AI Security
Protecting AI Models: Strategies for Adversarial Attack Mitigation

We have all observed the incredible progress made in the field of artificial intelligence (AI) models. However, along with advancement, new obstacles emerge, especially concerning adversarial attacks.
Our article delves into the strategies for mitigating these attacks, equipping you with the knowledge to safeguard your AI models.
From detecting vulnerabilities to implementing preventive measures, we explore the technical nuances and analytical approaches necessary for mastery in protecting AI models.
Join us as we navigate the intricate world of adversarial attack mitigation.
Key Takeaways
- Adversarial attacks pose significant risks to the reliability and trustworthiness of AI systems.
- Vulnerabilities in AI models, such as lack of robustness and susceptibility to adversarial examples, raise concerns about their reliability.
- Techniques such as adversarial sample detection, statistical analysis of input data, and model confidence analysis can help detect adversarial attacks.
- Strategies for adversarial attack prevention, including robust AI model development, input sanitization techniques, and regular model retraining, are crucial for ensuring the robustness and reliability of AI systems.
Types of Adversarial Attacks
In our exploration of adversarial attacks, we’ll delve into the various types of attacks that can target AI models.
One type of attack is known as transferability attacks. These attacks aim to take advantage of the vulnerability of AI models to adversarial examples that can be transferred from one model to another. By crafting a malicious input that fools one model, an attacker can exploit the similarity of AI models and trick other models into making incorrect predictions as well.
Another type of attack is physical attacks. These attacks involve manipulating the physical world to deceive AI models. For example, by adding imperceptible perturbations to stop signs, an attacker can trick an AI-powered autonomous vehicle into misclassifying the sign, posing a significant risk to safety.
Understanding these types of attacks is crucial in developing effective defense strategies to protect AI models.
Vulnerabilities in AI Models
To continue our exploration of adversarial attacks, let’s examine the vulnerabilities present in AI models.
AI models are susceptible to various vulnerabilities that can be exploited by adversaries to manipulate or deceive the system. One of the key vulnerabilities is the lack of robustness in AI models, which means they’re sensitive to small perturbations or changes in input data that can lead to misclassification or incorrect predictions.
Another vulnerability is the reliance on training data, as AI models can be fooled by carefully crafted adversarial examples that are designed to mislead the model into making wrong predictions.
These vulnerabilities have a significant impact on AI development, as they raise concerns about the reliability and trustworthiness of AI systems.
To address these vulnerabilities, potential solutions include developing more robust models that are resistant to adversarial attacks, incorporating adversarial training during model training, and implementing techniques like input sanitization and anomaly detection to detect and mitigate adversarial attacks.
Techniques to Detect Adversarial Attacks
We employ robust detection techniques to identify adversarial attacks on AI models. In order to effectively detect these attacks, we utilize advanced methods that can analyze the behavior and characteristics of the input data.
Here are four key techniques that we employ:
- Adversarial Sample Detection – We use algorithms to detect if a sample is adversarial by comparing it to known normal samples. This helps us identify any malicious inputs that might’ve been crafted to deceive the AI model.
- Statistical Analysis – By analyzing statistical properties of the input data, such as mean, variance, and distribution, we can detect any deviations that might indicate the presence of an adversarial attack.
- Model Confidence Analysis – We analyze the confidence scores produced by the AI model for different inputs. Adversarial attacks often lead to low confidence scores, as the model struggles to correctly classify the manipulated inputs.
- Input Tampering Detection – We examine the integrity of the input data, looking for any signs of tampering or modifications. Any discrepancies found can indicate the presence of an adversarial attack.
Strategies for Adversarial Attack Prevention
Building upon our robust detection techniques, our team implements proactive strategies to prevent adversarial attacks on AI models. Adversarial attack countermeasures are crucial in ensuring the robustness and reliability of AI systems.
One effective strategy is to focus on robust AI model development. This involves employing techniques such as adversarial training, where the model is trained on both clean and adversarial data to improve its resilience against attacks.
Additionally, we employ input sanitization techniques to filter out potential adversarial inputs. By carefully analyzing and preprocessing the input data, we can identify and discard malicious inputs before they reach the model.
Regular model retraining is also essential to ensure continued robustness against evolving attack methods.
Through these proactive strategies, we aim to build AI models that aren’t only accurate and efficient but also resilient to adversarial attacks.
Response and Recovery Plans
As part of our comprehensive approach to protecting AI models, we’ve developed response and recovery plans to swiftly address and mitigate the impact of adversarial attacks. These plans are crucial in minimizing the damage caused by such attacks and ensuring the resilience of our AI systems.
Here are the key elements of our incident management strategy:
- Rapid Detection: We’ve implemented advanced monitoring techniques to promptly identify any signs of adversarial attacks and trigger an immediate response.
- Containment and Mitigation: Once an attack is detected, our response team swiftly takes action to contain the impact and mitigate further damage by isolating affected systems and limiting the attacker’s access.
- Forensic Investigation: Post-attack analysis is conducted to understand the nature and extent of the attack. This helps us identify vulnerabilities and implement necessary measures to strengthen our defenses.
- Recovery and Adaptation: After addressing the immediate threats, we focus on restoring the affected AI models, ensuring their integrity, and adapting our defenses based on lessons learned from the incident.
Frequently Asked Questions
Can AI Models Be Protected From All Types of Adversarial Attacks?
We cannot fully protect AI models from all types of adversarial attacks. Robustness testing and countermeasures implementation are crucial, but adversaries constantly evolve their techniques, making it a continuous challenge to ensure complete security.
How Can Vulnerabilities in AI Models Be Identified and Addressed?
Identifying and addressing vulnerabilities in AI models is akin to inspecting a fortress for weak points and fortifying them. We employ techniques like robust training, adversarial training, and model monitoring to ensure the resilience of our models.
Are There Any Techniques to Detect Adversarial Attacks That Are Not Mentioned in the Article?
Detection techniques for adversarial attacks can be enhanced by employing novel approaches that go beyond those mentioned in the article. These innovative methods can provide an extra layer of security to AI models, ensuring better protection against potential malicious attacks.
Besides Prevention, Are There Any Strategies for Mitigating the Impact of Successful Adversarial Attacks?
When it comes to protecting AI models, we must not only focus on prevention but also on strategies for impact mitigation and post-attack recovery. This ensures that we can effectively respond to successful adversarial attacks.
What Are Some Common Challenges Faced During the Response and Recovery Phase After an Adversarial Attack?
Response and recovery challenges after an adversarial attack include identifying the extent of the breach, restoring compromised systems, and mitigating further damage. The incident response process is crucial for timely detection and effective resolution.
Conclusion
In conclusion, protecting AI models from adversarial attacks requires a multi-faceted approach. By understanding the types of attacks and vulnerabilities, implementing techniques to detect attacks, and employing strategies for prevention, organizations can enhance the security of their AI systems.
Just as a fortress relies on multiple layers of defense mechanisms to keep intruders at bay, safeguarding AI models requires a thorough and comprehensive defense strategy.
Only by being vigilant and proactive can we ensure the integrity and reliability of AI technologies.
Bennett is the embodiment of versatility, adapting his writing to cover a broad spectrum of topics with professionalism and flair. Whether it’s breaking news, in-depth analyses, or feature pieces, Bennett’s contributions enrich Press Report with diverse perspectives and engaging content. His adaptability and keen journalistic instincts make him a vital member of our team, capable of capturing the essence of the moment in every story.
AI Security
Unlock the Benefits of AI-Powered Cybersecurity Today
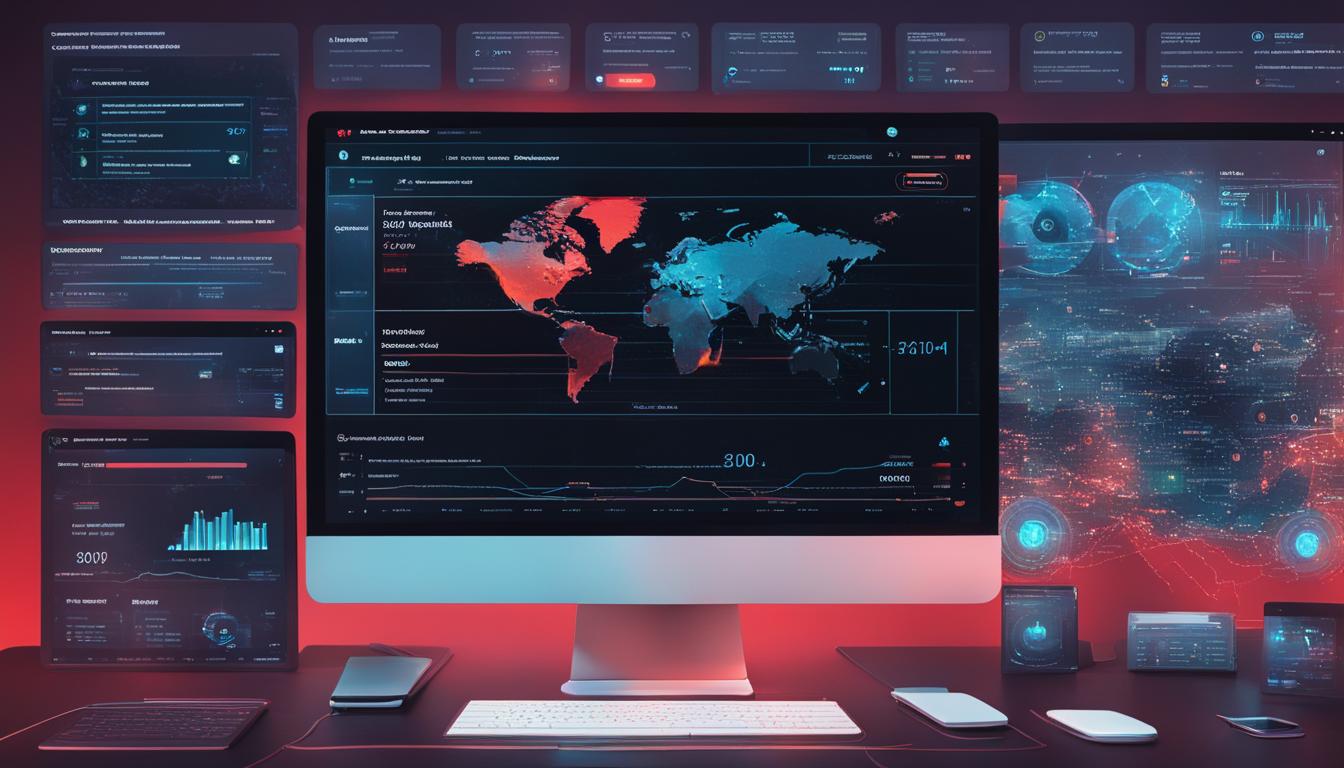
Join us as we delve into the benefits of AI-powered cybersecurity. In today’s digital age, enterprises face increasingly sophisticated cyber threats that can compromise sensitive data and disrupt operations. To combat these evolving challenges, AI has emerged as a critical element in cybersecurity, offering a range of advantages for detecting threats, managing incident responses, and improving security protocols overall.
In this article, we will explore how AI is revolutionizing the field of cybersecurity and the advantages it brings to organizations. From enhanced threat detection to automated incident response, AI-driven cybersecurity solutions are transforming the way we protect our digital assets.
Key Takeaways:
- AI-powered cybersecurity offers numerous benefits for organizations.
- AI can detect and respond to threats faster, automate processes, improve accuracy and efficiency, and provide scalability and cost savings.
- Implementing AI in cybersecurity can enhance incident response, identify potential threats more effectively, and proactively protect digital assets.
- Challenges of implementing AI in cybersecurity include data quality, adversarial attacks, and ethical considerations.
- The future of AI in cybersecurity holds great potential for protecting against evolving cyber threats.
Now, let’s delve into the role of AI in cybersecurity and discover how it is transforming the way we defend against cyberattacks.
The Role of AI in Cybersecurity
AI algorithms are revolutionizing the field of cybersecurity, enhancing organizations’ ability to protect against evolving threats. By employing AI in cybersecurity, we can detect anomalies and potential risks in real time, strengthening our overall defense mechanisms. AI algorithms have the power to process and interpret vast amounts of data from various sources, enabling us to identify patterns and behaviors indicative of cyberattacks.
One of the key advantages of AI in cybersecurity is the improvement in threat detection accuracy. AI-powered systems can analyze network traffic, user behavior, and system logs to identify suspicious activities that might go unnoticed by traditional methods. This allows us to proactively respond to potential threats before they cause significant damage. Additionally, AI can automate certain security tasks, such as analyzing log files and identifying vulnerabilities, which saves time and resources for organizations.
Another important aspect of AI in cybersecurity is its role in automated incident response. AI algorithms can swiftly analyze and assess the severity of security incidents, enabling us to take immediate action. This automated response capability helps to minimize the impact of cyberattacks and reduces overall response times. By leveraging AI in incident response, organizations can effectively mitigate threats and protect their digital assets.
Enhanced Cybersecurity with AI Algorithms
AI algorithms play a crucial role in enhancing cybersecurity defenses. They enable us to analyze vast amounts of data, detect anomalies, and automate incident response, thereby improving our overall security posture. With the ever-increasing complexity and sophistication of cyber threats, AI is becoming an indispensable tool in the fight against cybercrime.
Benefits of AI in Cybersecurity | Examples |
---|---|
Enhanced threat detection | Real-time analysis of network traffic for potential threats |
Automated incident response | Immediate action taken based on AI assessment of severity |
Improved accuracy and efficiency | AI algorithms can process and interpret large volumes of data with precision |
Cost savings | Automation of certain security tasks reduces the need for manual intervention |
By leveraging AI algorithms in cybersecurity, we can stay one step ahead of cybercriminals and protect our digital assets effectively. With the continuous advancements in AI technology, we anticipate even greater capabilities in the future, enabling us to combat emerging threats with more accuracy and efficiency.
AI algorithms enable us to process and interpret vast amounts of data, identify patterns, and detect potential cyber threats in real time. By automating certain security tasks and enhancing incident response capabilities, we can bolster our cybersecurity defenses and protect our digital assets.
Overall, the role of AI in cybersecurity is pivotal. Its ability to analyze data, detect anomalies, and automate processes provides organizations with a significant advantage in the fight against cyber threats. By embracing AI-powered cybersecurity solutions, we can enhance our overall security posture and ensure the integrity of our systems and data.
AI-Powered Solutions for Cybersecurity
In today’s rapidly evolving digital landscape, organizations are turning to AI-powered solutions to bolster their cybersecurity defenses. AI technology offers a range of benefits, enabling organizations to detect and respond to threats more effectively, automate processes, and enhance overall security. By harnessing the power of AI, organizations can stay one step ahead of cybercriminals and protect their valuable digital assets.
One significant application of AI in cybersecurity is through Security Information and Event Management (SIEM) systems. These systems leverage AI algorithms to analyze security incidents in real time, allowing organizations to identify patterns and anomalies indicative of cyber threats. By proactively monitoring and analyzing security events, organizations can swiftly detect and respond to potential breaches, minimizing the impact on their systems and data.
Another key AI-powered solution in cybersecurity is threat intelligence platforms. These platforms aggregate and analyze data from various sources, providing organizations with essential insights into emerging threats. By leveraging AI algorithms to process vast amounts of data, organizations gain a comprehensive understanding of the threat landscape, enabling them to implement proactive measures to mitigate potential risks.
AI-Powered Solutions for Cybersecurity | Benefits |
---|---|
Security Information and Event Management (SIEM) systems | Analyze security incidents in real time, identify patterns and anomalies indicative of cyber threats, proactive threat monitoring and response |
Threat intelligence platforms | Aggregate and analyze data from various sources, identify emerging threats, proactive risk mitigation |
Automated remediation systems | Initiate predefined actions to mitigate cybersecurity incidents, reduce response times and minimize the impact of attacks |
In addition to SIEM and threat intelligence, automated remediation systems powered by AI are invaluable in cybersecurity. These systems can automatically initiate predefined actions to mitigate cybersecurity incidents, significantly reducing response times and minimizing the impact of attacks. By leveraging AI-driven automation, organizations can swiftly and efficiently respond to threats, ensuring the integrity and security of their systems.
Overall, AI-powered solutions are revolutionizing the cybersecurity industry by providing organizations with enhanced capabilities to protect against cyber threats. Whether it’s through the real-time analysis of security incidents, comprehensive threat intelligence, or automated incident response, AI technology is proving to be a valuable asset in strengthening organizations’ cybersecurity defense strategies.
The Challenges of Implementing AI in Cybersecurity
Implementing AI in cybersecurity offers numerous benefits, but it also comes with its fair share of challenges. One of the key challenges is ensuring the quality of data used to train AI algorithms. Gathering sufficient and relevant data can be a daunting task for many organizations due to factors such as data silos and privacy concerns. Without high-quality data, AI algorithms may not be able to accurately detect and respond to cyber threats.
Another challenge is the potential for adversarial attacks, where cyber attackers manipulate AI systems to bypass security measures. Adversarial attacks exploit vulnerabilities in AI algorithms, making it difficult to trust the outputs and decisions made by AI-powered cybersecurity solutions. Organizations need to constantly stay vigilant and update their AI models to defend against emerging attack techniques.
Additionally, the generation of false positives can pose a challenge in AI-powered cybersecurity. False positives occur when an AI system incorrectly identifies benign activity as a potential threat, leading to unnecessary alerts and increased workload for cybersecurity teams. Organizations need to fine-tune their AI models to reduce false positives and ensure that they are not overwhelmed with irrelevant information.
Challenges of Implementing AI in Cybersecurity | Impact |
---|---|
Lack of quality data for training AI algorithms | Can lead to inaccurate threat detection and response |
Adversarial attacks on AI systems | Compromises trust in AI outputs and decisions |
Generation of false positives | Increases workload for cybersecurity teams |
Ethical and regulatory considerations also play a crucial role in implementing AI in cybersecurity. Organizations must ensure that AI-powered solutions adhere to ethical principles and legal frameworks to protect user privacy and prevent unintended consequences. Striking the right balance between innovation and responsible use of AI is essential in building trust and maintaining the integrity of cybersecurity practices.
Despite these challenges, organizations are increasingly adopting AI-powered cybersecurity solutions to bolster their defenses against cyber threats. By addressing the quality of data, guarding against adversarial attacks, and fine-tuning AI models to reduce false positives, organizations can harness the power of AI while mitigating the associated risks.
Benefits of AI in Threat Detection and Response
AI-driven threat detection and incident response have revolutionized the way organizations protect against cyberattacks. By leveraging AI technology, we can analyze large volumes of data and quickly identify abnormal behavior or potential risks. This allows us to respond in real time and mitigate the impact of cyber threats.
One of the key benefits of AI in threat detection is its ability to automate specific tasks. AI-powered systems can reroute traffic or isolate compromised systems, reducing response times and minimizing the damage caused by cyberattacks. These automated responses ensure that organizations can address threats promptly and effectively.
With AI-powered incident response, we can proactively protect against emerging threats and improve our overall cybersecurity posture. – Cybersecurity Expert
Another advantage of AI in threat detection and response is its ability to analyze vast amounts of data from various sources. By correlating different data points, AI algorithms can identify patterns and detect anomalies indicative of malicious activity. This enhanced data analysis enables organizations to detect and prevent cyber threats before they can cause significant damage.
Benefits of AI in Threat Detection and Response | Examples |
---|---|
Enhanced threat detection | AI algorithms can identify abnormal behavior and potential risks in real time. |
Automated incident response | AI-powered systems can initiate predefined actions to mitigate the impact of cyberattacks. |
Proactive protection | AI can proactively protect against emerging threats by analyzing vast amounts of data. |
Improved cybersecurity posture | By leveraging AI in threat detection and response, organizations can strengthen their overall security defenses. |
In conclusion, AI plays a crucial role in enhancing threat detection and incident response capabilities. By leveraging AI algorithms and automation, organizations can detect and respond to cyber threats more effectively and efficiently. The future of AI in cybersecurity is promising, as advancements in technology continue to strengthen our ability to protect against evolving cyber threats.
AI for Vulnerability Management
When it comes to managing vulnerabilities in today’s complex cybersecurity landscape, organizations are increasingly turning to AI-powered solutions. AI technology can analyze existing security measures, detect weak points, and prioritize critical security tasks, ultimately improving overall security posture. By leveraging AI algorithms, organizations can quickly scan devices and networks for potential vulnerabilities, providing more efficient and accurate results compared to manual processes.
One of the key advantages of using AI for vulnerability management is its ability to identify and mitigate vulnerabilities in real time. By continuously analyzing data and patterns, AI-powered systems can detect potential threats and vulnerabilities as they emerge, allowing for immediate response and remediation. This proactive approach helps organizations stay one step ahead of cyber attackers and minimize the risk of successful breaches.
To showcase the effectiveness of AI-powered vulnerability management, we have compiled a table highlighting the key features and benefits of this technology:
Key Features | Benefits |
---|---|
Automated vulnerability scanning | Reduces manual effort and improves efficiency |
Real-time threat detection | Enables immediate response and mitigation |
Prioritization of critical vulnerabilities | Focuses resources on high-risk areas |
Continuous monitoring and analysis | Identifies emerging threats and vulnerabilities |
Integration with existing security systems | Seamlessly fits into the organization’s cybersecurity framework |
By adopting AI-powered vulnerability management, organizations can effectively enhance their security defenses and protect against evolving cyber threats. However, it’s important to note that AI is not a standalone solution. It should be combined with other cybersecurity measures, such as regular patching, employee training, and strong access controls, to create a robust defense strategy.
In conclusion, AI-powered vulnerability management provides organizations with the ability to proactively detect and mitigate vulnerabilities, strengthen their security posture, and stay ahead in the ever-changing cybersecurity landscape. With continuous advancements in AI technology, we can expect further improvements in vulnerability management capabilities, helping organizations protect their valuable assets and data.
AI in User Authentication and Access Management
AI is revolutionizing the way organizations approach user authentication and access management. By leveraging AI technology, we can enhance security measures and strengthen protection against unauthorized access. AI-powered systems implement additional security layers, such as facial recognition and fingerprint scanners, ensuring the utmost security of sensitive information.
One of the key advantages of AI in user authentication is its ability to detect fraudulent login attempts. AI algorithms can analyze multiple parameters, such as user behavior and login patterns, to identify suspicious activities. This helps prevent credential stuffing and brute force attacks, safeguarding valuable data from potential threats.
With AI in access management, organizations can benefit from automated threat detection and response capabilities. AI-powered systems continuously monitor user activities and network traffic, correlating data points to identify anomalies indicative of malicious behavior. By leveraging real-time analytics and AI algorithms, organizations can proactively detect and respond to security incidents, minimizing the impact of potential breaches.
Table: AI in User Authentication and Access Management
Benefits of AI in User Authentication | Benefits of AI in Access Management |
---|---|
|
|
By embracing AI in user authentication and access management, organizations can ensure the security of their systems and protect against unauthorized access. The advanced capabilities of AI-powered systems enable organizations to stay one step ahead of potential threats, creating a robust and proactive cybersecurity framework.
AI for Fraud Detection and Prevention
AI technology has proven to be a valuable asset in the fight against fraud. By leveraging AI algorithms and machine learning techniques, organizations can analyze vast amounts of data and identify potential fraudulent activities in real time. From financial institutions to e-commerce platforms, businesses across various industries can benefit from AI-powered fraud detection and prevention solutions.
With AI, organizations can detect patterns and anomalies that may indicate fraudulent behavior. By correlating different data points and analyzing user behavior, AI-powered systems can proactively identify and mitigate fraud, protecting businesses and their customers from financial losses and reputational damage.
One of the key advantages of AI in fraud prevention is its ability to continuously learn and adapt. As fraudsters evolve their tactics, AI algorithms can evolve as well, staying one step ahead of new and emerging threats. This dynamic and adaptive nature of AI makes it a powerful tool in the ongoing battle against fraud.
The implementation of AI-powered fraud detection and prevention solutions can result in significant cost savings for businesses. By automating the detection process, organizations can reduce the need for manual monitoring and investigation, freeing up resources to focus on other critical areas of their operations.
Table: AI vs. Traditional Fraud Detection Methods
AI-powered Fraud Detection | Traditional Fraud Detection Methods |
---|---|
Uses advanced algorithms to analyze large amounts of data | Relies on manual review and investigation |
Detects anomalies and patterns indicative of fraudulent behavior | Relies on predefined rules and thresholds |
Can adapt and learn from new fraud patterns | Requires manual updates to detection rules |
Provides real-time detection and mitigation | May have delays in detecting and responding to fraud |
Offers scalability and cost savings | Can be resource-intensive and costly |
As technology continues to advance, AI will play an increasingly crucial role in fraud detection and prevention. By leveraging AI-powered solutions, organizations can protect their financial assets, maintain trust with their customers, and stay ahead of evolving fraud tactics.
AI-Powered Tools for Behavioral Analysis
AI plays a crucial role in analyzing user behavior and identifying anomalies that may indicate malicious activity. With AI-driven behavioral analysis, we can detect abnormal patterns and behaviors in real time, allowing for immediate response and threat mitigation. By leveraging AI algorithms, we enhance our ability to identify and respond to cyber threats, ensuring the integrity and security of our systems.
One of the key benefits of AI in behavioral analysis is its ability to process and analyze vast amounts of data from various sources. This enables us to detect subtle deviations in user behavior that could indicate potential security breaches. By leveraging AI, we can identify anomalies that may go unnoticed by traditional security measures, allowing us to take immediate action to mitigate risks.
Additionally, AI-powered tools provide us with the capability to continuously learn and adapt to evolving threats. By utilizing machine learning algorithms, we can train our AI systems to recognize new patterns and behaviors associated with emerging cyber threats. This proactive approach allows us to stay one step ahead of cybercriminals and protect our systems from sophisticated attacks.
Benefits of AI-Powered Tools for Behavioral Analysis | AI for Anomaly Detection |
---|---|
Enhanced threat detection capabilities | Detect abnormal patterns and behaviors |
Real-time response and threat mitigation | Immediate action to mitigate risks |
Processing and analyzing vast amounts of data | Identify subtle deviations in user behavior |
Continuous learning and adaptation to evolving threats | Stay one step ahead of cybercriminals |
By harnessing the power of AI in behavioral analysis, we can significantly enhance our cybersecurity defenses. With continuous monitoring and analysis of user behavior, we can detect and respond to potential threats in real time, minimizing the impact of cyberattacks. The combination of AI algorithms and machine learning techniques allows us to strengthen our overall cybersecurity posture and safeguard our digital assets.
The Future of AI in Behavioral Analysis
As AI technology continues to advance, the future of behavioral analysis holds even greater promise. The integration of AI with other cybersecurity measures, such as threat intelligence platforms and automated remediation systems, will further enhance our ability to detect and respond to cyber threats. Additionally, the development of AI algorithms that can identify and interpret complex behavioral patterns will provide us with deeper insights into potential risks and vulnerabilities.
Furthermore, the integration of AI-powered behavioral analysis with user authentication and access management systems will help us ensure the security of sensitive information and protect against unauthorized access. By combining various AI technologies, we can create a robust cybersecurity ecosystem that can adapt to evolving threats and provide comprehensive protection for our digital assets.
In conclusion, AI-powered tools for behavioral analysis offer significant advantages in identifying and mitigating cyber threats. By leveraging AI algorithms, we can detect abnormal patterns and behaviors, respond to potential risks in real time, and continuously adapt to emerging threats. The future of AI in behavioral analysis looks promising, as advancements in technology provide us with more accurate and efficient tools to protect against cybercrime.
AI for Network Segmentation and Security
AI-powered technologies are transforming the landscape of network security, providing organizations with advanced capabilities to protect their digital assets. By leveraging AI for network segmentation and security, we can enhance our defense strategies and guard against evolving cyber threats.
One of the key benefits of AI in network security is its ability to automate network segmentation. AI-powered systems can analyze network traffic patterns and identify potential security risks, allowing for the creation of secure zones within the network. This segmentation helps prevent unauthorized access to critical systems and data, minimizing the impact of potential breaches.
Additionally, AI can analyze network traffic in real-time, enabling organizations to detect and block malicious activities promptly. By leveraging AI algorithms, we can identify anomalies, such as unusual behavior or suspicious network traffic, and take immediate action to mitigate potential threats. This proactive approach enhances our overall cybersecurity defenses and ensures the integrity of our systems.
Benefits of AI for Network Segmentation and Security |
---|
Automated network segmentation |
Real-time analysis of network traffic |
Enhanced detection and mitigation of potential threats |
Prevention of unauthorized access to critical systems and data |
AI-powered technologies provide us with the tools to effectively segment our network and protect our digital assets from ever-evolving cyber threats. By automating network segmentation and analyzing network traffic in real-time, we can enhance our overall cybersecurity defenses and ensure the integrity of our systems.
In conclusion, leveraging AI for network segmentation and security unlocks numerous benefits for organizations. By automating network segmentation, analyzing network traffic in real-time, and proactively detecting and mitigating potential threats, we can fortify our cybersecurity defenses and protect against evolving cyber threats.
The Future of AI in Cybersecurity
The future of AI in cybersecurity is filled with immense potential and exciting advancements. As technology continues to evolve, AI will play an increasingly crucial role in protecting organizations against sophisticated cyber threats. With the constant development of AI algorithms and machine learning techniques, we can expect more accurate threat detection and automated response capabilities.
Advancements in AI will bolster organizations’ cybersecurity defenses by enabling them to stay one step ahead of emerging threats. AI-powered systems will have the ability to analyze and interpret vast amounts of data in real-time, detecting anomalies and potential risks with greater efficiency and effectiveness. This will allow organizations to proactively identify and mitigate cyber attacks, ensuring the integrity and security of their digital assets.
Furthermore, AI will continue to support organizations in their cybersecurity strategies by providing enhanced protection and defense mechanisms. By leveraging AI-powered cybersecurity solutions, organizations can harness the benefits of automated incident response, improved accuracy, and cost savings. With AI technology at their disposal, organizations will have the means to fortify their data protection strategies and maintain a strong cybersecurity posture.
AI Advancements in Cybersecurity
The field of AI in cybersecurity is continuously advancing, pushing the boundaries of what is possible. One key area of progress is the development of AI-powered threat intelligence platforms, which leverage machine learning to analyze data from various sources and identify emerging threats. These platforms enable proactive threat hunting and support decision-making processes to effectively combat cyber threats.
Additionally, advancements in AI algorithms will lead to better anomaly detection and behavioral analysis. AI-powered tools will be able to identify abnormal patterns and behaviors in real-time, allowing for immediate response and threat mitigation. This will significantly enhance organizations’ ability to detect and respond to threats, mitigating potential damage.
In conclusion, the future of AI in cybersecurity is bright. As technology continues to advance, AI will play a pivotal role in protecting organizations from increasingly sophisticated cyber threats. With advancements in AI algorithms and machine learning techniques, we can expect improved threat detection, automated incident response, and enhanced defense mechanisms. By embracing AI-powered cybersecurity solutions, organizations can ensure the integrity and security of their systems and stay one step ahead in the ever-evolving landscape of cybersecurity.
Advancements | Potential Impact |
---|---|
AI-powered threat intelligence platforms | Proactive threat hunting and effective decision-making |
Improved anomaly detection and behavioral analysis | Enhanced threat detection and response capabilities |
- Increased accuracy and efficiency in threat detection.
- Automated incident response, minimizing response times.
- Proactive identification and mitigation of emerging threats.
- Enhanced protection against evolving cyber threats.
Conclusion
AI-powered cybersecurity offers numerous benefits for organizations. By embracing this technology, we can enhance threat detection, automate incident response, improve accuracy and efficiency, and achieve cost savings. The future of AI in cybersecurity is promising, with continued advancements expected to strengthen our defenses against evolving cyber threats.
While implementing AI in cybersecurity may pose challenges, such as data quality and adversarial attacks, we can overcome them by addressing these issues proactively. Ensuring the quality of data used to train AI algorithms and implementing robust security measures will be crucial. Moreover, ethical considerations must be prioritized to ensure the responsible use of AI in cybersecurity.
As AI technology evolves, it will play an increasingly vital role in protecting against cyber threats. Advancements in AI algorithms and machine learning techniques will enable more accurate threat detection and automated response capabilities. By embracing AI-powered cybersecurity solutions, we can unlock the full benefits of this technology and fortify our data protection strategies.
FAQ
What are the benefits of AI-powered cybersecurity?
AI-powered cybersecurity offers enhanced threat detection, automated incident response, improved accuracy and efficiency, and cost savings for organizations.
How does AI play a role in cybersecurity?
AI algorithms enable organizations to process and interpret large amounts of data, identify anomalies and potential threats in real time, and improve overall cybersecurity defenses.
What are some AI-powered solutions for cybersecurity?
AI-powered solutions include Security Information and Event Management (SIEM) systems, threat intelligence platforms, and automated remediation systems.
What are the challenges of implementing AI in cybersecurity?
Challenges include the quality of data for training AI algorithms, adversarial attacks on AI systems, and addressing ethical and regulatory considerations.
How does AI enhance threat detection and response?
AI analyzes large amounts of data, identifies abnormal behavior or potential risks, and automates specific tasks to reduce response times and minimize the impact of cyberattacks.
How can AI help in vulnerability management?
AI can analyze existing security measures, identify vulnerabilities, and prioritize critical security tasks, providing more efficient and accurate results compared to manual processes.
What is the role of AI in user authentication and access management?
AI can implement additional security layers, detect fraudulent login attempts, and prevent credential stuffing and brute force attacks, ensuring the security of sensitive information.
How does AI assist in fraud detection and prevention?
AI analyzes data patterns, correlates different data points, and detects anomalies to proactively identify and mitigate fraudulent activities, protecting financial assets and maintaining trust.
How do AI-powered tools help in behavioral analysis?
AI algorithms analyze user behavior, detect abnormalities and malicious activity in real time, facilitating immediate response and threat mitigation.
How can AI improve network security?
AI automates network segmentation, analyzes network traffic for potential threats, and detects and blocks malicious activity, enhancing overall cybersecurity defenses.
What is the future of AI in cybersecurity?
Continual advancements in AI technology are expected to strengthen threat detection, automate response capabilities, and provide enhanced protection against evolving cyber threats.
Ava combines her extensive experience in the press industry with a profound understanding of artificial intelligence to deliver news stories that are not only timely but also deeply informed by the technological undercurrents shaping our world. Her keen eye for the societal impacts of AI innovations enables Press Report to provide nuanced coverage of technology-related developments, highlighting their broader implications for readers.
AI Security
How AI Will Contribute to Cybersecurity in 2024 Explained
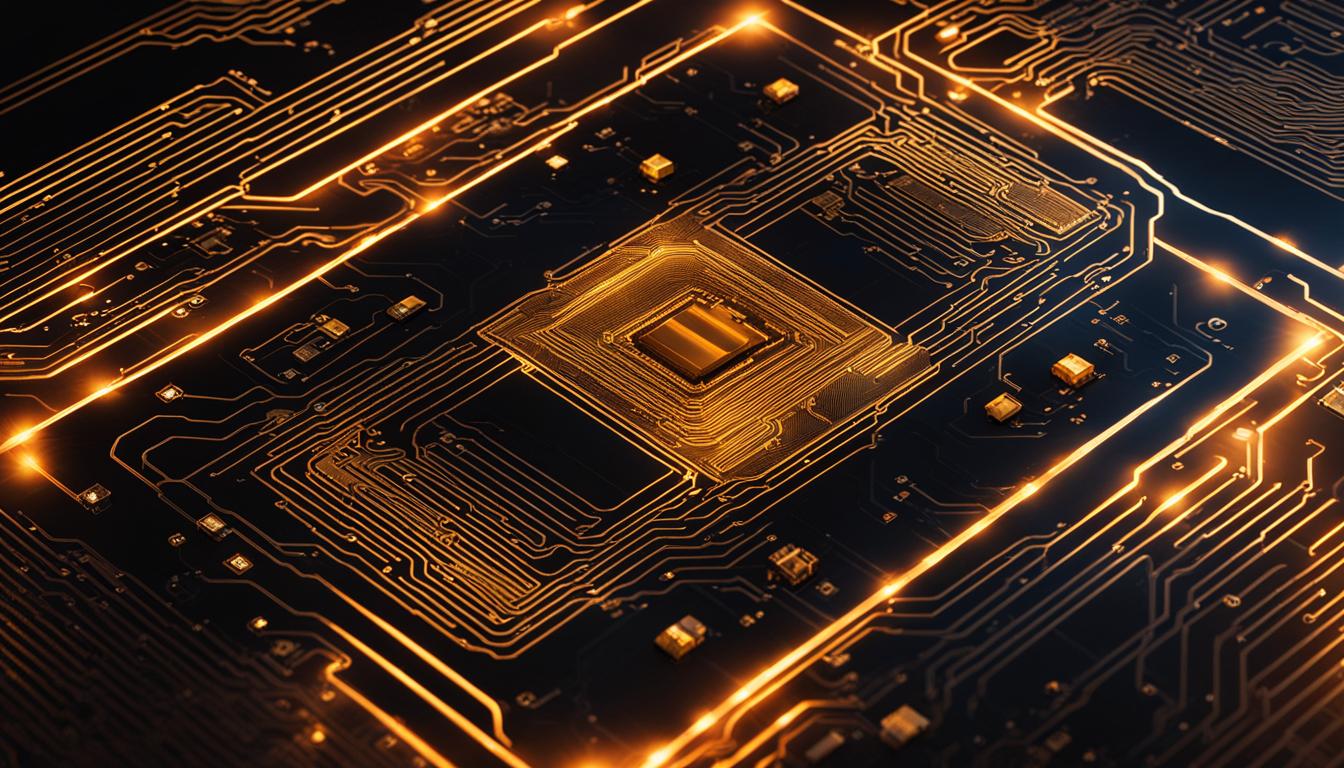
Welcome to our latest article, where we explore the exciting intersection of artificial intelligence (AI) and cybersecurity. As organizations increasingly adopt AI technology, a revolutionary change is taking place within the realm of cybersecurity. AI has the capability to replicate decision-making and problem-solving skills similar to those of humans, thanks to its powerful algorithms and computational capabilities. This piece seeks to investigate the impact of AI on cybersecurity and delve into the future prospects of this dynamic field.
AI brings a multitude of benefits to cybersecurity, including automation, predictive analysis, real-time threat detection, vulnerability management, and improved network security. These advancements can enhance our defenses against cyber threats and provide proactive solutions. However, as with any innovation, there are also challenges and risks to consider, such as investment costs and the need for skilled professionals.
Key Takeaways:
- AI is revolutionizing the field of cybersecurity with its ability to replicate human decision-making and problem-solving processes.
- Benefits of AI in cybersecurity include automation, predictive analysis, real-time threat detection, vulnerability management, and improved network security.
- Challenges and risks, such as investment costs and the need for skilled professionals, must also be considered.
- The future of cybersecurity with AI holds great promise, but organizations must navigate these challenges to fully harness its potential.
- By leveraging AI advancements, we can enhance our defenses and stay one step ahead of cyber threats.
What is AI?
Artificial Intelligence (AI) is a revolutionary technology that replicates human intelligence by utilizing complex algorithms, statistical models, and computational power. It enables machines to acquire knowledge, make decisions, and solve problems in a manner similar to humans. Through machine learning, AI systems can adapt and improve their performance over time, making them capable of handling complex tasks that surpass human capabilities.
AI technology works by processing vast amounts of data, evaluating patterns, and using this information to make predictions or decisions. It can perform tasks at a higher level and faster rate than humans, making it invaluable in many industries, including cybersecurity.
AI has various applications across different domains, such as natural language processing, computer vision, and robotics. In cybersecurity, AI plays a crucial role in automating processes, enhancing threat detection, and improving network security. By leveraging AI-powered solutions, organizations can benefit from automation, predictive analysis, real-time threat detection, vulnerability management, and improved overall cybersecurity.
AI Applications
AI finds applications in various areas, including:
- Automated Threat Detection: AI algorithms can analyze network traffic, identify patterns, and detect anomalies in real time, enabling organizations to respond quickly to potential threats.
- Vulnerability Management: AI-powered systems can automate the detection, identification, and remediation of known vulnerabilities, strengthening an organization’s security posture.
- Predictive Analysis: By analyzing historical and present data, AI can identify potential vulnerabilities and risks before they occur, allowing proactive measures to be taken to mitigate the impact.
- Enhanced Network Security: AI can monitor and track network traffic, detect anomalies, and manage endpoint lifecycle, providing a robust defense against cyber threats.
“AI technology is revolutionizing the field of cybersecurity, empowering organizations to automate processes, improve threat detection, and enhance overall network security.”
AI Benefits for Cybersecurity
Artificial Intelligence (AI) integrated with machine learning algorithms offers significant advantages for cybersecurity. By leveraging AI technology, organizations can enhance their defense mechanisms and strengthen their overall security posture. Let’s take a closer look at some key benefits of using AI in cybersecurity:
Automation in Cybersecurity
AI-powered automation simplifies and accelerates various cybersecurity processes. It eliminates manual tasks, such as data analysis, threat hunting, and incident response, allowing security professionals to focus their efforts on more strategic initiatives. With AI automation, organizations can detect and respond to threats in real-time, minimizing the impact of potential cyber attacks.
Predictive Analysis in Cybersecurity
AI’s predictive analysis capabilities enable organizations to proactively identify vulnerabilities and predict potential cyber threats. By analyzing historical and real-time data, AI algorithms can identify patterns and anomalies, providing early warnings of potential security breaches. This empowers cybersecurity teams to take preventive actions and implement timely security measures to mitigate risks.
Real-Time Threat Detection in Cybersecurity
AI-powered cybersecurity solutions excel at real-time threat detection. By continuously monitoring and analyzing network traffic, AI algorithms can identify and respond to suspicious activities in milliseconds. This enables organizations to detect and mitigate emerging threats promptly, reducing the vulnerability window and preventing potentially devastating cyber attacks.
Vulnerability Management in Cybersecurity
AI plays a critical role in vulnerability management by automating the detection, identification, and remediation of known vulnerabilities. Through continuous scanning and analysis, AI-powered systems can identify and prioritize vulnerabilities based on their potential impact and exploitability. This helps organizations efficiently allocate resources and address vulnerabilities before they can be exploited by threat actors.
Network Security with AI
AI enhances network security by monitoring and tracking network traffic in real-time. It can detect anomalies and suspicious activities that may indicate unauthorized access or potential security breaches. AI-powered network security solutions provide organizations with comprehensive visibility into their network infrastructure, enabling proactive defense measures and effective response to emerging threats.
Overall, AI brings numerous benefits to cybersecurity, from automation and predictive analysis to real-time threat detection and vulnerability management. By harnessing the power of AI, organizations can stay one step ahead of cyber threats and safeguard their critical assets.
Benefits | Description |
---|---|
Automation | AI streamlines cybersecurity processes and eliminates manual tasks. |
Predictive Analysis | AI enables proactive identification of vulnerabilities and prediction of threats. |
Real-Time Threat Detection | AI detects and responds to threats in real-time, minimizing the impact of cyber attacks. |
Vulnerability Management | AI automates the detection, identification, and remediation of known vulnerabilities. |
Network Security | AI enhances network security by monitoring and tracking network traffic. |
“AI brings numerous benefits to cybersecurity, from automation and predictive analysis to real-time threat detection and vulnerability management.”
AI Challenges and Risks in Cybersecurity
As AI technology continues to advance and be integrated into cybersecurity systems, it brings with it a set of challenges and risks that organizations must navigate. While the benefits of AI in cybersecurity are significant, it is important to be aware of the potential drawbacks and take proactive measures to address them.
One of the main challenges is the high investment costs associated with implementing AI cybersecurity solutions. The design and maintenance of AI systems require resources and expertise, which can be a barrier for smaller organizations with limited budgets. Additionally, training AI models with accurate and reliable data sets can be time-consuming and complex.
Another significant risk is the emergence of AI-powered attacks. Deepfake social engineering and automated malware are examples of how adversaries can leverage AI technology to create sophisticated and targeted cyber attacks. These attacks pose new and evolving risks that organizations need to be prepared to defend against.
Key Challenges and Risks in AI Cybersecurity
To summarize, the challenges and risks of using AI in cybersecurity are:
- High investment costs
- Complexity in designing and maintaining AI systems
- Time-consuming data training process
- Emergence of AI-powered attacks
It is crucial for organizations to ensure the ethical and responsible use of AI in cybersecurity. This involves implementing strong governance frameworks, adhering to established ethical guidelines, and continuously monitoring and updating AI systems to mitigate any potential risks. Furthermore, addressing the skills shortage in the cybersecurity industry and investing in training and education programs can help bridge the gap and ensure that organizations have the necessary expertise to leverage AI effectively and securely.
AI in cybersecurity offers numerous benefits, but organizations must be vigilant in understanding and mitigating the challenges and risks. It is only through a comprehensive approach that combines cutting-edge AI technology, skilled professionals, and robust governance that we can harness the power of AI to protect against evolving cyber threats.
Generative AI and AI-Powered Attacks
As we look into the future of cybersecurity, one area that stands out is the rise of generative AI and its impact on AI-powered attacks. Generative AI tools have the ability to accelerate the adoption of AI in cybersecurity, shaping the way attackers carry out their malicious activities. One notable example is the use of deepfake attacks, where AI technology is employed to create highly convincing and realistic fake content, such as videos or audio recordings.
Deepfake attacks pose a significant threat as they can be used for various malicious purposes, including social engineering, disinformation campaigns, and fraud. With the advancements in AI, attackers can now create more sophisticated and personalized attacks that are difficult to detect and defend against. These attacks not only exploit the vulnerabilities of individuals and organizations but also undermine trust and credibility in the digital realm.
To combat the growing threat of AI-powered attacks, organizations need to prioritize awareness, education, and the implementation of AI-based defense mechanisms. AI systems can help in identifying and mitigating deepfake attacks by analyzing patterns and inconsistencies in the content. Additionally, adopting a zero trust approach that continuously monitors and verifies the identity and behavior of users and devices can add an extra layer of protection against AI-powered attacks.
Challenges in Detecting Deepfake Attacks
Detecting deepfake attacks is a challenging task due to the sophistication of the technology involved. Traditional methods of authentication and verification may be insufficient in identifying manipulated content. As deepfake technology becomes more advanced, it is crucial for cybersecurity professionals to stay updated with the latest techniques and tools for detecting and preventing these attacks.
Table:
Challenges | Solutions |
---|---|
Lack of training data | Building large and diverse datasets for training AI models to detect deepfake content |
Evolution of deepfake technology | Continuous research and development of AI-based algorithms to counter emerging deepfake techniques |
Human judgment and visual inspection | Combining AI algorithms with human expertise to verify the authenticity of content |
By understanding the challenges and implementing effective solutions, we can better equip ourselves against the rising tide of generative AI and AI-powered attacks. It is crucial to foster collaboration between cybersecurity professionals, researchers, and policymakers to develop robust defense strategies and create a safer digital environment.
Next-Level Phishing Attacks
In today’s digital landscape, phishing attacks have become increasingly sophisticated, and the use of generative AI tools is poised to take these attacks to the next level. Attackers are now able to create smarter and more personalized approaches, including the use of deepfake technology. With generative AI, attackers can create convincing fake emails and messages that are tailored to deceive specific individuals or organizations.
Imagine receiving an email that appears to be from your CEO or a familiar colleague, but it’s actually an AI-generated message designed to trick you into revealing sensitive information or performing fraudulent actions. These deepfake phishing attacks can be difficult to detect and can have devastating consequences for individuals and organizations.
To mitigate the risk of next-level phishing attacks, organizations need to prioritize awareness and education. Employees should be trained to recognize the signs of phishing attempts and to verify the authenticity of emails and messages before taking any action. Implementing multi-factor authentication and encryption can also provide an added layer of security.
Example of a Phishing Attack email:
“Hello [Recipient’s Name],
I hope this email finds you well. I urgently need your assistance regarding a confidential matter. Please review the attached document and provide your feedback as soon as possible. Your prompt attention to this request is greatly appreciated.
Sincerely,
[Sender’s Name]
CEO, XYZ Corporation”
Furthermore, organizations can leverage AI systems to detect and respond to phishing attacks in real-time. AI-powered algorithms can analyze incoming emails and identify suspicious patterns or characteristics that indicate a potential phishing attempt. By combining AI technology with regular security updates and employee training, organizations can strengthen their defense against next-level phishing attacks.
Cybersecurity in the Board Room
In today’s digital landscape, cybersecurity is no longer just a technical concern. It has become a strategic priority that demands the attention and involvement of board directors. As organizations face increasingly sophisticated cyber threats, board members are recognizing the need to possess cybersecurity expertise and knowledge. The board room is now a critical space for discussions on cybersecurity governance and the responsibilities of board directors in ensuring the security and resilience of the organization’s digital assets.
Cybersecurity governance involves establishing policies, procedures, and frameworks to guide the organization’s approach to cybersecurity. Board directors play a crucial role in setting the strategic direction, overseeing risk management, and ensuring compliance with relevant regulations and industry standards. They are responsible for taking a proactive approach to cybersecurity, staying informed about the latest threats and trends, and making informed decisions to protect the organization against potential cybersecurity risks.
In recent years, boards have been devoting more time and resources to cybersecurity. They are seeking to broaden their knowledge and skills through specialized training programs and are increasingly collaborating with cybersecurity leaders and experts to enhance their understanding of the evolving threat landscape. However, there are areas where improvements can be made.
Cybersecurity Responsibilities of Board Directors
- Establishing a cybersecurity culture: Board directors should foster a cybersecurity-conscious culture within the organization, promoting a shared responsibility for cybersecurity among all employees.
- Third-party cybersecurity risk considerations: Boards need to ensure that third-party vendors and partners adhere to robust cybersecurity practices to minimize the risk of breaches through external connections.
- Cyber program maturity assessments: Regular assessments of the organization’s cybersecurity program are essential to identify areas for improvement and ensure the effectiveness of security measures.
In conclusion, the board room plays a critical role in shaping an organization’s cybersecurity strategy. Board directors need to develop a deep understanding of cybersecurity governance, take an active role in overseeing cybersecurity measures, and collaborate with cybersecurity leaders to effectively protect the organization against cyber threats.
Responsibilities of Board Directors | Actions |
---|---|
Establishing a cybersecurity culture | Foster a cybersecurity-conscious culture within the organization and promote a shared responsibility for cybersecurity among all employees. |
Third-party cybersecurity risk considerations | Ensure that third-party vendors and partners adhere to robust cybersecurity practices to minimize the risk of breaches through external connections. |
Cyber program maturity assessments | Conduct regular assessments of the organization’s cybersecurity program to identify areas for improvement and ensure the effectiveness of security measures. |
IoT Cyber Attacks
The increasing number of connected devices, including those used for remote work, poses significant cybersecurity risks. Internet of Things (IoT) devices often lack proper security protocols and can be vulnerable to attacks. Weak passwords and insecure operations make home consumer IoT devices especially at risk. It is crucial for organizations and individuals to prioritize the security of IoT devices and networks to mitigate these risks and protect sensitive data.
IoT cyber attacks can have severe consequences, including unauthorized access to personal information, disruption of critical infrastructure, and even physical harm. Attackers can exploit vulnerabilities in IoT devices to gain control over them, enabling them to launch distributed denial-of-service (DDoS) attacks, infiltrate networks, and gather sensitive data. Furthermore, compromised IoT devices can be used as entry points to launch attacks on other connected devices and systems.
To address these challenges, organizations and individuals should adopt best practices for securing IoT devices. This includes regularly updating firmware and software, using strong and unique passwords, disabling unnecessary features, and implementing network segmentation to isolate IoT devices from critical systems. It is also essential to stay informed about the latest security threats and vulnerabilities in IoT devices and implement appropriate security measures.
Key considerations for securing IoT devices:
- Implement strong authentication mechanisms, such as two-factor authentication, to prevent unauthorized access to IoT devices.
- Regularly update firmware and software to ensure devices are protected against known vulnerabilities.
- Disable unnecessary features and services that may introduce additional security risks.
- Segment IoT devices from critical systems to minimize the potential impact of a compromised device.
- Monitor network traffic and behavior of IoT devices for any signs of suspicious activity.
By taking proactive steps to secure IoT devices, organizations and individuals can mitigate the cybersecurity risks associated with the increasing number of connected devices and safeguard their data and privacy.
Cyber Resilience – Beyond Cyber Security
In the ever-evolving landscape of cybersecurity, it’s crucial to go beyond traditional security measures and embrace cyber resilience. While cybersecurity focuses on preventing attacks, cyber resilience ensures the continuity of operations even after a breach. It’s about being prepared for the inevitable, adapting to emerging threats, and quickly recovering from disruptions.
At its core, cyber resilience means understanding that breaches will happen and focusing on minimizing the impact. It involves building robust systems that can withstand attacks, detecting and responding to incidents promptly, and recovering operations swiftly. Cyber resilience goes beyond technical solutions; it encompasses organizational culture, processes, and a proactive mindset.
In today’s digital world, businesses must prioritize cyber resilience to safeguard their operations and protect sensitive data. By investing in technologies, such as AI-powered threat intelligence and real-time monitoring, organizations can detect and respond to threats more effectively. Additionally, implementing backup and recovery mechanisms ensures the continuity of critical operations, minimizing downtime and data loss in the event of an incident.
Resilience in Cybersecurity
Resilience in cybersecurity means being able to adapt and respond to ever-changing threats, evolving technologies, and emerging attack vectors. It involves proactive planning, regular assessments, and continuous improvement to strengthen security postures. Cyber resilience also entails fostering a culture of cybersecurity awareness and empowering employees to respond effectively to potential incidents.
Ensuring cyber resilience requires collaboration across departments and stakeholders, as well as ongoing evaluation and adaptation of security measures. By embracing cyber resilience, organizations can better navigate the complex and evolving cybersecurity landscape, ensuring the continuity of operations and minimizing the impact of breaches.
Continuity of Operations
The continuity of operations is a critical aspect of cyber resilience. It encompasses the ability to maintain essential business functions and services during and after a cyber incident. Organizations must have robust plans in place to ensure that operations can continue, even if systems are compromised or disrupted.
This includes having backup systems, data recovery processes, and clear communication channels to keep stakeholders informed. Regular testing and simulation exercises help identify gaps and weaknesses in continuity plans, allowing for timely improvements. By prioritizing continuity of operations, organizations can minimize the financial, reputational, and operational impacts of cyber incidents.
Less Than Zero Trust
In the ever-evolving landscape of cybersecurity, the concept of zero trust has gained momentum as organizations seek to strengthen their defenses against sophisticated cyber threats. Zero trust challenges the traditional notion of a secure perimeter and recognizes that not all users or devices within a network can be trusted. As we look ahead to 2024, zero trust is set to take on a new dimension, becoming more adaptive and holistic, enabled by continuous AI-powered real-time authentication and activity monitoring.
Continuous authentication is a key component of the less than zero trust model. Rather than relying solely on a one-time authentication process, continuous authentication ensures that users are continuously and dynamically authenticated throughout their session. This helps to prevent unauthorized access and identify any suspicious activities in real time. By continuously monitoring user behavior, such as keystrokes, mouse movements, and device interactions, organizations can detect anomalies and respond promptly to potential threats.
Activity monitoring is another important aspect of the less than zero trust model. It involves the constant monitoring and analysis of user activities and network traffic. By analyzing activity logs and network data, organizations can identify patterns, detect unusual behaviors, and respond to potential security incidents in a timely manner. Activity monitoring also provides valuable insights into user behavior, which can be used to enhance security policies and training programs.
Implementing the less than zero trust model requires a shift in mindset and a comprehensive approach to cybersecurity. It involves a combination of technology, processes, and people. Organizations need to invest in advanced AI-powered authentication solutions and deploy robust monitoring and analysis tools. They also need to implement strong access controls, enforce the principle of least privilege, and provide ongoing cybersecurity training to employees. By adopting the less than zero trust model, organizations can enhance their overall network security and better protect their critical assets.
As cyber threats continue to evolve and become more sophisticated, the less than zero trust approach provides a proactive and adaptive defense strategy. By continuously authenticating users and monitoring activities, organizations can significantly reduce the risk of unauthorized access and potential security breaches. As we move into 2024, adopting the less than zero trust model will be crucial in ensuring the security and resilience of organizations’ digital infrastructure.
Cyber Warfare and State-Sponsored Attacks
In today’s interconnected world, cyber warfare has become a prominent tool for states in both military operations and political influence. State-sponsored cyber attacks can range from disruptive tactics to undermine infrastructure to targeted attacks aimed at compromising sensitive information. These attacks often involve sophisticated techniques that exploit vulnerabilities in network systems and employ advanced malware.
One significant area where cyber attacks have been prevalent is in military operations. Cyber warfare has become an integral part of modern warfare, with states employing cyber attacks to disrupt enemy communications, compromise critical infrastructure, and gain strategic advantages. These attacks can have severe consequences, impacting military capabilities and potentially leading to physical destruction.
Another area where state-sponsored cyber attacks have gained attention is in elections. Major elections around the world have been targeted by cyber attackers seeking to manipulate voter sentiment, compromise electoral systems, and undermine the democratic process. These attacks pose a significant threat to the integrity of democratic elections and can have far-reaching implications for national security and international relations.
“Cyber attacks by states have the potential to cause significant damage and disrupt critical systems. It is crucial for governments and organizations to enhance their defenses and response capabilities to mitigate the risks posed by state-sponsored cyber attacks.”
Cyber Attacks in 2024: Trends and Challenges
In the coming years, the frequency and sophistication of state-sponsored cyber attacks are expected to increase. As technology advances and the digital landscape evolves, new vulnerabilities will emerge, creating opportunities for cyber attackers to exploit. It is imperative that governments and organizations remain vigilant and proactive in strengthening their cybersecurity measures to counter these threats.
Furthermore, international cooperation and diplomatic efforts will play a crucial role in combating cyber warfare. Collaborative initiatives will be necessary to establish norms and regulations in cyberspace, promote responsible behavior, and facilitate information sharing between nations. By working together, we can better protect our critical infrastructure, defend against state-sponsored attacks, and safeguard the stability of the digital world.
Conclusion
In reflecting on the cybersecurity landscape in 2024, it is evident that the future of cybersecurity is heavily intertwined with the advancements in AI. The use of AI in cybersecurity will continue to revolutionize the field, offering automation, predictive analysis, real-time threat detection, vulnerability management, and improved network security.
However, while AI brings numerous benefits, it also presents challenges and risks. Organizations must address the skills shortage and invest in cybersecurity professionals with technical and soft skills. Additionally, board directors play a crucial role in cybersecurity governance, and their expertise and involvement are becoming increasingly important.
The rising number of IoT devices and evolving cyber threats call for enhanced security measures. Securing IoT devices and networks should be a priority for organizations to mitigate cybersecurity risks. Furthermore, regulations and compliance will continue to impact cybersecurity practices, requiring organizations to stay up-to-date with the latest standards.
Overall, the future of cybersecurity is a dynamic and ever-evolving landscape. With AI as a transformative force, both defending against attacks and developing more sophisticated attack techniques will be shaped by its advancements. As we move forward, it is crucial that we stay informed, adaptable, and proactive in addressing the emerging cybersecurity trends in 2024 and beyond.
FAQ
What is AI?
AI, or artificial intelligence, refers to technology that simulates human intelligence by replicating decision-making and problem-solving processes. It uses complex algorithms, statistical models, and computational power to acquire and use knowledge and improve performance over time.
What are the benefits of AI for cybersecurity?
AI offers numerous benefits for cybersecurity, including automation of processes, time-saving capabilities, enhanced detection of malware variants, predictive analysis to identify vulnerabilities and risks, real-time threat detection, vulnerability management, and improved network security.
What are the challenges and risks of using AI in cybersecurity?
Some challenges and risks of using AI in cybersecurity include high investment costs, the need for resources and expertise to design and maintain AI systems, time-consuming training of AI models with accurate data sets, and the emergence of AI-powered attacks, such as deepfake social engineering and automated malware.
How can generative AI impact cybersecurity?
Generative AI tools can enable smarter and more personalized cyber attacks, such as deepfake attacks. These AI-powered attacks range from deepfake social engineering to automated malware that adapts to evade detection. Defense against these attacks requires organization-wide awareness, education, and the use of AI and zero trust principles.
What are the risks associated with next-level phishing attacks?
With the use of generative AI tools, phishing attacks can become more sophisticated and personalized, including the use of deepfake techniques. Organizations need to prioritize awareness and education to mitigate the risk of phishing attacks. AI systems and zero trust principles can also play a role in preventing and responding to these attacks.
How can boards contribute to cybersecurity governance?
Boards can contribute to cybersecurity governance by ensuring they have members with cybersecurity expertise, devoting time to cybersecurity discussions, seeking to broaden their knowledge and skills, and increasing collaboration with cybersecurity leaders. They should also consider third-party cybersecurity risk and assess the maturity of their cyber programs.
What are the cybersecurity risks associated with IoT devices?
The increasing number of connected devices, including those used for remote work, poses cybersecurity risks. IoT devices often lack proper security protocols and can be vulnerable to attacks. Weak passwords and insecure operations make home consumer IoT devices especially at risk. Organizations should prioritize securing IoT devices and networks.
What is cyber resilience?
Cyber resilience is an important aspect of cybersecurity that focuses on the ability to recover and continue operations in the event of a breach. While cybersecurity aims to prevent attacks, resilience measures ensure business continuity even after a successful breach. Organizations should prioritize developing the capability to recover quickly, minimize data loss and downtime, and adapt to evolving threats.
What is the concept of zero trust in cybersecurity?
Zero trust challenges the notion of a secure perimeter and extends beyond the corporate network to remote workers, partners, and IoT devices. In 2024, zero trust becomes adaptive and holistic, enabled by continuous AI-powered real-time authentication and activity monitoring. It helps mitigate the risk of insider threats and improve overall network security.
How prevalent are cyber attacks in military and civilian operations?
Cyber warfare is increasingly used by states in military and civilian operations. Common tactics include phishing attacks and distributed denial-of-service attacks. In 2024, cyber attacks are expected to be prevalent in military operations worldwide, and major elections may face increased cyber threats. Governments and organizations need to enhance their defenses and response capabilities against state-sponsored attacks.
What can we expect in the cybersecurity landscape in 2024?
The cybersecurity landscape in 2024 will be shaped by advancements in AI and the increasing importance of cyber resilience. Organizations need to address the skills shortage and invest in cybersecurity professionals with both technical and soft skills. Board directors play a crucial role in cybersecurity governance. The rising number of IoT devices and evolving cyber threats require enhanced security measures. Regulations and compliance will continue to impact cybersecurity practices. Overall, AI will have a transformative impact on cybersecurity, both in defending against attacks and in the development of more sophisticated attack techniques.
Bennett is the embodiment of versatility, adapting his writing to cover a broad spectrum of topics with professionalism and flair. Whether it’s breaking news, in-depth analyses, or feature pieces, Bennett’s contributions enrich Press Report with diverse perspectives and engaging content. His adaptability and keen journalistic instincts make him a vital member of our team, capable of capturing the essence of the moment in every story.
AI Security
Unlock the Benefits of AI-Powered Cybersecurity Today
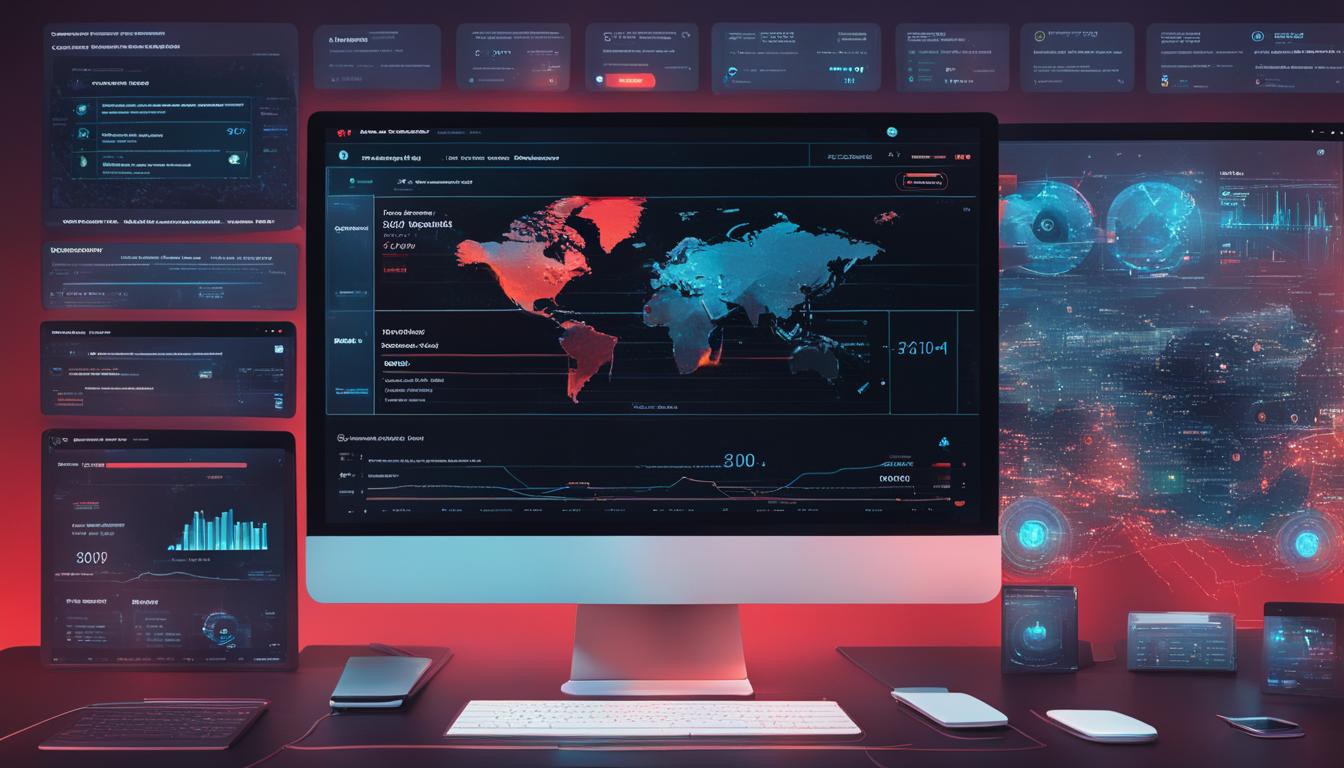
Welcome to our spotlight on the benefits of utilizing artificial intelligence in cybersecurity. In today’s digital landscape, companies face increasingly sophisticated and changing cyber threats that endanger sensitive data and could disrupt business operations. In tackling these issues, artificial intelligence is emerging as a vital tool in the area of cybersecurity, offering a wide range of advantages in identifying threats, reacting to breaches, and improving security protocols overall.
In this article, we will explore how AI is revolutionizing the field of cybersecurity and the advantages it brings to organizations. From enhanced threat detection to automated incident response, AI-driven cybersecurity solutions are transforming the way we protect our digital assets.
Key Takeaways:
- AI-powered cybersecurity offers numerous benefits for organizations.
- AI can detect and respond to threats faster, automate processes, improve accuracy and efficiency, and provide scalability and cost savings.
- Implementing AI in cybersecurity can enhance incident response, identify potential threats more effectively, and proactively protect digital assets.
- Challenges of implementing AI in cybersecurity include data quality, adversarial attacks, and ethical considerations.
- The future of AI in cybersecurity holds great potential for protecting against evolving cyber threats.
Now, let’s delve into the role of AI in cybersecurity and discover how it is transforming the way we defend against cyberattacks.
The Role of AI in Cybersecurity
AI algorithms are revolutionizing the field of cybersecurity, enhancing organizations’ ability to protect against evolving threats. By employing AI in cybersecurity, we can detect anomalies and potential risks in real time, strengthening our overall defense mechanisms. AI algorithms have the power to process and interpret vast amounts of data from various sources, enabling us to identify patterns and behaviors indicative of cyberattacks.
One of the key advantages of AI in cybersecurity is the improvement in threat detection accuracy. AI-powered systems can analyze network traffic, user behavior, and system logs to identify suspicious activities that might go unnoticed by traditional methods. This allows us to proactively respond to potential threats before they cause significant damage. Additionally, AI can automate certain security tasks, such as analyzing log files and identifying vulnerabilities, which saves time and resources for organizations.
Another important aspect of AI in cybersecurity is its role in automated incident response. AI algorithms can swiftly analyze and assess the severity of security incidents, enabling us to take immediate action. This automated response capability helps to minimize the impact of cyberattacks and reduces overall response times. By leveraging AI in incident response, organizations can effectively mitigate threats and protect their digital assets.
Enhanced Cybersecurity with AI Algorithms
AI algorithms play a crucial role in enhancing cybersecurity defenses. They enable us to analyze vast amounts of data, detect anomalies, and automate incident response, thereby improving our overall security posture. With the ever-increasing complexity and sophistication of cyber threats, AI is becoming an indispensable tool in the fight against cybercrime.
Benefits of AI in Cybersecurity | Examples |
---|---|
Enhanced threat detection | Real-time analysis of network traffic for potential threats |
Automated incident response | Immediate action taken based on AI assessment of severity |
Improved accuracy and efficiency | AI algorithms can process and interpret large volumes of data with precision |
Cost savings | Automation of certain security tasks reduces the need for manual intervention |
By leveraging AI algorithms in cybersecurity, we can stay one step ahead of cybercriminals and protect our digital assets effectively. With the continuous advancements in AI technology, we anticipate even greater capabilities in the future, enabling us to combat emerging threats with more accuracy and efficiency.
AI algorithms enable us to process and interpret vast amounts of data, identify patterns, and detect potential cyber threats in real time. By automating certain security tasks and enhancing incident response capabilities, we can bolster our cybersecurity defenses and protect our digital assets.
Overall, the role of AI in cybersecurity is pivotal. Its ability to analyze data, detect anomalies, and automate processes provides organizations with a significant advantage in the fight against cyber threats. By embracing AI-powered cybersecurity solutions, we can enhance our overall security posture and ensure the integrity of our systems and data.
AI-Powered Solutions for Cybersecurity
In today’s rapidly evolving digital landscape, organizations are turning to AI-powered solutions to bolster their cybersecurity defenses. AI technology offers a range of benefits, enabling organizations to detect and respond to threats more effectively, automate processes, and enhance overall security. By harnessing the power of AI, organizations can stay one step ahead of cybercriminals and protect their valuable digital assets.
One significant application of AI in cybersecurity is through Security Information and Event Management (SIEM) systems. These systems leverage AI algorithms to analyze security incidents in real time, allowing organizations to identify patterns and anomalies indicative of cyber threats. By proactively monitoring and analyzing security events, organizations can swiftly detect and respond to potential breaches, minimizing the impact on their systems and data.
Another key AI-powered solution in cybersecurity is threat intelligence platforms. These platforms aggregate and analyze data from various sources, providing organizations with essential insights into emerging threats. By leveraging AI algorithms to process vast amounts of data, organizations gain a comprehensive understanding of the threat landscape, enabling them to implement proactive measures to mitigate potential risks.
AI-Powered Solutions for Cybersecurity | Benefits |
---|---|
Security Information and Event Management (SIEM) systems | Analyze security incidents in real time, identify patterns and anomalies indicative of cyber threats, proactive threat monitoring and response |
Threat intelligence platforms | Aggregate and analyze data from various sources, identify emerging threats, proactive risk mitigation |
Automated remediation systems | Initiate predefined actions to mitigate cybersecurity incidents, reduce response times and minimize the impact of attacks |
In addition to SIEM and threat intelligence, automated remediation systems powered by AI are invaluable in cybersecurity. These systems can automatically initiate predefined actions to mitigate cybersecurity incidents, significantly reducing response times and minimizing the impact of attacks. By leveraging AI-driven automation, organizations can swiftly and efficiently respond to threats, ensuring the integrity and security of their systems.
Overall, AI-powered solutions are revolutionizing the cybersecurity industry by providing organizations with enhanced capabilities to protect against cyber threats. Whether it’s through the real-time analysis of security incidents, comprehensive threat intelligence, or automated incident response, AI technology is proving to be a valuable asset in strengthening organizations’ cybersecurity defense strategies.
The Challenges of Implementing AI in Cybersecurity
Implementing AI in cybersecurity offers numerous benefits, but it also comes with its fair share of challenges. One of the key challenges is ensuring the quality of data used to train AI algorithms. Gathering sufficient and relevant data can be a daunting task for many organizations due to factors such as data silos and privacy concerns. Without high-quality data, AI algorithms may not be able to accurately detect and respond to cyber threats.
Another challenge is the potential for adversarial attacks, where cyber attackers manipulate AI systems to bypass security measures. Adversarial attacks exploit vulnerabilities in AI algorithms, making it difficult to trust the outputs and decisions made by AI-powered cybersecurity solutions. Organizations need to constantly stay vigilant and update their AI models to defend against emerging attack techniques.
Additionally, the generation of false positives can pose a challenge in AI-powered cybersecurity. False positives occur when an AI system incorrectly identifies benign activity as a potential threat, leading to unnecessary alerts and increased workload for cybersecurity teams. Organizations need to fine-tune their AI models to reduce false positives and ensure that they are not overwhelmed with irrelevant information.
Challenges of Implementing AI in Cybersecurity | Impact |
---|---|
Lack of quality data for training AI algorithms | Can lead to inaccurate threat detection and response |
Adversarial attacks on AI systems | Compromises trust in AI outputs and decisions |
Generation of false positives | Increases workload for cybersecurity teams |
Ethical and regulatory considerations also play a crucial role in implementing AI in cybersecurity. Organizations must ensure that AI-powered solutions adhere to ethical principles and legal frameworks to protect user privacy and prevent unintended consequences. Striking the right balance between innovation and responsible use of AI is essential in building trust and maintaining the integrity of cybersecurity practices.
Despite these challenges, organizations are increasingly adopting AI-powered cybersecurity solutions to bolster their defenses against cyber threats. By addressing the quality of data, guarding against adversarial attacks, and fine-tuning AI models to reduce false positives, organizations can harness the power of AI while mitigating the associated risks.
Benefits of AI in Threat Detection and Response
AI-driven threat detection and incident response have revolutionized the way organizations protect against cyberattacks. By leveraging AI technology, we can analyze large volumes of data and quickly identify abnormal behavior or potential risks. This allows us to respond in real time and mitigate the impact of cyber threats.
One of the key benefits of AI in threat detection is its ability to automate specific tasks. AI-powered systems can reroute traffic or isolate compromised systems, reducing response times and minimizing the damage caused by cyberattacks. These automated responses ensure that organizations can address threats promptly and effectively.
With AI-powered incident response, we can proactively protect against emerging threats and improve our overall cybersecurity posture. – Cybersecurity Expert
Another advantage of AI in threat detection and response is its ability to analyze vast amounts of data from various sources. By correlating different data points, AI algorithms can identify patterns and detect anomalies indicative of malicious activity. This enhanced data analysis enables organizations to detect and prevent cyber threats before they can cause significant damage.
Benefits of AI in Threat Detection and Response | Examples |
---|---|
Enhanced threat detection | AI algorithms can identify abnormal behavior and potential risks in real time. |
Automated incident response | AI-powered systems can initiate predefined actions to mitigate the impact of cyberattacks. |
Proactive protection | AI can proactively protect against emerging threats by analyzing vast amounts of data. |
Improved cybersecurity posture | By leveraging AI in threat detection and response, organizations can strengthen their overall security defenses. |
In conclusion, AI plays a crucial role in enhancing threat detection and incident response capabilities. By leveraging AI algorithms and automation, organizations can detect and respond to cyber threats more effectively and efficiently. The future of AI in cybersecurity is promising, as advancements in technology continue to strengthen our ability to protect against evolving cyber threats.
AI for Vulnerability Management
When it comes to managing vulnerabilities in today’s complex cybersecurity landscape, organizations are increasingly turning to AI-powered solutions. AI technology can analyze existing security measures, detect weak points, and prioritize critical security tasks, ultimately improving overall security posture. By leveraging AI algorithms, organizations can quickly scan devices and networks for potential vulnerabilities, providing more efficient and accurate results compared to manual processes.
One of the key advantages of using AI for vulnerability management is its ability to identify and mitigate vulnerabilities in real time. By continuously analyzing data and patterns, AI-powered systems can detect potential threats and vulnerabilities as they emerge, allowing for immediate response and remediation. This proactive approach helps organizations stay one step ahead of cyber attackers and minimize the risk of successful breaches.
To showcase the effectiveness of AI-powered vulnerability management, we have compiled a table highlighting the key features and benefits of this technology:
Key Features | Benefits |
---|---|
Automated vulnerability scanning | Reduces manual effort and improves efficiency |
Real-time threat detection | Enables immediate response and mitigation |
Prioritization of critical vulnerabilities | Focuses resources on high-risk areas |
Continuous monitoring and analysis | Identifies emerging threats and vulnerabilities |
Integration with existing security systems | Seamlessly fits into the organization’s cybersecurity framework |
By adopting AI-powered vulnerability management, organizations can effectively enhance their security defenses and protect against evolving cyber threats. However, it’s important to note that AI is not a standalone solution. It should be combined with other cybersecurity measures, such as regular patching, employee training, and strong access controls, to create a robust defense strategy.
In conclusion, AI-powered vulnerability management provides organizations with the ability to proactively detect and mitigate vulnerabilities, strengthen their security posture, and stay ahead in the ever-changing cybersecurity landscape. With continuous advancements in AI technology, we can expect further improvements in vulnerability management capabilities, helping organizations protect their valuable assets and data.
AI in User Authentication and Access Management
AI is revolutionizing the way organizations approach user authentication and access management. By leveraging AI technology, we can enhance security measures and strengthen protection against unauthorized access. AI-powered systems implement additional security layers, such as facial recognition and fingerprint scanners, ensuring the utmost security of sensitive information.
One of the key advantages of AI in user authentication is its ability to detect fraudulent login attempts. AI algorithms can analyze multiple parameters, such as user behavior and login patterns, to identify suspicious activities. This helps prevent credential stuffing and brute force attacks, safeguarding valuable data from potential threats.
With AI in access management, organizations can benefit from automated threat detection and response capabilities. AI-powered systems continuously monitor user activities and network traffic, correlating data points to identify anomalies indicative of malicious behavior. By leveraging real-time analytics and AI algorithms, organizations can proactively detect and respond to security incidents, minimizing the impact of potential breaches.
Table: AI in User Authentication and Access Management
Benefits of AI in User Authentication | Benefits of AI in Access Management |
---|---|
|
|
By embracing AI in user authentication and access management, organizations can ensure the security of their systems and protect against unauthorized access. The advanced capabilities of AI-powered systems enable organizations to stay one step ahead of potential threats, creating a robust and proactive cybersecurity framework.
AI for Fraud Detection and Prevention
AI technology has proven to be a valuable asset in the fight against fraud. By leveraging AI algorithms and machine learning techniques, organizations can analyze vast amounts of data and identify potential fraudulent activities in real time. From financial institutions to e-commerce platforms, businesses across various industries can benefit from AI-powered fraud detection and prevention solutions.
With AI, organizations can detect patterns and anomalies that may indicate fraudulent behavior. By correlating different data points and analyzing user behavior, AI-powered systems can proactively identify and mitigate fraud, protecting businesses and their customers from financial losses and reputational damage.
One of the key advantages of AI in fraud prevention is its ability to continuously learn and adapt. As fraudsters evolve their tactics, AI algorithms can evolve as well, staying one step ahead of new and emerging threats. This dynamic and adaptive nature of AI makes it a powerful tool in the ongoing battle against fraud.
The implementation of AI-powered fraud detection and prevention solutions can result in significant cost savings for businesses. By automating the detection process, organizations can reduce the need for manual monitoring and investigation, freeing up resources to focus on other critical areas of their operations.
Table: AI vs. Traditional Fraud Detection Methods
AI-powered Fraud Detection | Traditional Fraud Detection Methods |
---|---|
Uses advanced algorithms to analyze large amounts of data | Relies on manual review and investigation |
Detects anomalies and patterns indicative of fraudulent behavior | Relies on predefined rules and thresholds |
Can adapt and learn from new fraud patterns | Requires manual updates to detection rules |
Provides real-time detection and mitigation | May have delays in detecting and responding to fraud |
Offers scalability and cost savings | Can be resource-intensive and costly |
As technology continues to advance, AI will play an increasingly crucial role in fraud detection and prevention. By leveraging AI-powered solutions, organizations can protect their financial assets, maintain trust with their customers, and stay ahead of evolving fraud tactics.
AI-Powered Tools for Behavioral Analysis
AI plays a crucial role in analyzing user behavior and identifying anomalies that may indicate malicious activity. With AI-driven behavioral analysis, we can detect abnormal patterns and behaviors in real time, allowing for immediate response and threat mitigation. By leveraging AI algorithms, we enhance our ability to identify and respond to cyber threats, ensuring the integrity and security of our systems.
One of the key benefits of AI in behavioral analysis is its ability to process and analyze vast amounts of data from various sources. This enables us to detect subtle deviations in user behavior that could indicate potential security breaches. By leveraging AI, we can identify anomalies that may go unnoticed by traditional security measures, allowing us to take immediate action to mitigate risks.
Additionally, AI-powered tools provide us with the capability to continuously learn and adapt to evolving threats. By utilizing machine learning algorithms, we can train our AI systems to recognize new patterns and behaviors associated with emerging cyber threats. This proactive approach allows us to stay one step ahead of cybercriminals and protect our systems from sophisticated attacks.
Benefits of AI-Powered Tools for Behavioral Analysis | AI for Anomaly Detection |
---|---|
Enhanced threat detection capabilities | Detect abnormal patterns and behaviors |
Real-time response and threat mitigation | Immediate action to mitigate risks |
Processing and analyzing vast amounts of data | Identify subtle deviations in user behavior |
Continuous learning and adaptation to evolving threats | Stay one step ahead of cybercriminals |
By harnessing the power of AI in behavioral analysis, we can significantly enhance our cybersecurity defenses. With continuous monitoring and analysis of user behavior, we can detect and respond to potential threats in real time, minimizing the impact of cyberattacks. The combination of AI algorithms and machine learning techniques allows us to strengthen our overall cybersecurity posture and safeguard our digital assets.
The Future of AI in Behavioral Analysis
As AI technology continues to advance, the future of behavioral analysis holds even greater promise. The integration of AI with other cybersecurity measures, such as threat intelligence platforms and automated remediation systems, will further enhance our ability to detect and respond to cyber threats. Additionally, the development of AI algorithms that can identify and interpret complex behavioral patterns will provide us with deeper insights into potential risks and vulnerabilities.
Furthermore, the integration of AI-powered behavioral analysis with user authentication and access management systems will help us ensure the security of sensitive information and protect against unauthorized access. By combining various AI technologies, we can create a robust cybersecurity ecosystem that can adapt to evolving threats and provide comprehensive protection for our digital assets.
In conclusion, AI-powered tools for behavioral analysis offer significant advantages in identifying and mitigating cyber threats. By leveraging AI algorithms, we can detect abnormal patterns and behaviors, respond to potential risks in real time, and continuously adapt to emerging threats. The future of AI in behavioral analysis looks promising, as advancements in technology provide us with more accurate and efficient tools to protect against cybercrime.
AI for Network Segmentation and Security
AI-powered technologies are transforming the landscape of network security, providing organizations with advanced capabilities to protect their digital assets. By leveraging AI for network segmentation and security, we can enhance our defense strategies and guard against evolving cyber threats.
One of the key benefits of AI in network security is its ability to automate network segmentation. AI-powered systems can analyze network traffic patterns and identify potential security risks, allowing for the creation of secure zones within the network. This segmentation helps prevent unauthorized access to critical systems and data, minimizing the impact of potential breaches.
Additionally, AI can analyze network traffic in real-time, enabling organizations to detect and block malicious activities promptly. By leveraging AI algorithms, we can identify anomalies, such as unusual behavior or suspicious network traffic, and take immediate action to mitigate potential threats. This proactive approach enhances our overall cybersecurity defenses and ensures the integrity of our systems.
Benefits of AI for Network Segmentation and Security |
---|
Automated network segmentation |
Real-time analysis of network traffic |
Enhanced detection and mitigation of potential threats |
Prevention of unauthorized access to critical systems and data |
AI-powered technologies provide us with the tools to effectively segment our network and protect our digital assets from ever-evolving cyber threats. By automating network segmentation and analyzing network traffic in real-time, we can enhance our overall cybersecurity defenses and ensure the integrity of our systems.
In conclusion, leveraging AI for network segmentation and security unlocks numerous benefits for organizations. By automating network segmentation, analyzing network traffic in real-time, and proactively detecting and mitigating potential threats, we can fortify our cybersecurity defenses and protect against evolving cyber threats.
The Future of AI in Cybersecurity
The future of AI in cybersecurity is filled with immense potential and exciting advancements. As technology continues to evolve, AI will play an increasingly crucial role in protecting organizations against sophisticated cyber threats. With the constant development of AI algorithms and machine learning techniques, we can expect more accurate threat detection and automated response capabilities.
Advancements in AI will bolster organizations’ cybersecurity defenses by enabling them to stay one step ahead of emerging threats. AI-powered systems will have the ability to analyze and interpret vast amounts of data in real-time, detecting anomalies and potential risks with greater efficiency and effectiveness. This will allow organizations to proactively identify and mitigate cyber attacks, ensuring the integrity and security of their digital assets.
Furthermore, AI will continue to support organizations in their cybersecurity strategies by providing enhanced protection and defense mechanisms. By leveraging AI-powered cybersecurity solutions, organizations can harness the benefits of automated incident response, improved accuracy, and cost savings. With AI technology at their disposal, organizations will have the means to fortify their data protection strategies and maintain a strong cybersecurity posture.
AI Advancements in Cybersecurity
The field of AI in cybersecurity is continuously advancing, pushing the boundaries of what is possible. One key area of progress is the development of AI-powered threat intelligence platforms, which leverage machine learning to analyze data from various sources and identify emerging threats. These platforms enable proactive threat hunting and support decision-making processes to effectively combat cyber threats.
Additionally, advancements in AI algorithms will lead to better anomaly detection and behavioral analysis. AI-powered tools will be able to identify abnormal patterns and behaviors in real-time, allowing for immediate response and threat mitigation. This will significantly enhance organizations’ ability to detect and respond to threats, mitigating potential damage.
In conclusion, the future of AI in cybersecurity is bright. As technology continues to advance, AI will play a pivotal role in protecting organizations from increasingly sophisticated cyber threats. With advancements in AI algorithms and machine learning techniques, we can expect improved threat detection, automated incident response, and enhanced defense mechanisms. By embracing AI-powered cybersecurity solutions, organizations can ensure the integrity and security of their systems and stay one step ahead in the ever-evolving landscape of cybersecurity.
Advancements | Potential Impact |
---|---|
AI-powered threat intelligence platforms | Proactive threat hunting and effective decision-making |
Improved anomaly detection and behavioral analysis | Enhanced threat detection and response capabilities |
- Increased accuracy and efficiency in threat detection.
- Automated incident response, minimizing response times.
- Proactive identification and mitigation of emerging threats.
- Enhanced protection against evolving cyber threats.
Conclusion
AI-powered cybersecurity offers numerous benefits for organizations. By embracing this technology, we can enhance threat detection, automate incident response, improve accuracy and efficiency, and achieve cost savings. The future of AI in cybersecurity is promising, with continued advancements expected to strengthen our defenses against evolving cyber threats.
While implementing AI in cybersecurity may pose challenges, such as data quality and adversarial attacks, we can overcome them by addressing these issues proactively. Ensuring the quality of data used to train AI algorithms and implementing robust security measures will be crucial. Moreover, ethical considerations must be prioritized to ensure the responsible use of AI in cybersecurity.
As AI technology evolves, it will play an increasingly vital role in protecting against cyber threats. Advancements in AI algorithms and machine learning techniques will enable more accurate threat detection and automated response capabilities. By embracing AI-powered cybersecurity solutions, we can unlock the full benefits of this technology and fortify our data protection strategies.
FAQ
What are the benefits of AI-powered cybersecurity?
AI-powered cybersecurity offers enhanced threat detection, automated incident response, improved accuracy and efficiency, and cost savings for organizations.
How does AI play a role in cybersecurity?
AI algorithms enable organizations to process and interpret large amounts of data, identify anomalies and potential threats in real time, and improve overall cybersecurity defenses.
What are some AI-powered solutions for cybersecurity?
AI-powered solutions include Security Information and Event Management (SIEM) systems, threat intelligence platforms, and automated remediation systems.
What are the challenges of implementing AI in cybersecurity?
Challenges include the quality of data for training AI algorithms, adversarial attacks on AI systems, and addressing ethical and regulatory considerations.
How does AI enhance threat detection and response?
AI analyzes large amounts of data, identifies abnormal behavior or potential risks, and automates specific tasks to reduce response times and minimize the impact of cyberattacks.
How can AI help in vulnerability management?
AI can analyze existing security measures, identify vulnerabilities, and prioritize critical security tasks, providing more efficient and accurate results compared to manual processes.
What is the role of AI in user authentication and access management?
AI can implement additional security layers, detect fraudulent login attempts, and prevent credential stuffing and brute force attacks, ensuring the security of sensitive information.
How does AI assist in fraud detection and prevention?
AI analyzes data patterns, correlates different data points, and detects anomalies to proactively identify and mitigate fraudulent activities, protecting financial assets and maintaining trust.
How do AI-powered tools help in behavioral analysis?
AI algorithms analyze user behavior, detect abnormalities and malicious activity in real time, facilitating immediate response and threat mitigation.
How can AI improve network security?
AI automates network segmentation, analyzes network traffic for potential threats, and detects and blocks malicious activity, enhancing overall cybersecurity defenses.
What is the future of AI in cybersecurity?
Continual advancements in AI technology are expected to strengthen threat detection, automate response capabilities, and provide enhanced protection against evolving cyber threats.
In an era where technology intersects with every aspect of life, Maxwell bridges the gap between artificial intelligence and journalism. As a writer and AI expert, he explores the implications of emerging technologies on society, economy, and culture. Maxwell’s expertise allows Press Report to offer forward-thinking insights into the future of AI, making complex topics accessible and engaging for our audience.
-
AI in Legal2 weeks ago
Revolutionizing Justice: How AI is Transforming the Legal Landscape
-
AI Entertainment1 month ago
OpenAI Releases SORA: Breakthrough AI Platform
-
AI in Business2 weeks ago
AI: The Powerhouse Driving Tomorrow’s Industries
-
AI in Legal2 weeks ago
From Briefs to Bytes: The Impact of AI on Legal Documentation
-
AI in Business2 weeks ago
Secure Your Future with AI Data Privacy Assurance
-
AI in Medicine2 weeks ago
Revolutionizing the Future: AI in Healthcare and Medicine
-
AI Security2 weeks ago
Unlock the Power of Robust AI Security for Your Business
-
AI in Business2 weeks ago
AI’s Effect on Employment: Trends & Insights