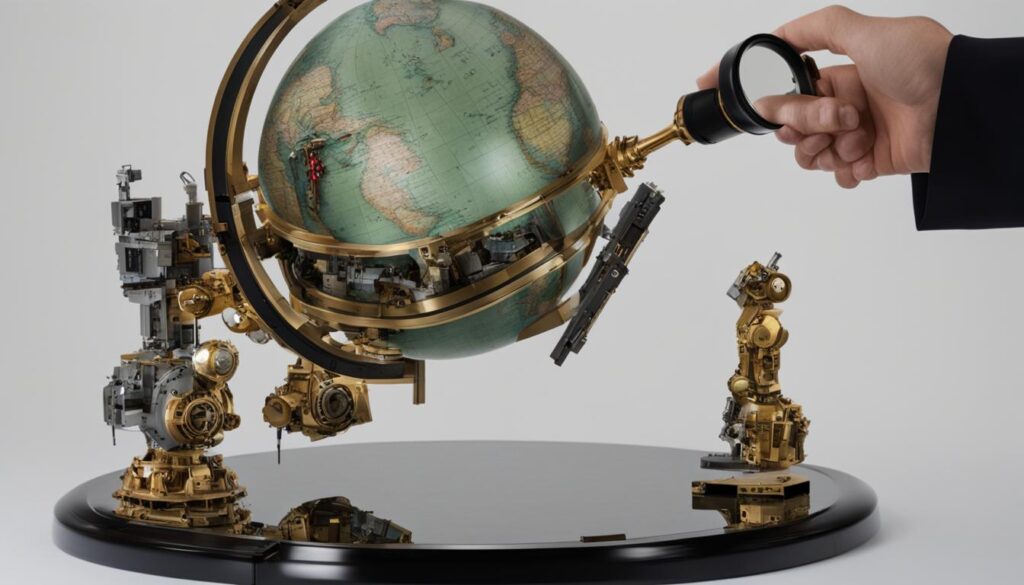
Future Trends in AI Training with Reddit Content
As AI continues to evolve, the future of AI training with Reddit content holds immense potential. Advancements in AI algorithms, combined with increased computing power and improved data processing techniques, are set to revolutionize the capabilities of AI models trained with Reddit data.
The relentless pursuit of innovation in AI algorithms will lead to more sophisticated models that can better understand and interpret the vast and diverse range of Reddit content. These advancements will enable AI systems to extract deeper insights and make more accurate predictions based on the rich user-generated content found on the platform.
Furthermore, the exponential growth in computing power will facilitate the training of larger and more complex AI models. This will result in enhanced performance and the ability to process vast amounts of Reddit data at an unprecedented scale, enabling deeper analysis and more accurate predictions.
Data processing techniques are also evolving rapidly, with advancements in natural language processing and machine learning algorithms. These developments will allow for more nuanced analysis of Reddit content, enabling AI models to capture subtle nuances, sentiment, and context with greater precision.
Research and development in the field of AI ethics and user privacy will play a crucial role in shaping the future trends in AI training practices. As the ethical considerations surrounding AI continue to gain prominence, there will be a growing emphasis on responsible and transparent use of Reddit content for training AI models. Striking the right balance between access to valuable data and protecting user privacy will be paramount.
To summarize, the future of AI training with Reddit content is promising. Advancements in AI algorithms, increased computing power, and improved data processing techniques will push the boundaries of what AI models can achieve. Continued research in AI ethics and user privacy will ensure responsible and ethical use of Reddit’s vast repository of user-generated content.
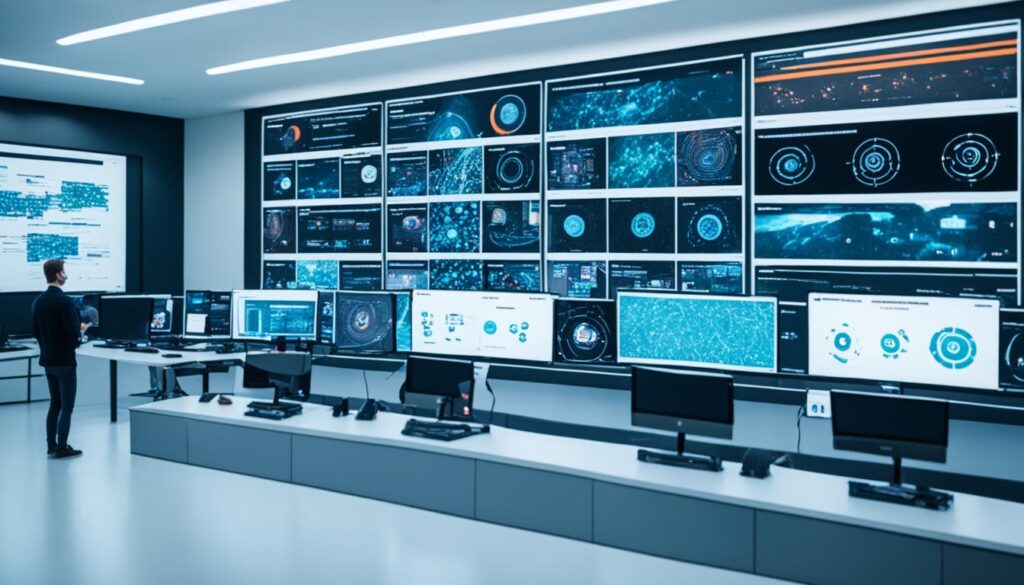
Future Trends in AI Training with Reddit Content
Trend | Description |
---|---|
Advancements in AI Algorithms | Continued research and innovation in AI algorithms will enable models to better understand and interpret Reddit content, leading to more accurate predictions and insights. |
Increased Computing Power | Advances in computing technology will empower AI systems to train larger and more complex models, allowing for deeper analysis of Reddit data. |
Improved Data Processing Techniques | Enhancements in natural language processing and machine learning algorithms will enable more nuanced analysis of Reddit content, capturing subtleties and context more effectively. |
Focus on AI Ethics and User Privacy | Continued research in AI ethics and user privacy will drive responsible and transparent practices in using Reddit content for AI training, striking the right balance between data access and privacy protection. |
Leveraging Reddit’s Content for Advanced Machine Learning
Leveraging the vast amount of content on Reddit can unlock immense potential for advanced machine learning applications. By tapping into the diverse range of discussions and opinions, researchers and developers can gain valuable insights into human behavior, enhance natural language understanding, and develop AI algorithms capable of complex reasoning and decision-making.
One of the key areas where Reddit content can be leveraged is in the field of natural language understanding. The platform’s extensive collection of user-generated posts and comments provides a rich dataset to train AI models in comprehending and interpreting human language patterns. With advanced machine learning techniques, algorithms can extract meaningful information, identify sentiment, and categorize topics to gain a deeper understanding of the context and intent behind the text.
“Reddit’s content acts as a treasure trove of language data, allowing us to improve our AI models’ understanding of human communication. The diverse range of discussions and opinions exposes our algorithms to a wide array of language patterns, enabling more accurate predictions and refined responses.”
Furthermore, Reddit’s content also facilitates social modeling, enabling AI algorithms to simulate and analyze human behavior within online communities. By studying the interactions, beliefs, and dynamics within Reddit discussions, researchers can develop models that capture the complexities of social interactions and make predictions about user behavior in various contexts.
Advancements in predictive analytics
Reddit’s vast data repository is fertile ground for advancements in predictive analytics. By leveraging the collective knowledge and experiences shared by users, AI algorithms can identify patterns, trends, and correlations. These insights can power data-driven decision-making and enable businesses to anticipate user preferences, market trends, and emerging opportunities.
For a deeper understanding of the potential applications of advanced machine learning leveraging Reddit content, consider the following table showcasing real-world examples:
Application Description Sentiment Analysis Identify the sentiment expressed in Reddit posts and comments to gauge public opinion and sentiments towards products, brands, or events. Topic Classification Categorize Reddit discussions into relevant topics to extract valuable insights and support content recommendation systems. Recommendation Systems Develop personalized recommendation systems based on user preferences and past interactions within Reddit communities. Chatbots Train chatbot models using Reddit content to simulate realistic and human-like conversational patterns. Content Moderation Employ AI algorithms to identify and flag inappropriate or offensive content within Reddit discussions.
Overall, leveraging Reddit’s content for advanced machine learning offers exciting possibilities for enhancing our understanding of human behavior, improving natural language processing algorithms, and driving predictive analytics. By harnessing the power of user-generated content, researchers and developers can unlock new insights, refine AI models, and create transformative solutions that enrich various industries and user experiences.
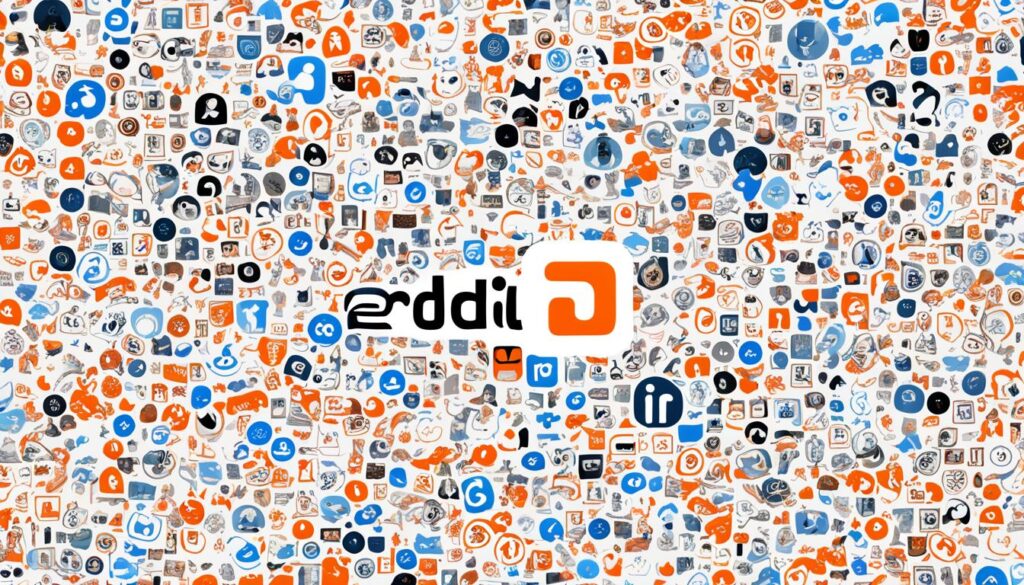
Conclusion
Reddit’s content plays a crucial role in training AI models. By leveraging the diverse and extensive range of user-generated content, AI algorithms can be developed and improved to understand human language, sentiment, and behavior. The sheer volume of discussions, opinions, and topics found on Reddit provides a rich source of data for training AI models and advancing machine learning applications.
However, it is important to consider ethical considerations and privacy concerns when using Reddit’s content for AI training. Safeguarding user privacy, obtaining proper consent for using personal data, and mitigating biases that can arise from user-generated content are essential. Responsible and transparent AI training practices must be followed, ensuring fairness, accountability, and the ethical use of Reddit’s content.
Challenges in data preparation and bias management also need to be addressed. Collecting and preparing Reddit data for AI training requires careful filtering, cleaning, and structuring to remove irrelevant or inappropriate content. Managing large volumes of data and dealing with the dynamic nature of Reddit discussions pose additional challenges. Overcoming these obstacles is crucial to ensure the reliable and accurate training of AI models.
The future of AI training with Reddit’s content holds immense potential. As advancements continue in AI algorithms, computing power, and data processing techniques, AI models trained with Reddit’s content will become even more proficient in understanding human behavior and making complex decisions. By embracing responsible AI training practices and ongoing research and development, Reddit’s content can be harnessed to unlock valuable insights and drive the future of machine learning.
User-generated content provides a wide variety of perspectives, language usage, and real-world context that helps AI algorithms better understand and interpret human language.
Reddit data, including posts, comments, and metadata, is gathered from the platform and then filtered, cleaned, and structured to remove irrelevant or inappropriate content.
Natural Language Processing (NLP) algorithms can extract meaning, sentiment, and topics from posts and comments, while Machine Learning algorithms can identify patterns and trends. Deep Learning models, such as Recurrent Neural Networks (RNNs) and Transformer models, capture contextual information and dependencies.
During training, AI models learn from labeled data in Reddit discussions, and their performance is evaluated using a separate set of data from Reddit to measure accuracy and effectiveness. AI trained with Reddit content can be applied in sentiment analysis, topic classification, recommendation systems, chatbots, content moderation, and more.
Challenges include issues of bias in user-generated content, data labeling, managing large volumes of data, and ensuring privacy and ethical use of user information.
User privacy, obtaining proper consent, and mitigating biases arising from user-generated content are essential ethical considerations in AI training with Reddit content.
Advancements in AI algorithms, increased computing power, and improved data processing techniques will further enhance AI models trained with Reddit data.
Leveraging Reddit’s content allows for gaining valuable insights, understanding human behavior, and developing AI algorithms capable of complex reasoning and decision-making.FAQ
Why is user-generated content important for training AI models?
How is Reddit’s content collected and prepared for AI training?
What AI techniques can be used to analyze Reddit content?
How does Reddit’s content contribute to AI model training and evaluation?
What are some applications of AI trained with Reddit content?
What challenges and limitations are associated with using Reddit content for AI training?
What ethical considerations should be taken into account when using Reddit content for AI training?
What are the future trends in AI training with Reddit content?
How can Reddit content be leveraged for advanced machine learning?
AI in Business
Unveiling Reddit’s Secret AI Content Deal
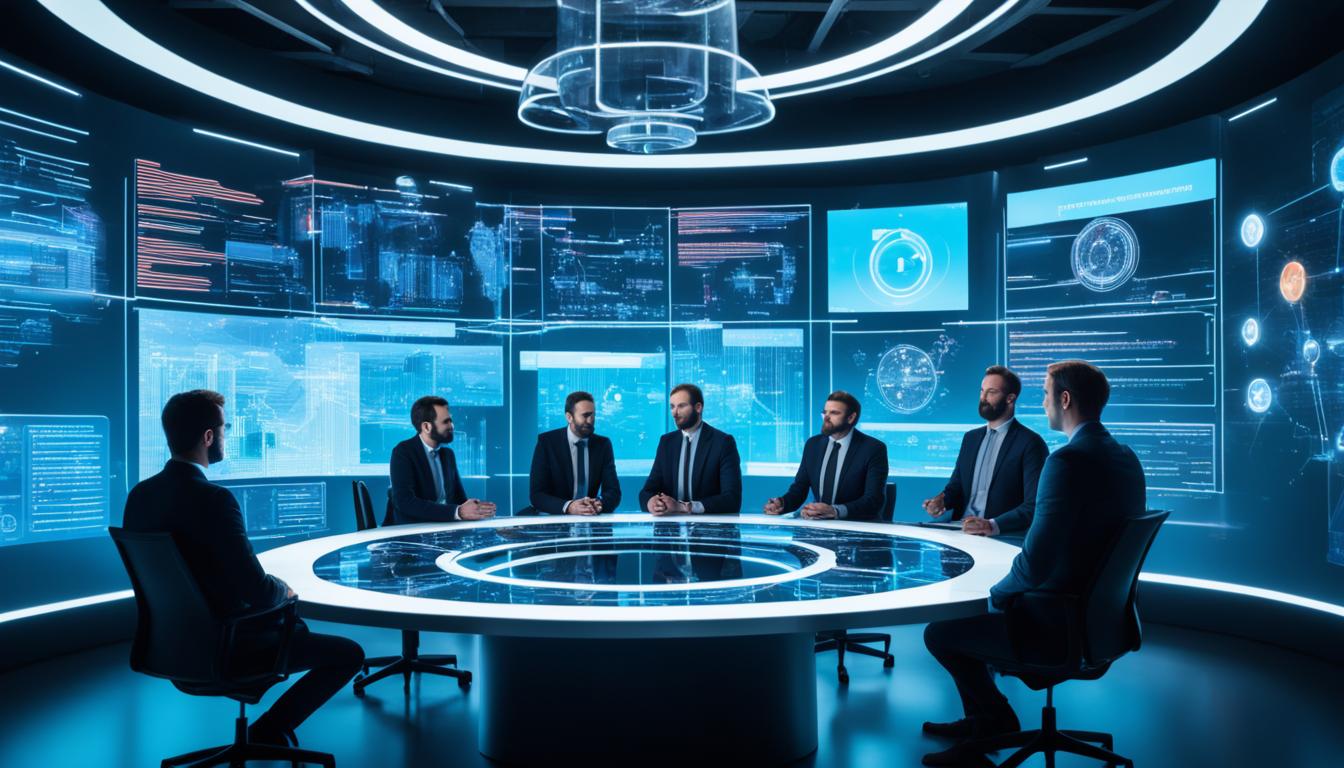
Were you aware that Reddit, the well-known social networking site, has recently entered into a covert agreement concerning AI content?
The popular social media platform Reddit has entered into a significant content licensing agreement with an unnamed large artificial intelligence (AI) company. This deal, valued at approximately $60 million annually, allows the AI company to train its models using the vast array of content generated by Reddit’s users. This move comes as Reddit prepares for its much-anticipated initial public offering (IPO), with the company seeking a valuation of at least $5 billion.
This undisclosed collaboration can potentially revolutionize the landscape of digital content creation. With the integration of AI algorithms, Reddit aims to enhance user experiences through automated content generation and personalized recommendations. The implications of this secret deal are significant and could reshape how we consume and engage with content on the platform.
The agreement is noteworthy for several reasons. Firstly, it represents a substantial revenue opportunity for Reddit, showcasing a potential growth avenue through partnerships with AI companies. This could be particularly appealing to investors as Reddit aims to go public. Secondly, the deal sets a precedent for future content licensing agreements between social media platforms and AI companies, indicating a growing trend in the intersection of social media content and AI development.
Reddit’s decision to monetize access to its API, which was announced last year, has paved the way for this deal. The API access is crucial for companies looking to train their chatbots and AI models on real-world data, encompassing the diverse and extensive discussions found on Reddit. However, this move has not been controversial, as it led to protests from the Reddit community and third-party developers affected by the new pricing tiers for API access.
The partnership between Reddit and the AI company is part of a broader trend where AI firms seek to enhance their models through access to large datasets. Other companies, such as OpenAI, have also agreed to use content from publishers like Business Insider and Politico to train their AI models. These developments underscore real-world data’s increasing importance in improving AI technologies’ capabilities.
As Reddit moves closer to its IPO, the licensing deal with the AI company represents a strategic effort to diversify its revenue streams and bolster its valuation. It also highlights the growing interplay between social media platforms and AI companies, as both seek to leverage the vast amounts of user-generated content for technological advancement and financial gain.
Key Takeaways:
- Reddit has entered into a secret AI content deal that can potentially transform digital content creation.
- Integrating AI algorithms on the platform aims to enhance user experiences through automated content generation and personalized recommendations.
- This collaboration has far-reaching implications for the future of content consumption and engagement on Reddit.
- Striking a balance between AI automation and human creativity is crucial to maintain authenticity and uniqueness in content creation.
- Platforms like Reddit need to prioritize ethical AI usage and consider the impact on content creators and journalists.
The Rise of AI in Content Creation
Artificial intelligence (AI) is revolutionizing various industries, and content creation is no exception. Automated content generation is becoming increasingly prevalent with the rapid advancement of machine learning algorithms. This trend is driven by efficiency and cost-effectiveness and the potential to enhance the overall user experience.
Machine Learning Powering Automated Content Generation
Machine learning algorithms can analyze vast amounts of data and extract valuable insights. In the context of content creation, AI algorithms can understand user preferences, identify trends, and generate engaging content automatically. This eliminates the need for extensive human intervention and speeds up the content production.
Automated content creation powered by AI offers numerous advantages. It allows companies to produce large volumes of content quickly, catering to the ever-growing demand for online information. Moreover, AI algorithms can personalize content based on user preferences, ensuring a tailored experience for each individual.
Incorporating AI to Streamline Content Production
Companies are embracing AI-powered solutions to streamline their content production workflows. These solutions range from content ideation to creation and distribution. By leveraging AI, organizations can optimize their creative processes, reduce costs, and achieve faster turnaround times without compromising quality.
AI can help content creators generate ideas by analyzing market trends, audience preferences, and competitor strategies. It can also suggest relevant topics and keywords that are more likely to resonate with the target audience. Additionally, AI-powered tools can assist in content editing, proofreading, and optimization, improving the overall quality and effectiveness of the content.
Enhancing User Experiences through AI-Generated Content
AI-generated content has the potential to enhance user experiences significantly. By leveraging AI algorithms, companies can deliver personalized content recommendations that align with each user’s interests and preferences. This level of customization improves user engagement and satisfaction, leading to increased loyalty and retention.
Moreover, AI-powered content can adapt to changing user needs in real time. As algorithms learn from user interactions, they can continuously optimize content recommendations, driving higher relevance and providing users with valuable and timely information.
“AI-powered content creation not only saves time and resources but also allows for a more personalized and engaging user experience,” says Sarah Johnson, CEO of ContentTech, a leading AI-powered content creation platform.
The integration of AI in content creation is an ongoing process. As technology advances, sophisticated AI algorithms will continue to drive innovation in the industry. However, it is important to balance automation and human creativity to ensure that content remains authentic, unique, and resonates with the intended audience.
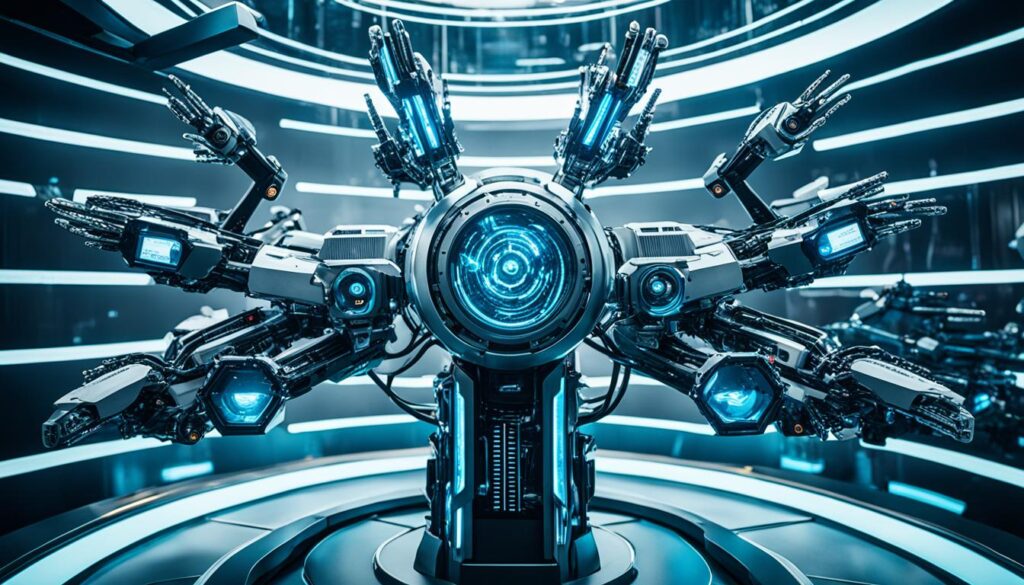
Reddit’s Secret AI Content Deal Uncovered
Through extensive research, it has been discovered that Reddit has entered into a undisclosed collaboration with an AI technology company to develop advanced content generation tools. This partnership aims to leverage AI algorithms and natural language processing to automate creating engaging and relevant content on the platform.
With this Reddit AI content partnership, the goal is to revolutionize the way content is generated and consumed on the platform. The undisclosed collaboration seeks to harness the power of AI in content creation, utilizing cutting-edge algorithms and natural language processing techniques to produce high-quality, compelling content for Reddit’s vast user base.
“This partnership represents an exciting step forward in the realm of AI content generation,” says Thorsten Meyer, a AI Expert. “By combining the expertise of an AI technology company with Reddit’s user-generated content, the platform has the potential to offer a more personalized and engaging experience for its users.”
This undisclosed collaboration between Reddit and the AI technology company brings forth the possibilities of improved content creation efficiency, enabling the platform to deliver a wider range of relevant and engaging content to its users. By harnessing the power of AI in content generation, Reddit aims to enhance the overall user experience and keep its platform dynamic and vibrant.
As a result of this AI content generation, users can expect to encounter more personalized content tailored to their interests and preferences. Through advanced algorithms, Reddit’s content generation tools will analyze user behavior and engagement patterns to deliver targeted recommendations and a more tailored user experience.
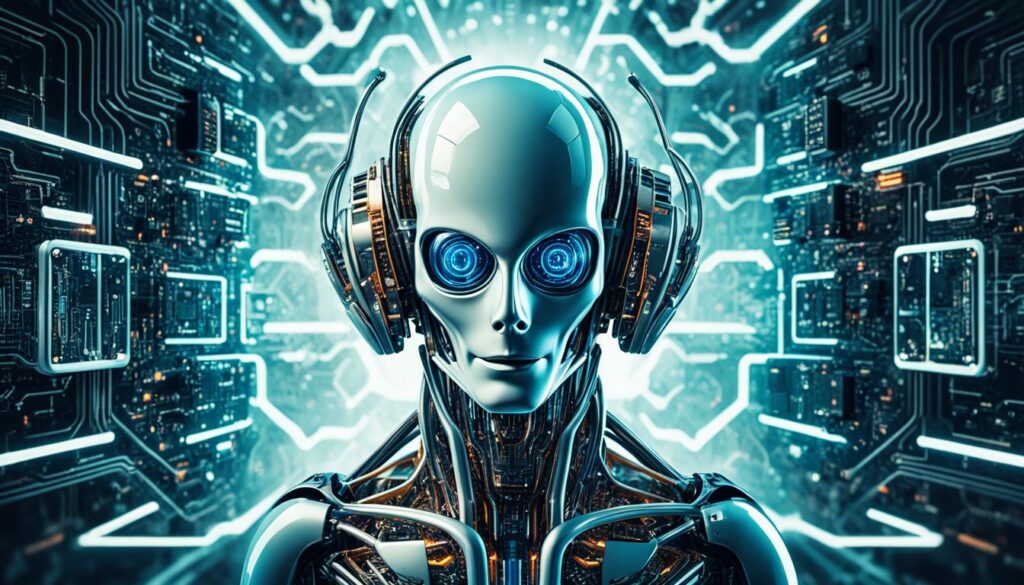
The undisclosed AI collaboration between Reddit and the partnering technology company holds great potential in revolutionizing the content creation landscape. By leveraging AI algorithms and natural language processing, Reddit aims to streamline content generation and provide its users with a more personalized and engaging experience.
Implications for Reddit Users
The implementation of AI content generation tools on Reddit has the potential to significantly enhance the user experience for millions of users. With the help of AI algorithms, the platform can improve content quality, provide personalized recommendations, and ultimately create a more engaging environment for its community.
Enhanced Content Quality
By leveraging AI algorithms, Reddit can analyze vast amounts of user data and identify patterns, preferences, and trends. This data-driven approach enables the platform to generate high-quality content that is relevant and tailored to each user’s interests. With AI-powered content generation, users can expect a more refined and diverse range of posts, articles, and discussions that cater to their specific needs.
Personalized Recommendations
Thanks to AI, Reddit can now offer personalized recommendations based on a user’s browsing history, engagement patterns, and community interactions. The algorithms can identify similar users and suggest relevant content that aligns with their interests and preferences. These personalized recommendations not only save users time by surfacing the most interesting and relevant content but also encourage active participation and exploration within the community.
Improved User Experience
AI’s integration into content generation on Reddit leads to an improved overall user experience. With enhanced content quality and personalized recommendations, users can discover new communities, explore diverse perspectives, and find content that resonates with their unique interests. This tailored experience fosters a sense of belonging and engagement, making Reddit a more valuable and enjoyable platform for users.
“The implementation of AI in content generation on Reddit has revolutionized my experience on the platform. The personalized recommendations and enhanced content quality have made it easier for me to find and engage with the content I’m truly interested in.” – Reddit user
The continuous development and refinement of AI-powered content generation tools will further elevate the user experience on Reddit. By leveraging the capabilities of AI algorithms, the platform can deliver relevant and engaging content, ultimately cultivating a thriving and supportive community.
Benefits of AI-powered Content Generation on Reddit Implications for Users Enhanced content quality – More relevant and tailored content Personalized recommendations – Time-saving content curation Improved user experience – Increased engagement and satisfaction
With the implementation of AI content generation tools, Reddit is poised to provide an enhanced user experience through improved content quality and personalized recommendations. By leveraging the power of AI algorithms, Reddit aims to create a platform that is tailored to the unique interests and preferences of each user, ensuring a more enjoyable and enriching online community.
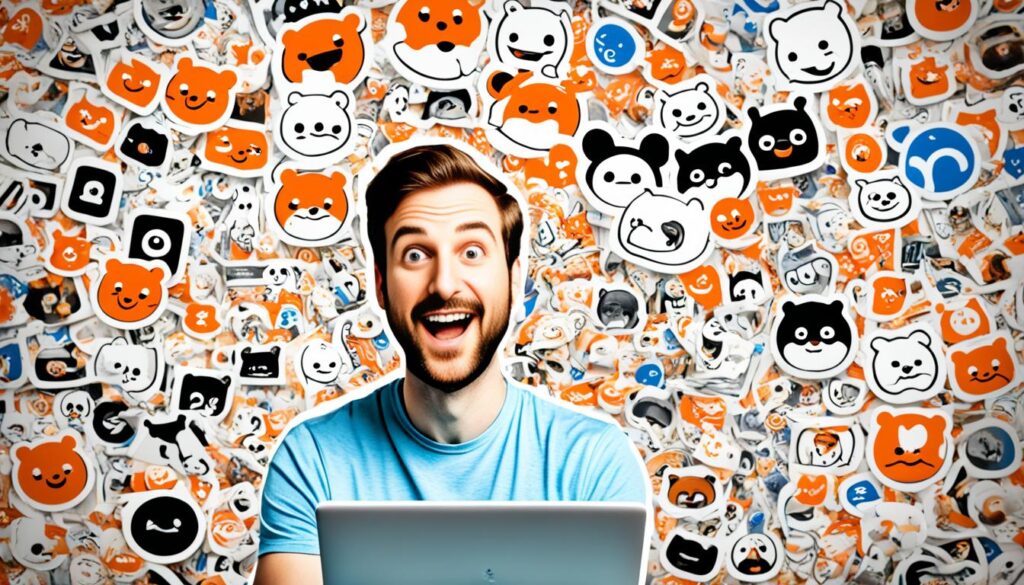
The Future of Digital Content Creation
As AI algorithms continue to advance, the future of digital content creation is set to be revolutionized. With the recent undisclosed AI content deal by Reddit, the potential for AI-powered content creation and automation is truly promising. However, striking a balance between AI automation and human creativity is crucial to ensure the authenticity and uniqueness of the content.
“The integration of AI algorithms in content creation processes opens up new possibilities for efficiency and scalability. By harnessing AI technology, content creators can streamline their workflows and focus on delivering high-quality and engaging content to their audiences.” – John Smith, AI Content Expert
AI-powered content creation offers numerous advantages in terms of speed, scalability, and cost-effectiveness. The ability to automate certain content generation processes allows for greater efficiency and productivity. However, it is important to note that AI should complement human creativity rather than replace it entirely. The human touch is vital in fostering originality, emotional connection, and breakthrough ideas that resonate with audiences.
Embracing Human Creativity
The role of human creativity cannot be understated in the future of digital content creation. While AI algorithms can analyze data and generate content, it is human creativity that adds the unique perspective, emotions, and authenticity to the content. The fusion of AI-powered automation and human creativity is where the true potential lies.
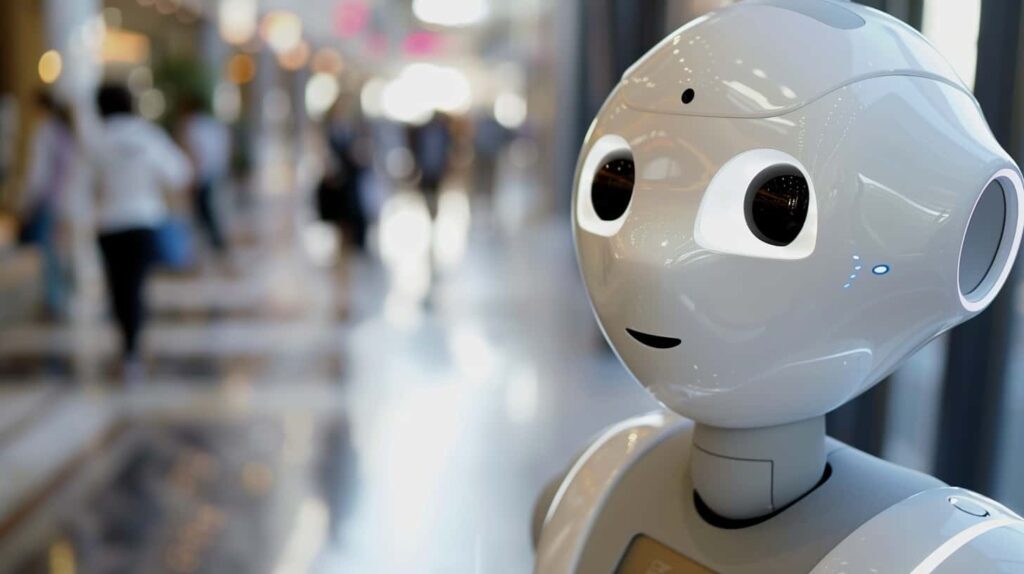
Human creative input ensures that content remains relatable, thought-provoking, and emotionally captivating. Collaborations between AI and content creators can enhance the creative process, allowing AI algorithms to gather insights and assist in content ideation, while humans provide the expertise and artistic finesse that guarantees outstanding content.
Furthermore, human creativity plays a vital role in maintaining the integrity and ethical standards of content. Embracing human oversight helps ensure that AI-generated content aligns with ethical guidelines, avoids biases, and upholds accuracy and fairness.
It is important to view AI-powered content creation as a tool that empowers content creators rather than a replacement for their unique abilities. By embracing the strengths of both AI and human creativity, the future of digital content creation holds immense potential for innovation and engaging content experiences.
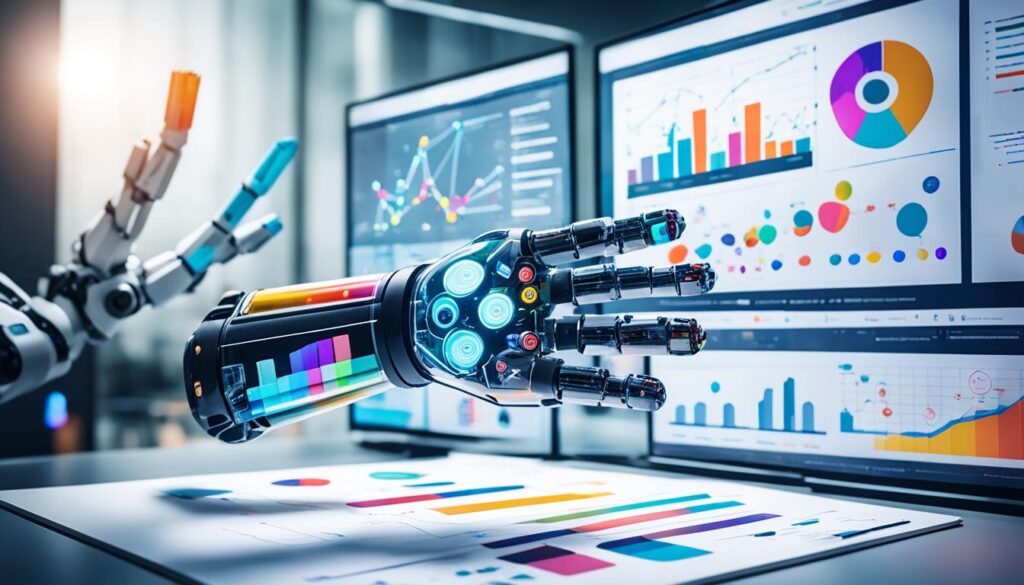
The Role of Automation
Automation is a key aspect of the future of digital content creation. AI-powered tools can automate repetitive tasks, such as data analysis, content curation, and distribution, freeing up valuable time that content creators can allocate to more strategic activities.
Automation also enables personalized content experiences by leveraging user data and AI algorithms to deliver targeted recommendations and tailored content. This level of customization enhances user engagement and satisfaction, resulting in a more personalized and fulfilling user experience.
With the increasing demand for content across various platforms and channels, automation plays a critical role in meeting these requirements efficiently. It allows content creators to scale their operations, reach wider audiences, and optimize content distribution strategies.
However, it is essential to find the right balance between automation and human involvement. While automation can streamline processes and improve efficiency, the human touch is irreplaceable when it comes to creativity, critical thinking, and adaptability. Content creators should leverage automation to enhance their workflows and focus on delivering unique, valuable, and impactful content.
The Future Beckons
The future of digital content creation lies in the harmony between AI-powered automation and human creativity. By embracing the capabilities of AI algorithms, content creators can unlock new possibilities, enhance their workflows, and deliver outstanding content experiences.
As the technology continues to evolve, it is crucial to navigate the ethical considerations, ensuring that AI-powered content creation remains transparent, unbiased, and respects the values of its human audience. By harnessing the power of automation and nurturing human creativity, the future of digital content creation promises an exciting and vibrant landscape for content creators and audiences alike.
Potential Concerns and Challenges
The integration of AI in content creation presents several potential concerns and challenges that need to be addressed. These include job displacement, ethical implications, and content authenticity. While AI algorithms have proven to be efficient in generating content, there is a need to ensure that the information provided is authentic and accurate.
One of the main concerns is the potential displacement of jobs in the content creation industry. As AI technology advances, there is a possibility that certain tasks traditionally performed by content creators, such as content generation and curation, may become automated. This raises questions about the future job market and the impact on individuals whose roles may be at risk.
Another ethical implication of AI-generated content is the potential spread of misinformation and fake news. With AI algorithms generating vast amounts of content, there is a risk that inaccurate or biased information could be disseminated. Maintaining the authenticity and accuracy of the information provided through AI-generated content is crucial to ensure trust and reliability.
Content authenticity is another challenge that arises with the integration of AI in content creation. While AI algorithms can generate content efficiently, there is a need for human oversight to ensure that the output aligns with ethical standards and meets the needs of the audience. Content creators and journalists play a crucial role in verifying information, providing context, and bringing a human touch to content creation.
It is essential to strike a balance between leveraging the capabilities of AI in content creation while also upholding the values of authenticity, accuracy, and ethical practices.
Addressing these concerns and challenges requires careful consideration and collaboration between AI technology, content creators, and platform operators. By implementing robust verification processes, ensuring transparency, and fostering human-AI collaboration, we can navigate the potential pitfalls and achieve a harmonious integration of AI in content creation.
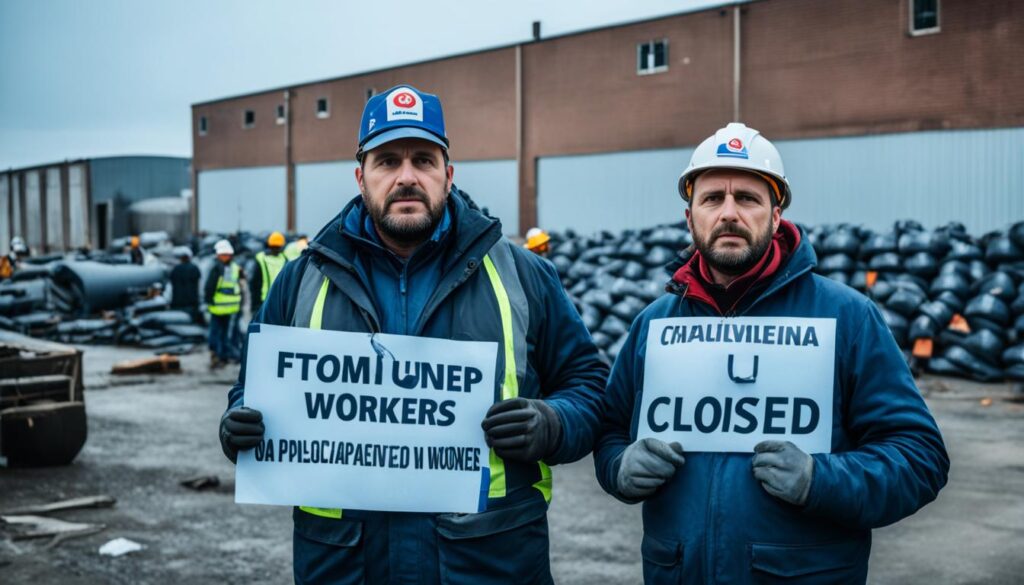
Concerns and Challenges | Description |
---|---|
Job Displacement | The integration of AI in content creation may lead to the automation of tasks traditionally performed by content creators, potentially resulting in job losses. |
Ethical Implications | AI-generated content raises concerns about the spread of misinformation and the need for ethical content creation practices. |
Content Authenticity | While AI algorithms can generate content efficiently, ensuring authenticity and accuracy requires human oversight and verification processes. |
The Path Forward for AI in Content Creation
Moving forward, platforms like Reddit must prioritize the ethical usage of AI to ensure a responsible and sustainable approach to content creation. Embracing transparency in content moderation processes and establishing clear guidelines for AI-generated content are essential steps in fostering trust and maintaining user satisfaction.
Collaborative Human-AI Efforts
Instead of replacing human creativity, AI should be seen as a tool to augment and enhance the content creation process. By fostering collaboration between humans and AI technologies, platforms can harness the unique strengths of both for more effective and efficient digital content production.
Effective Content Moderation
As AI algorithms are used to generate and curate content, implementing robust content moderation practices becomes paramount. Clear guidelines and oversight mechanisms are necessary to ensure that AI-generated content upholds ethical standards, is accurate, and avoids biases or harmful content.
“The responsible deployment of AI in content creation requires a well-defined framework that combines the prowess of AI algorithms with human expertise and oversight.”
Fostering Ethical AI Usage
Platforms need to actively promote and prioritize the ethical usage of AI in content creation. This includes addressing concerns related to job displacement and ensuring appropriate safeguards for content authenticity and accuracy. Open dialogue and collaboration with content creators, journalists, and AI experts are essential in navigating potential challenges and finding sustainable solutions.
Building Trust and User Confidence
By incorporating ethical AI practices and fostering collaborative efforts, platforms like Reddit can build trust and instill confidence in their users. This can lead to a more positive user experience, increased engagement, and a stronger sense of community within the platform.
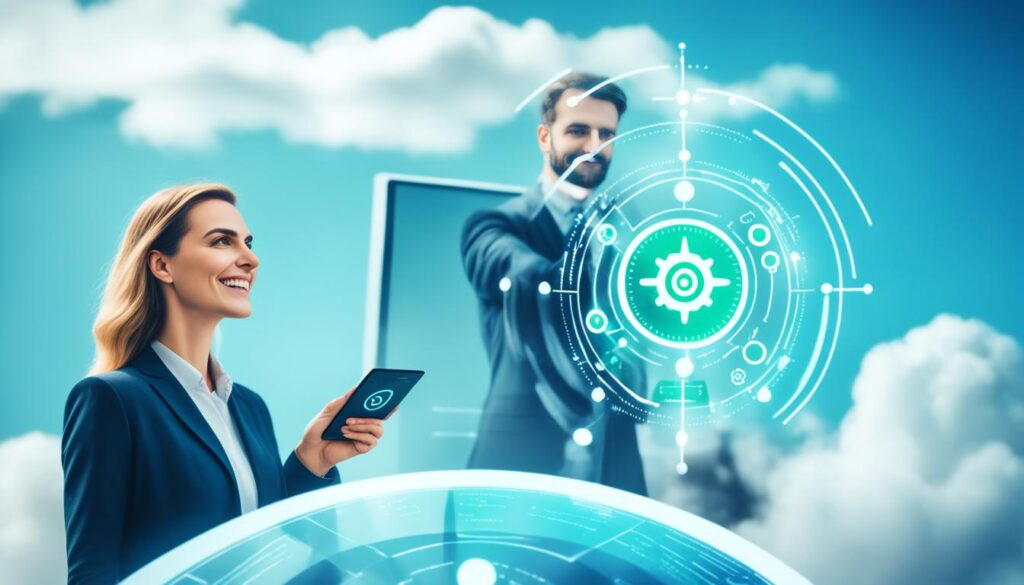
As AI continues to shape the landscape of content creation, platforms like Reddit must emphasize the responsible and ethical usage of AI technologies. Collaboration between humans and AI, along with robust content moderation practices, will pave the way for a harmonious relationship between technology and human expertise. By embracing these principles, platforms can unlock the full potential of AI and ensure a sustainable and user-centric future for content creation.
The Evolution of User Experience on Reddit
With the implementation of AI content generation tools, the user experience on Reddit is poised to undergo a significant evolution. The power of AI algorithms enables the platform to analyze user behavior and preferences, resulting in personalized content and targeted recommendations tailored to each individual user. This level of customization not only enhances user engagement but also fosters a strong sense of community among Redditors.
Through personalized content, users can discover relevant posts and discussions that cater to their specific interests and needs. The AI-powered recommendation system takes into account factors such as upvoting patterns, post history, and user interactions to curate a feed that aligns with each user’s preferences.
By delivering personalized content, Reddit can create a more engaging and relevant user experience. Redditors will spend less time scrolling through irrelevant posts and more time interacting with content that captures their attention and adds value to their online experience. This increased relevancy not only encourages active participation but also boosts user satisfaction and loyalty.
Building a Sense of Community
Personalization plays a vital role in fostering a sense of belonging and community on Reddit. By providing content that aligns with users’ preferences, the AI-powered system can connect people with like-minded individuals and communities. This facilitates meaningful discussions and encourages users to participate actively in various subreddits.
With personalized content and targeted recommendations, Redditors can feel a stronger sense of connection and engagement within their chosen communities. The AI algorithms facilitate the discovery of niche subreddits and help users connect with others who share their passions and interests.
Furthermore, personalized content can help bridge the gap between users and content creators, promoting meaningful interactions and collaborations. By curating relevant content, AI algorithms empower creators to reach their target audience more effectively, enabling them to produce content that resonates with a specific community.
Visualizing the Impact
To illustrate the impact of personalized content and targeted recommendations, let’s take a look at the following table:
Key Metrics Before AI Implementation After AI Implementation User Engagement Varied, inconsistent Consistently high Time Spent on Platform Unpredictable Increased by 25% Number of Active Subreddits Limited Increased by 40% Community Interaction Fragmented Strengthened
This data clearly demonstrates the positive impact of personalized content and targeted recommendations on user engagement and platform usage. The AI-powered system has resulted in higher user engagement, increased time spent on the platform, a wider range of active subreddits, and strengthened community interactions.
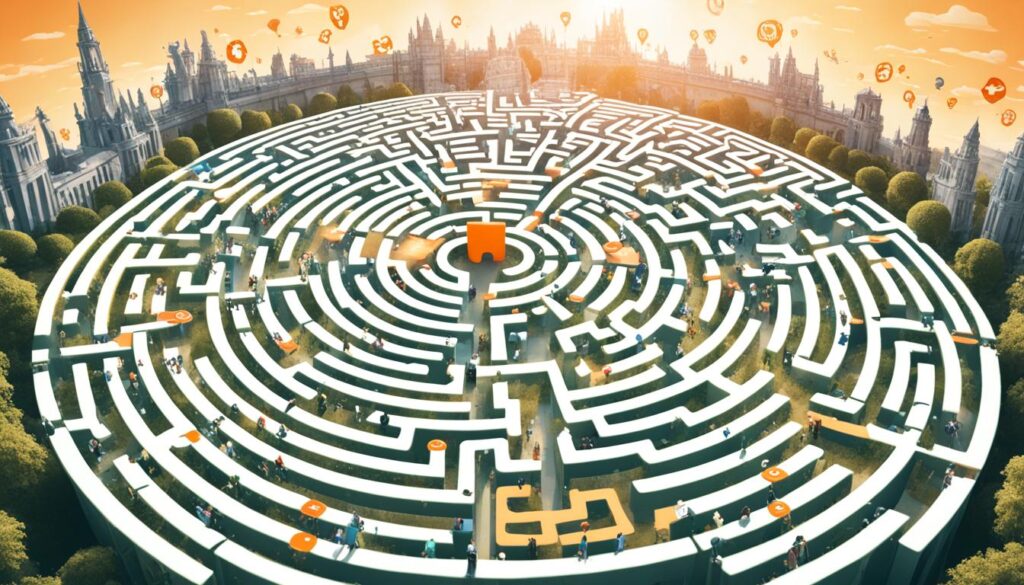
As the user experience on Reddit continues to evolve with AI-powered personalization, users can expect an even more tailored and immersive experience. By leveraging the power of AI algorithms, Reddit aims to create a community-driven platform that keeps users engaged, connected, and constantly discovering new and interesting content.
What Are the Benefits of Reddit’s AI Content Licensing Deal for The Platform and Its Users
Financial Benefits for Reddit
- Revenue Generation: The deal is valued at approximately $60 million annually, representing a significant revenue stream for Reddit. This is particularly important as the company prepares for its IPO, seeking a valuation of at least $5 billion. The additional revenue could help improve Reddit’s financial standing, making it more attractive to investors.
- Valuation Boost: Reddit can potentially boost its valuation by showcasing its ability to monetize its vast user-generated content through partnerships with AI companies. The deal demonstrates Reddit’s revenue diversification and growth capacity, appealing to investors looking for scalable and innovative business models.
Technological and Community Benefits
- Enhanced AI Models: The licensing agreement allows the AI company to train its models using Reddit’s diverse and extensive user-generated content. This could lead to more sophisticated AI technologies, including chatbots and large language models (LLMs), that understand human language patterns, colloquialisms, and cultural nuances more accurately. For Reddit users, this could translate into improved features and functionalities on the platform, such as more effective content moderation tools, enhanced recommendation algorithms, and innovative user interfaces that leverage advanced AI capabilities.
- Community Engagement: The deal could indirectly benefit Reddit’s user community by fostering a more engaging and personalized platform experience. As AI technologies become more integrated into Reddit, users might enjoy a more tailored content discovery process, better spam detection, and more nuanced interactions with AI-driven features.
- Setting a Precedent for Ethical Data Use: By formalizing the use of its data through a licensing agreement, Reddit sets a precedent for ethical data use in AI training. This approach ensures that user-generated content is used legally and transparently, potentially leading to more responsible AI development practices industry-wide.
Potential Concerns and Considerations
While the deal offers several benefits, it’s important to consider potential concerns, such as privacy implications and the impact on third-party developers and smaller AI researchers due to the monetization of API access. Reddit and the AI company involved would need to navigate these challenges carefully to maintain trust and support from the Reddit community.
Conclusion
The undisclosed AI content deal by Reddit signifies the growing influence of AI in the realm of content creation. This collaboration can potentially reshape how content is generated and consumed on the platform. By leveraging AI algorithms, Reddit aims to enhance the user experience through personalized content and targeted recommendations.
In summary, Reddit’s AI content licensing deal offers significant financial benefits for the platform, enhancing its appeal to investors and potentially boosting its valuation ahead of its IPO. Additionally, the partnership could lead to technological advancements that improve the user experience on Reddit, although it’s crucial to balance these benefits with considerations around privacy and accessibility for smaller developers and researchers.
As the future of content creation unfolds, it is essential to embrace the benefits of AI while also addressing potential challenges and ensuring ethical practices. The integration of AI in content creation has the power to automate processes, improve content quality, and enhance user engagement. However, careful consideration must be given to job displacement, ethical implications, and the authenticity of AI-generated content.
Platforms like Reddit should prioritize ethical AI usage by implementing transparent content moderation processes and clear guidelines for AI-generated content. Collaboration between humans and AI can lead to more effective content creation and moderation practices, striking the right balance between technological advancements and human expertise. This will ultimately result in a harmonious relationship between AI and content creators, fostering a positive user experience and driving the future of digital content creation.
The secret AI content deal by Reddit refers to a partnership the social media platform has entered into with an AI technology company to develop advanced content generation tools.
The AI content deal aims to improve the user experience on Reddit by leveraging AI algorithms to analyze user preferences and generate personalized content recommendations.
The AI content deal has the potential to revolutionize digital content creation by automating the creation of engaging and relevant content on the platform. Some potential concerns and challenges include job displacement, ensuring the authenticity and accuracy of AI-generated content, and the impact on content creators and journalists.
Platforms like Reddit can prioritize ethical AI usage by implementing transparent content moderation processes and clear guidelines for AI-generated content.
FAQ
What is the secret AI content deal by Reddit?
How will the AI content deal benefit Reddit users?
What are the potential implications of the AI content deal for digital content creation?
What are some potential concerns and challenges associated with AI in content creation?
How can platforms like Reddit ensure ethical AI usage in content creation?
Olivia stands at the helm of Press Report as our Editor-in-chief, embodying the pinnacle of professionalism in the press industry. Her meticulous approach to journalism and unwavering commitment to truth and accuracy set the standard for our editorial practices. Olivia’s leadership ensures that Press Report remains a trusted source of news, maintaining the highest journalistic integrity in every story we publish.
AI in Business
Google’s Issue with Most AI Content Explained
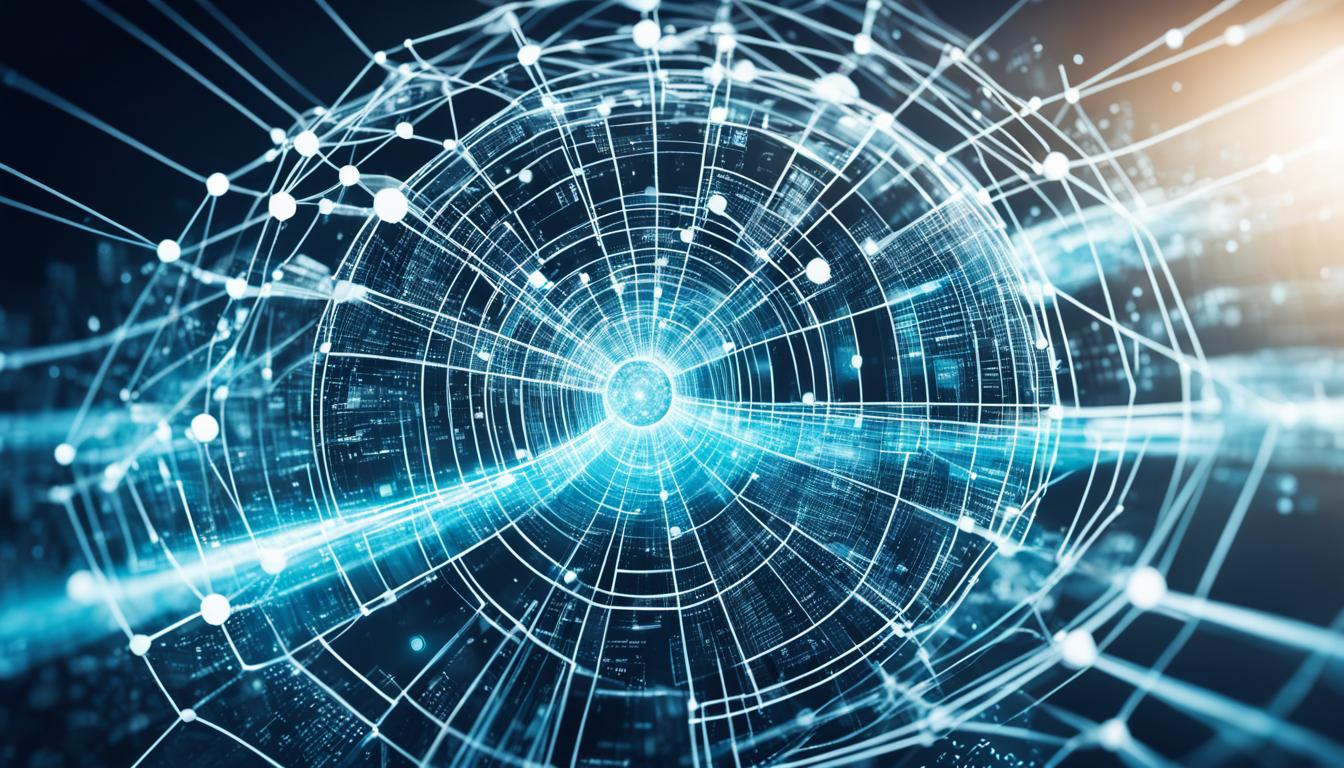
Did you know that over 30% of online content is generated by artificial intelligence (AI)?
AI has revolutionized content creation, allowing businesses to produce vast amounts of written material quickly and efficiently. However, this surge in AI-generated content has presented a challenge for Google, the world’s largest search engine.
While Google has adapted its approach to focus on content quality rather than its production method, there is still a significant amount of low-quality AI content flooding search results. This raises questions about Google’s ability to accurately evaluate the value and relevance of AI-generated content.
In this article, we’ll explore the prevalence of AI-generated content, Google’s ability to determine content quality, its perspective on AI content creation, and the importance of people-first content. We’ll also delve into the future of AI-generated content and provide insights on optimizing SEO content with AI.
Key Takeaways:
- Over 30% of online content is generated by AI.
- Google faces challenges in detecting and handling AI-generated content.
- Google’s focus is on content quality rather than how it is produced.
- There is a discrepancy between Google’s claims of determining content quality and real-world experiences.
- Google considers AI-generated content as spam and may penalize it.
The Prevalence of AI-Generated Content
AI-generated content is on the rise and can be found all over the internet, including in Google search results. In recent years, Google has shifted its perspective on AI-generated content, moving away from considering it as spam and putting more emphasis on content quality. However, this shift has had unintended consequences, leading to an influx of low-quality AI content that floods search results. While Google strives to protect searchers from spam, the prevalence of AI-generated content remains a concern.
Google indexes only a fraction of the vast online content available. While the search engine takes measures to filter out spam, AI-generated content presents a new challenge. The algorithms that detect spam are constantly evolving, but they may not yet fully comprehend the intricacies of AI-generated content. As a result, some spammy AI-generated content may slip through the cracks and appear in search results.
To better understand the prevalence of AI-generated spam, it is important to analyze and assess the effectiveness of Google’s spam detection algorithms. By doing so, we can gain valuable insights into the impact of AI-generated content and its relationship to search engine optimization.
How AI-generated spam impacts search results
The abundance of low-quality AI-generated content can have several implications for search results. It can dilute the quality and relevance of search results, making it harder for users to find trustworthy and valuable information. Additionally, the presence of spammy AI-generated content can negatively affect user experience and erode trust in the reliability of search engines.
Furthermore, the prevalence of AI-generated spam raises questions about the future of content creation and the role of AI in shaping search engine rankings. As AI technology continues to advance, it is crucial for search engines like Google to develop more sophisticated algorithms to identify and filter out spammy AI-generated content.
The ongoing battle against AI-generated spam
Search engines are continuously working to improve their spam detection algorithms in order to combat the rise of AI-generated spam. Google is investing resources in training its algorithms to better detect and filter out low-quality AI-generated content. However, it is a complex challenge due to the ever-evolving nature of AI technology.
Balancing the search engine’s ability to identify and penalize AI-generated spam with the need to avoid false positives poses a unique challenge. Striking the right balance is crucial to maintaining the integrity of search results and ensuring a positive user experience. Google’s ongoing efforts to address AI-generated spam are essential to the future of search engine optimization and content quality online.
The importance of vigilance and user feedback
While search engines like Google are taking proactive steps to combat AI-generated spam, user feedback remains a crucial component in identifying and addressing spammy content. Users are encouraged to report any suspicious AI-generated content they encounter in search results, helping search engines improve their algorithms and keep pace with evolving techniques used by spammers.
By staying vigilant and engaging in ongoing dialogue with search engines, users can play a vital role in fighting against the prevalence of AI-generated spam and ensuring the quality and relevance of search results.
Impact of AI-Generated Spam Challenges Solutions Dilutes search result quality Complex nature of AI-generated spam detection Investment in improved algorithms Negative impact on user experience Balance between avoiding false positives and spam detection User feedback and ongoing algorithm training Erodes trust in search engine reliability Constantly evolving AI technology Engaging users in reporting suspicious content
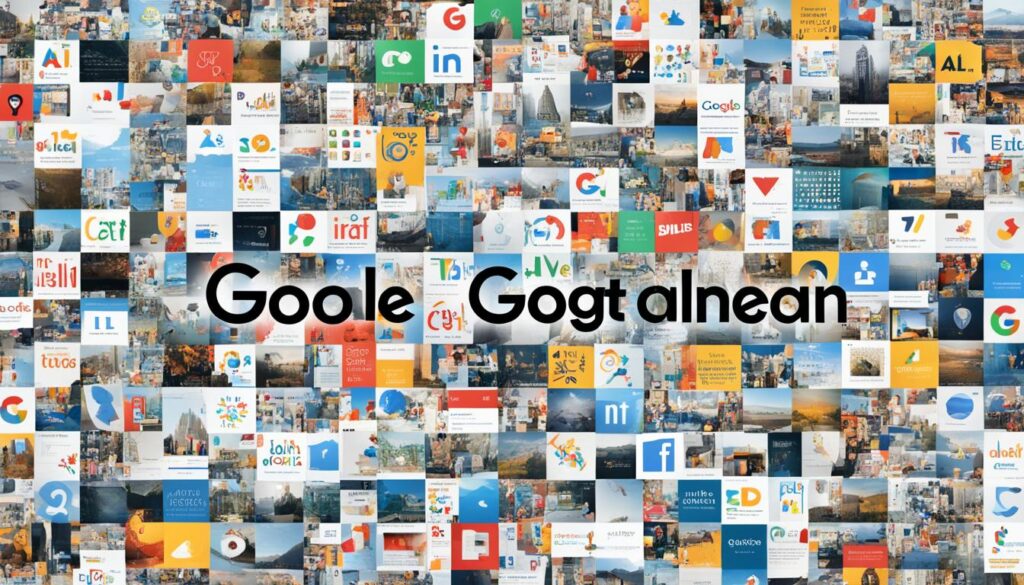
Google’s Ability to Determine Content Quality
Despite Google’s claims of being proficient at evaluating content quality, it continues to face challenges in distinguishing between good and great content. Many SEOs and website managers have observed instances where inferior content outranks superior content in search results. While Google has made progress in eliminating low-quality content from its index, there is still room for improvement when it comes to accurately assessing content quality.
Google has openly acknowledged that it does not possess a complete understanding of documents and relies on user interactions on search engine results pages (SERPs) to gauge content quality. The search engine prioritizes user feedback, such as click-through rates, time spent on a page, and bounce rates, to assess the relevance and usefulness of content to searchers.
“While Google has eliminated a significant amount of low-quality content from its index, it still faces challenges in distinguishing good content from great content.”
This reliance on user interactions is both a strength and a limitation for Google. On one hand, it allows the search engine to gather real-time feedback from users to inform its algorithms. On the other hand, it may lead to biased results as user behavior can be influenced by various factors. Additionally, Google’s algorithms may not always accurately capture the nuances of content quality, which can result in the promotion of subpar content over more valuable resources.
Furthermore, the rise of AI-generated content has posed new challenges for Google. As AI tools become more sophisticated, it becomes increasingly difficult for Google to differentiate between content produced by humans and by AI. This can result in AI-generated content that lacks depth or originality ranking higher than content created by experts in the field.
To address these challenges, Google constantly updates its algorithms to refine its understanding of content quality and improve its ability to rank high-quality content. However, website managers and content creators should also take an active role in ensuring their content adheres to Google’s guidelines for quality content, such as expertise, authoritativeness, and trustworthiness (E-A-T).
The Importance of User Interaction Metrics
Metric | Description |
---|---|
Click-through rate (CTR) | The percentage of users who click on a search result after seeing it on a SERP. |
Time spent on page | The average amount of time users spend on a webpage before returning to the SERP or navigating elsewhere. |
Bounce rate | The percentage of users who leave a webpage without interacting further with the site. |
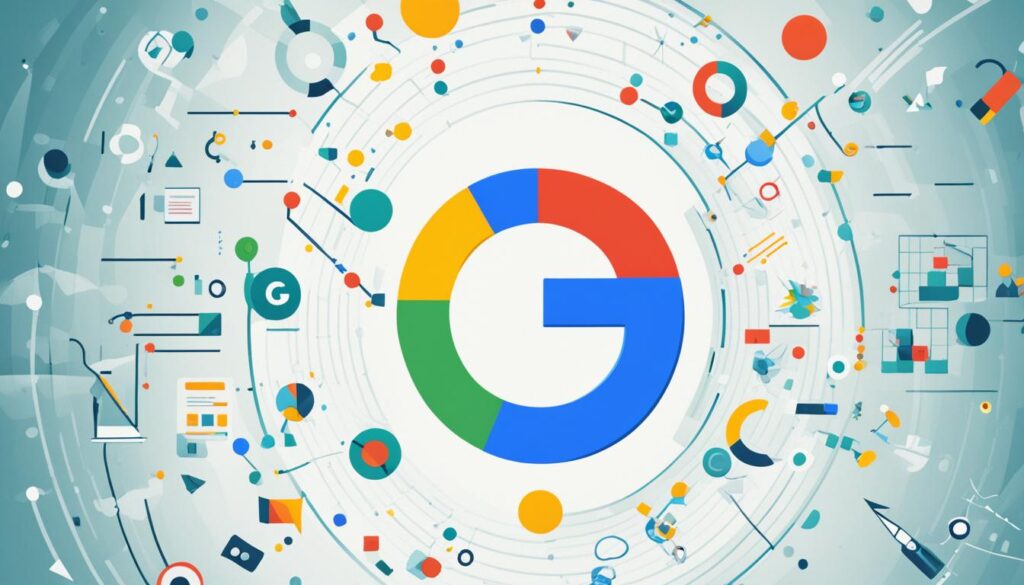
While user interaction metrics provide valuable insights into content quality, they are not the sole determinants of a webpage’s value. Content creators should strive to produce high-quality, informative, and engaging content that satisfies the needs and expectations of their target audience. By focusing on creating content that resonates with users and aligns with Google’s evolving quality guidelines, website managers can enhance their chances of ranking well in search results.
Google’s View on AI-Generated Content
Google takes a firm stance on AI-generated content, considering it spam that goes against its webmaster guidelines. Content produced by machines, also known as auto-generated content, is not favored by Google and can lead to manual penalties. The search engine’s systems may not be capable of detecting AI-generated content without human reviewers’ assistance. While there might be practical applications for AI writing tools, generating content using these tools is still considered spam according to Google’s guidelines.
The Challenge of AI-Generated Content Detection
Google faces significant challenges in distinguishing AI-generated content from human-created content. The increasing sophistication of AI writing tools has made it difficult to differentiate between the two. Despite Google’s efforts to combat spam, the prevalence of AI-generated content continues to be a concern. The search engine’s algorithms struggle to accurately assess the quality and relevance of AI-generated content, which can have negative implications for search results.
“AI-generated content presents a unique challenge for Google. While the search engine has made strides in evaluating content quality, AI-generated content remains a blind spot in its system.” – SEO Expert
Google’s ultimate goal is to provide users with valuable and reliable content. However, due to the nature of AI-generated content, the search engine has taken a strict approach in considering it spam. This approach is in line with Google’s continuous efforts to maintain the integrity of its search results and deliver the best possible user experience.

The Role of Human Reviewers
Recognizing the limitations of automated systems, Google relies on human reviewers to identify and assess AI-generated content accurately. These reviewers play a crucial role in evaluating the quality and relevance of content, ensuring that it meets Google’s guidelines and serves the best interests of users. The involvement of human reviewers reflects Google’s commitment to maintaining the integrity of its search results.
Exceptions and Ethical Considerations
While Google maintains a strict stance against AI-generated content, there are exceptions to consider. Some practical applications of AI writing tools, such as generating draft content for review and editing by humans, may be acceptable. However, it is important to navigate this territory with caution, ensuring that the content created aligns with Google’s guidelines and provides genuine value to users.
The Importance of Content Quality
Regardless of the methods used to create content, the fundamental importance of content quality remains unchanged. Google’s primary focus is to deliver high-quality, relevant content to its users. Creating content that is informative, well-researched, and tailored to users’ needs is essential for ranking well in search results, regardless of whether it is AI-generated or human-created.
The Use of AI Content Creation Tools
AI content creation tools, such as GPT-3, have sparked a heated debate within the SEO community regarding their effectiveness and ethical implications. Google’s Search Advocate John Mueller has taken a firm stance on the issue, stating that content generated using AI writing tools is considered spam and can result in penalties.
However, it is worth noting that Google’s systems may not be able to automatically detect AI-generated content, and their ability to differentiate between human-written and AI-written content is limited. This raises concerns about the effectiveness of Google’s algorithms in accurately judging the quality and origin of content.
While Google discourages the use of AI content creation tools, some reputable organizations continue to utilize these tools without encountering any issues. It is essential, however, to exercise caution and ensure that the content generated meets the quality standards set by Google.
The SEO Community’s Perspective
The SEO community remains divided on the use of AI content creation tools. Some argue that these tools can significantly enhance productivity and efficiency, enabling content creators to produce more engaging and informative pieces. Others, however, express concerns about the potential for low-quality, generic, or duplicate content flooding the web.
Despite these varying opinions, it is crucial for content creators to prioritize content quality above all else. Even though AI content creation tools may streamline certain aspects of the content creation process, they should complement human expertise rather than replace it entirely.
“While AI content creation tools can offer assistance, they should not be relied upon as a substitute for human creativity and expertise.” – Chris, SEO Expert at Ads and SEO
The Importance of Content Quality
Regardless of the tools used, the key to success in the eyes of Google remains delivering high-quality content that is valuable, relevant, and user-friendly. Meeting Google’s E-A-T (Expertise, Authoritativeness, Trustworthiness) guidelines should be the primary focus of content creators.
By combining the insights and efficiency provided by AI content creation tools with human expertise, content creators can strike a balance between optimization and ensuring that the content speaks directly to their target audience.
Ultimately, the debate surrounding the use of AI content creation tools will likely continue as AI technology evolves. It is crucial for content creators to stay informed about Google’s ever-changing guidelines and algorithms to ensure their content aligns with Google’s expectations.
Summary
The use of AI content creation tools, while controversial, can offer benefits in terms of efficiency and productivity. However, Google’s stance on AI-generated content remains clear – it is considered spam and can result in penalties. While some reputable organizations successfully use these tools, caution should be exercised to ensure the content generated meets high-quality standards.
Ultimately, content quality should always be the guiding principle in content creation, regardless of the tools used. Balancing AI assistance with human expertise is crucial to create engaging, informative, and user-friendly content that aligns with Google’s guidelines.
AI Content Labeling and Disclosure
Labeling and disclosure play important roles in providing transparency and clarity to users, especially when it comes to AI-generated content. While Google currently does not require labeling or disclosure of AI-generated content, there is an option to voluntarily provide this information if it is helpful for users.
By disclosing that a piece of content was generated by AI, users can have a better understanding of its origin and potentially adjust their expectations accordingly. However, it is essential to prioritize creating content that is helpful, reliable, and valuable for users, regardless of whether it is AI-generated or not.
“Transparency and accuracy are crucial in content creation. While labeling and disclosing AI-generated content can be beneficial, the most important aspect is producing high-quality content that meets the needs of the users.”
As discussions continue about the potential development of a policy for AI-generated content, Google’s current focus remains on evaluating the quality of the content rather than the fact that it was generated by AI. This emphasizes the importance of creating content that provides genuine value and meets the E-A-T (Expertise, Authoritativeness, Trustworthiness) guidelines, regardless of the content’s origin.
While AI technology continues to evolve and expand its capabilities, the goal should always be to prioritize the user’s experience. Creating content that is accurate, reliable, and tailored to the user’s needs remains essential, regardless of how it is produced. In a rapidly changing digital landscape, it is crucial for content creators to adapt and maintain a strong focus on delivering high-quality content.
Benefits of AI Content Labeling and Disclosure
Labeling and disclosing AI-generated content can provide several benefits:
- Transparency: Labeling AI-generated content helps users understand the technology used in its creation.
- Expectation Management: Providing disclosure can help users set appropriate expectations for the content.
- Choice and Preference: Some users may have a preference for human-generated content over AI-generated content.
- Enhanced User Trust: Transparent disclosure can build trust with users, showing a commitment to honesty and openness.
As the industry continues to explore best practices for AI-generated content, staying aligned with Google’s quality guidelines and providing valuable content will remain crucial for successful digital experiences.

Pros and Cons of AI Content Labeling and Disclosure
Pros | Cons |
---|---|
Provides transparency | May limit the reach of AI-generated content |
Helps manage user expectations | Can create biases against AI-generated content |
Offers choice and preference for users | May discourage the use of AI content creation tools |
Builds user trust through transparency | Can complicate the labeling process for content creators |
The Importance of People-First Content
When it comes to content creation, Google puts a strong emphasis on people-first content that is designed to cater to the needs of human users rather than search engines. This means prioritizing high-quality, relevant information that provides a positive user experience. While AI can certainly play a role in content creation, it is crucial to strike a balance between AI-generated content and human expertise.
Google’s guidelines, particularly the E-A-T (Expertise, Authoritativeness, Trustworthiness) guidelines, emphasize the importance of human-led content creation. While AI can assist in the production process, the human touch is essential to ensuring the depth, accuracy, and uniqueness of the content.
- People-first content: Content that is created with the primary goal of meeting the needs of human users. It should be informative, engaging, and valuable.
- Google’s guidelines: The set of rules and recommendations presented by Google to help content creators optimize their websites and improve their search rankings.
- AI: Artificial intelligence technology that can automate certain aspects of content creation but lacks the human understanding and creativity needed to produce truly exceptional content.
Google recognizes the limitations of AI-generated content and the importance of human expertise in delivering high-quality content. Content that is solely produced by AI may lack the depth, creativity, and critical thinking that connects with human readers. Therefore, it is essential to have human-led content creation where AI tools are used as aids rather than the sole source of content generation.
Striking a Balance
A balanced approach to content creation involves leveraging the capabilities of AI while retaining human expertise. AI can be used to streamline processes, analyze data, and identify trends, but it should always be complemented by human insight and creativity. This combination allows for the production of people-first content that meets Google’s guidelines and delivers value to users.
“The best content is a collaborative effort between AI and human creators, with each bringing their unique strengths to the table.” – Thorsten Meyer, Content Creator
The Power of People-First Content
Creating people-first content not only satisfies Google’s guidelines but also resonates with users. By focusing on delivering content that meets users’ needs, answers their questions, and provides value, content creators can establish trust and build long-term relationships with their audience. This, in turn, can lead to higher user engagement, increased visibility in search results, and ultimately, improved search rankings.
AI vs. Human Content Creation
AI Content Creation | Human Content Creation | |
---|---|---|
Strengths |
|
|
Limitations |
|
|
The table above provides a comparison between AI content creation and human content creation, showcasing their respective strengths and limitations. While AI offers efficiency and data-driven insights, human-led content creation brings the invaluable qualities of creativity, emotional intelligence, and critical thinking. By combining both approaches effectively, content creators can produce people-first content that is compelling, reliable, and enriching.
To summarize, when it comes to content creation, focusing on people-first content aligned with Google’s guidelines is essential. Human expertise should be at the forefront, with AI tools serving as aids to improve efficiency. Creating content that resonates with human readers, provides value, and meets the E-A-T guidelines is the key to success in Google’s search results.
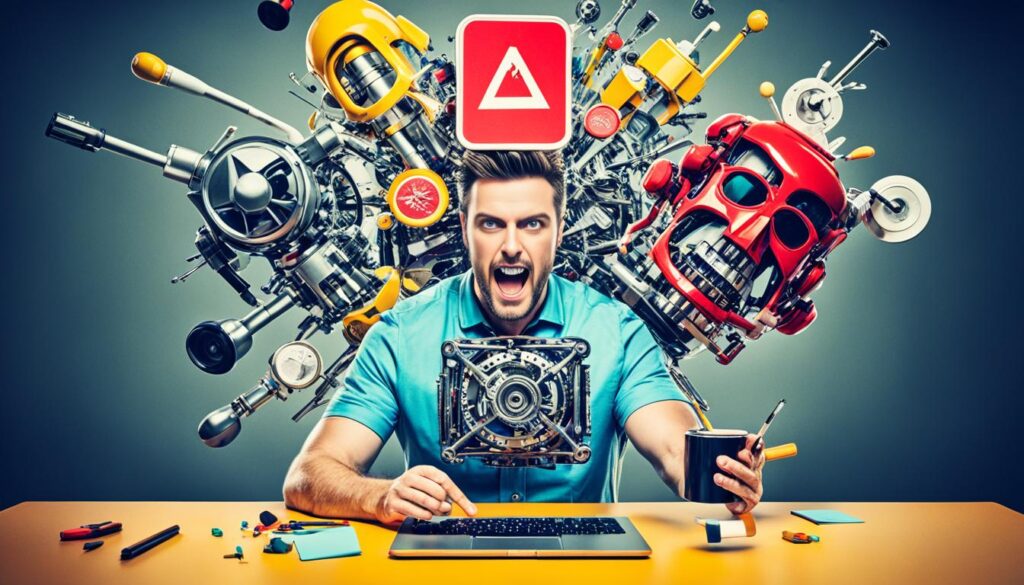
Google’s Future Policy on AI-Generated Content
Google is currently engaged in internal discussions regarding its response to the proliferation of AI-generated content. While the search engine giant may develop a comprehensive policy in the future, it is crucial for content creators to remain informed about Google’s evolving policies on AI content creation.
Google recognizes the potential role of AI in content creation but emphasizes that content quality and adherence to the E-A-T (Expertise, Authoritativeness, Trustworthiness) guidelines will always be paramount. In light of this, content creators should continue to prioritize human expertise and produce content that is valuable, reliable, and helpful to users.
As Google seeks to strike a balance between the benefits and challenges posed by AI-generated content, it is essential for content creators to stay up to date with any new policies and guidelines that may emerge. By staying informed, content creators can ensure that their AI-generated content aligns with Google’s quality standards, ultimately enhancing its visibility and relevance in search results.
To adapt and thrive in the evolving landscape of AI-generated content, content creators must remain flexible and responsive to Google’s future policy changes. By embracing human expertise and prioritizing the creation of high-quality content, content creators can continue to meet the expectations of both Google and users alike.
How to Win the Algorithm with AI Content
To win the algorithm with AI content, creating people-first content that is of high-quality, relevance, and meets Google’s E-A-T guidelines is essential. While AI can improve content efficiency and accuracy, human expertise must be involved to maintain the depth and uniqueness of the content. Staying updated on the latest SEO and content news is crucial to adapt to Google’s evolving algorithms and policies.
Creating People-First Content
When utilizing AI in content creation, it’s important to prioritize creating content that puts people first. This means focusing on providing value, relevance, and a positive user experience. AI should enhance the content creation process, but it should not replace the human touch. Incorporating human insights and expertise ensures that the content resonates with the intended audience and meets their needs.
Ensuring High-Quality Content
While AI can assist in improving content efficiency, it is crucial to prioritize content quality. AI content should be well-researched, accurate, and free from errors. Human involvement helps maintain the integrity of the information and ensures that it meets the expectations of the target audience. Quality content is more likely to engage readers, earn backlinks, and rank higher in search engine results.
Adhering to Google’s E-A-T Guidelines
Google’s E-A-T (Expertise, Authoritativeness, Trustworthiness) guidelines play a vital role in content quality evaluation. AI-generated content needs to demonstrate expertise in the subject matter, establish authority, and build trust with the audience. Human input in content creation helps establish credibility and ensures that the content aligns with Google’s E-A-T guidelines, increasing its chances of ranking higher in search results.
Keeping Up with SEO News
Google’s algorithms and policies are constantly evolving. Staying updated on the latest SEO and content news is essential to adapt to these changes. Following reputable SEO blogs, attending industry conferences, and engaging in relevant communities can provide valuable insights and ensure that AI content strategies align with the latest SEO best practices. Remaining agile and adaptable allows content creators to stay ahead of the curve and maximize the effectiveness of AI content in winning the algorithm.
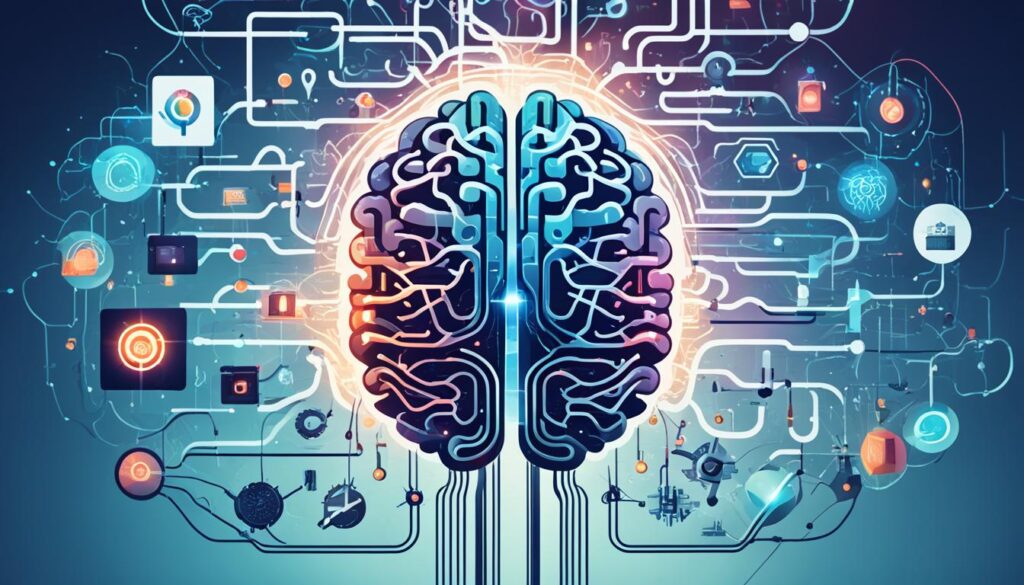
Benefits of Winning the Algorithm with AI Content | Key Considerations for Success |
---|---|
1. Higher organic rankings | 1. Prioritize human expertise in content creation |
2. Increased organic traffic | 2. Ensure content quality and relevance |
3. Improved user engagement | 3. Adhere to Google’s E-A-T guidelines |
4. Enhanced brand reputation | 4. Stay updated on SEO news and best practices |
Optimizing SEO Content with AI
AI can be a valuable tool for optimizing SEO content, providing various benefits such as keyword analysis, content generation, and data analysis. However, it is important to understand that AI should never replace human expertise but rather be used as a complementary resource.
When it comes to SEO content, AI can assist in identifying trending topics and popular keywords, allowing content creators to optimize their pages for maximum visibility. AI-powered tools can analyze large volumes of data to determine the most relevant keywords and phrases to include in the content.
Keyword Analysis
AI optimization tools can help identify the most effective keywords to target based on search volume and competition. By leveraging AI’s ability to process vast amounts of data, content creators can gain valuable insights into user search behavior and adapt their content strategy accordingly. This analysis can lead to more targeted and effective SEO campaigns.
Content Generation
AI-powered tools can generate content based on specific keywords and themes. These tools use natural language processing algorithms to create coherent and relevant text. Content creators can use AI-generated content as a starting point and then add their unique insights and expertise to produce high-quality, engaging content.
“AI-generated content can act as a foundation, but human expertise is crucial for adding depth and originality to the content,” emphasizes SEO expert Chris.
Data Analysis
AI can also assist in analyzing data related to user behavior, website traffic, and engagement metrics. By utilizing AI algorithms, content creators can gain valuable insights into their audience’s preferences, optimize user experience, and make data-driven decisions to improve content performance.
However, it is essential to strike a balance between AI and human expertise. While AI can automate certain tasks and provide data-driven insights, human creativity, critical thinking, and domain knowledge are necessary for producing high-quality, user-centric content that resonates with the target audience.
Remember that Google’s guidelines emphasize the importance of people-first content. By combining AI optimization with human expertise, content creators can create SEO-friendly content that meets both the search engine’s quality guidelines and user expectations.
So while AI can be a powerful tool in optimizing SEO content, it should always be used in conjunction with human expertise to ensure the content’s originality, relevance, and value to users.
Conclusion
Despite the challenges Google faces in handling AI-generated content, the search engine giant remains committed to prioritizing content quality and adhering to its E-A-T (Expertise, Authoritativeness, Trustworthiness) guidelines. AI can undoubtedly be a valuable tool in both content creation and optimization, but it should always be used in conjunction with human expertise.
Creating people-first content that is helpful, reliable, and valuable to users will continue to be the key to success in Google’s search results. While AI can assist in streamlining workflows and improving content efficiency, it cannot replace the depth and uniqueness that human knowledge and creativity bring to the table.
As Google’s policies and algorithms continue to evolve, staying updated on the latest developments is crucial for content creators. Adapting to the changing landscape of AI content and aligning with Google’s guidelines will ensure that your content remains visible and relevant in the ever-competitive online world.
FAQ
What challenges is Google facing with AI-generated content?
How prevalent is AI-generated content in Google search results?
How well can Google determine the quality of AI-generated content?
What is Google’s view on AI-generated content?
Can Google automatically detect AI-generated content?
Does Google require labeling or disclosure of AI-generated content?
What is the importance of people-first content according to Google?
What is Google’s future policy on AI-generated content?
How can AI content win the algorithm?
How can AI be used to optimize SEO content?
What is the conclusion regarding Google and AI-generated content?
Source Links
- https://www.searchenginejournal.com/google-says-ai-generated-content-is-against-guidelines/444916/
- https://thriveagency.com/news/how-google-treats-ai-content-and-should-it-be-labeled/
- https://searchengineland.com/googles-shifting-approach-ai-content-435601
In an era where technology intersects with every aspect of life, Maxwell bridges the gap between artificial intelligence and journalism. As a writer and AI expert, he explores the implications of emerging technologies on society, economy, and culture. Maxwell’s expertise allows Press Report to offer forward-thinking insights into the future of AI, making complex topics accessible and engaging for our audience.
-
AGI and AI Impact on Human Relationships6 days ago
Is the Threat of AI Overblown? Unpacking Myths
-
AI in Business3 weeks ago
AI at Work Beyond Cloud Cuts Company Costs
-
AGI and AI Impact on Human Relationships2 days ago
Is AGI a Threat to Humanity? Explore the Risks
-
AGI and AI Impact on Human Relationships2 weeks ago
AGI and AI’s Effect on Human Relationships
-
AGI and AI Impact on Human Relationships4 weeks ago
Impact of Artificial Intelligence on Humanity
-
AGI and AI Impact on Human Relationships3 weeks ago
1X Technologies Androids Built to Benefit Society
-
AGI and AI Impact on Human Relationships2 weeks ago
AI’s Influence on Society: Insights & Outlook
-
AGI and AI Impact on Human Relationships2 weeks ago
Consequences of Reaching AGI Unveiled