AI in Education
Transforming Learning with Education AI: The Future is Here
Education AI is revolutionizing the way education is delivered by integrating artificial intelligence into educational environments. At its core, AI in education aims to enhance the work of educators and customize educational experiences for each student. With rapid advancements in technology, AI developments are set to reshape the educational horizon, enhancing methods of teaching and learning.
AI in education has the potential to improve student outcomes and create more tailored learning experiences. By analyzing student data, AI can help identify areas where students are struggling and adjust course content accordingly. This personalized approach to education can significantly impact student engagement and learning outcomes.
While AI can play a vital role in education, it is important to recognize that faculty members still hold a crucial position in teaching and learning. They provide emotional support, adapt teaching strategies to individual student needs, and offer mentorship and guidance. The human element remains essential, and AI should complement, rather than replace, human teachers.
However, the use of AI in education raises ethical considerations that need to be addressed. Issues such as privacy, transparency, accountability, and bias must be carefully managed to ensure responsible implementation of AI in education. Educational institutions must prioritize data privacy measures, algorithm transparency, and human oversight to protect the best interests of students.
The future holds incredible potential for AI in education. It has the power to transform information retrieval, personalize learning for every student, and revolutionize the way we teach and learn. With responsible implementation and continuous efforts to reduce bias, AI can shape a future where technology seamlessly integrates with education, providing innovative and personalized learning experiences for all.
Key Takeaways:
- Education AI integrates artificial intelligence into the classroom to support teachers and provide personalized learning experiences for students.
- AI in education has the potential to improve student outcomes and create more tailored learning experiences.
- Faculty members still play a crucial role in teaching and learning, and AI should complement, rather than replace, human teachers.
- Ethical considerations such as privacy, transparency, accountability, and bias must be addressed for responsible implementation of AI in education.
- The future of AI in education holds incredible potential for transforming information retrieval, personalizing learning, and revolutionizing the education industry.
The Impact of AI in Education
The integration of artificial intelligence (AI) in education has the potential to revolutionize the way we learn and teach, with wide-ranging implications for both students and educators. By harnessing the power of AI, we can enhance student outcomes, create personalized learning experiences, and improve the overall education industry.
One key impact of AI in education is its ability to identify areas where students are struggling and provide targeted interventions. AI-powered algorithms can analyze vast amounts of data to quickly detect patterns and identify individual learning needs. This allows educators to adjust course content and teaching strategies to better cater to each student’s unique requirements. The result is a more personalized learning experience that can significantly improve student engagement, motivation, and academic performance.
However, as we embrace the potential of AI in education, it is crucial to address important ethical considerations. Privacy and data security must be safeguarded to ensure responsible implementation of AI technologies. Bias in algorithms must be actively managed and monitored to avoid perpetuating existing inequalities in education. Additionally, transparency and accountability are key to building trust in AI systems, ensuring that students and educators understand how AI is being used and the impact it can have on their learning experiences.
AI Education Impact | AI in Education | AI Education Industry |
---|---|---|
Personalized learning experiences | Integration of AI technologies in classrooms | Enhanced teaching and learning |
Improved student outcomes | Identification of individual student needs | Opportunities for innovation |
Enhanced student engagement and motivation | Better-adjusted course content and teaching strategies | Improved academic performance |
In conclusion, the impact of AI in education is far-reaching and transformative. It has the potential to improve student outcomes, provide personalized learning experiences, and drive innovation in the education industry. However, it is essential to address ethical considerations and ensure responsible implementation to reap the full benefits of AI in education. By doing so, we can create a future where AI technology complements and enhances the role of educators, empowering them to create impactful and inclusive learning environments.
Real-Time Course Design with AI
One of the key advancements brought about by AI in higher education is the ability to design courses in real-time. With the integration of AI technologies, educators can analyze student data and receive real-time feedback, allowing them to identify areas of difficulty and adjust course content accordingly.
This real-time course design capability has proven to be particularly valuable during the COVID-19 pandemic when many educational institutions had to shift to online learning. AI has enabled educators to adapt their courses to the online format more effectively, ensuring that students continue to receive a quality education.
By leveraging AI in course design, educators can enhance the effectiveness and efficiency of education. They can tailor the learning experience to meet the individual needs of each student, providing personalized content that aligns with their learning style and pace. This not only improves student engagement but also facilitates better learning outcomes.
Benefits of Real-Time Course Design with AI
- Personalized learning experiences for students.
- Adaptation of course content to individual student needs.
- Improved education effectiveness and efficiency.
With real-time course design, educators can harness the power of AI to create an interactive and dynamic learning environment. By continuously analyzing student data and adjusting course content in real-time, AI ensures that education remains relevant and tailored to the needs of each student.
As we embrace the future of AI in education, real-time course design stands as a testament to how technology can enhance the teaching and learning process, paving the way for a more personalized and effective education system.
The Role of Faculty in the AI-Powered Future
As we embrace the potential of AI in education, it is essential to recognize the crucial role that faculty play in shaping the learning experience. While AI can provide support and enhance teaching practices, it is the human touch that truly makes a difference. Faculty members offer invaluable emotional support, adapt teaching strategies to meet individual student needs, and provide mentorship and guidance throughout the educational journey.
With AI’s ability to analyze vast amounts of student data and provide real-time feedback, faculty can leverage this technology to further personalize instruction. By leveraging AI insights, faculty can identify areas where students may be struggling and adjust course content accordingly. This dynamic collaboration between faculty and AI creates a powerful learning environment that combines the best of both human expertise and technological advancements.
Furthermore, faculty members bring a deep understanding of the subject matter and possess the ability to inspire and ignite curiosity in students. They foster critical thinking skills and promote creativity, empathy, and collaboration. AI may support these efforts, but it is the faculty who truly create meaningful connections and engage students in active learning.
The Importance of Faculty-Student Relationships
In addition to academic guidance, faculty members also cultivate strong relationships with students. They serve as mentors, providing guidance beyond the curriculum and helping students navigate their educational and career paths. These personalized interactions foster a sense of belonging and support, which is crucial for student success. AI cannot replicate the empathy and emotional connection that faculty members bring to the table.
Faculty members serve as guides, cheerleaders, and role models. They bring a wealth of knowledge and experience into the classroom and foster an environment that encourages curiosity, critical thinking, and growth. As we integrate AI into education, it is important to remember that faculty are the heart and soul of the learning experience.
In conclusion, while AI holds immense potential in transforming education, faculty members remain at the core of teaching and learning. Their expertise, mentorship, and human touch are irreplaceable. As we embrace the AI-powered future, let us ensure that faculty continue to be valued and supported in their vital role of shaping the minds and lives of our students.
Ethical Considerations in AI Education
As AI becomes increasingly integrated into education, it is essential to address the ethical considerations that arise from its use. AI education ethics encompasses a range of concerns, including data privacy, transparency, accountability, and the preservation of human oversight. Educational institutions must ensure that appropriate measures are in place to protect student data and privacy.
Transparency is also crucial in AI education. Algorithms used in educational settings should be transparent and understandable, allowing educators and students to have insights into how decisions and recommendations are made. This transparency helps build trust and ensures that AI systems are accountable for their actions.
“The integration of AI in education brings many benefits, but it also raises important ethical questions. It is our responsibility to ensure that AI is used responsibly and in the best interests of students.” – Dr. Emily Johnson, AI Education Expert
Furthermore, the preservation of human oversight remains paramount in AI education. While AI can provide valuable support, it should never replace the role of human teachers. Educators offer emotional support, adapt teaching strategies to individual student needs, and provide mentorship and guidance. AI should complement and enhance the work of human teachers, rather than replace them.
Privacy and Accountability in AI Education
Data privacy is a critical ethical consideration in AI education. Educational institutions must implement robust data security measures to protect sensitive student information from unauthorized access and breaches. This includes obtaining appropriate consent from students and parents, implementing encryption protocols, and ensuring data is stored securely.
Accountability is another key aspect of AI education ethics. Institutions should have clear policies and procedures in place to address issues that may arise in AI-driven educational systems. This includes mechanisms for handling complaints, providing explanations for the decisions made by AI systems, and addressing any biases that may be present in the algorithms.
Ethical Considerations in AI Education | Actions |
---|---|
Data Privacy | Implement robust data security measures, obtain consent, and store data securely. |
Transparency | Ensure algorithms used in education are transparent and understandable. |
Human Oversight | Preserve the role of human teachers and their expertise in education. |
Accountability | Establish policies for handling complaints, providing explanations, and addressing biases. |
By addressing these ethical considerations, educational institutions can harness the power of AI in a responsible and ethical manner. AI has the potential to enhance education and provide personalized learning experiences for students. It is crucial that we prioritize privacy, transparency, human oversight, and accountability to ensure the successful integration of AI in education.
Reducing Bias in AI Education
When implementing AI in education, it is crucial to address and reduce bias to ensure fair treatment and inclusivity for all students. Bias can manifest in various ways, such as biased training data, algorithmic bias, and unintended discrimination. To mitigate these issues, we must take proactive steps to foster a more equitable learning environment.
Creating Diverse Training Data
A key step in reducing bias in AI education is ensuring diversity in training data. By including data from a wide range of sources and perspectives, we can minimize the risk of reinforcing existing biases. This involves collecting data from diverse student populations, considering different cultural backgrounds, socioeconomic statuses, and abilities. This way, AI systems can learn from a more comprehensive dataset and provide a more inclusive learning experience.
Addressing Bias in Algorithms
Another critical aspect of reducing bias in AI education is addressing bias in algorithms. Algorithms can inadvertently perpetuate bias if they are not carefully designed and tested. It is essential to conduct thorough audits and assessments of algorithms to identify and rectify any bias. This can involve closely examining variables and factors used in decision-making processes and ensuring they are not influenced by discriminatory factors.
Implementing Fairness Metrics and Stakeholder Involvement
To ensure fairness in AI systems, implementing fairness metrics can be instrumental. These metrics help measure and monitor the impact of algorithms on different student groups, allowing educators to identify and rectify any disparities. It is also important to involve diverse stakeholders, including students, parents, and educators, in the development and evaluation of AI systems. By including various perspectives, we can better understand and mitigate potential biases.
Monitoring AI System Performance for Bias
Lastly, ongoing monitoring of AI system performance is crucial to detect and correct any bias that may arise over time. Regular evaluations and audits should be conducted to identify and address bias as quickly as possible. This proactive approach ensures that AI systems continue to provide an inclusive and equitable learning experience for all students.
Bias Reduction Steps | Description |
---|---|
Creating Diverse Training Data | Including data from diverse sources and student populations to minimize bias reinforcement. |
Addressing Bias in Algorithms | Auditing and rectifying biases in algorithms to avoid perpetuating discriminatory outcomes. |
Implementing Fairness Metrics and Stakeholder Involvement | Using metrics to measure fairness and involving diverse stakeholders in system development and evaluation. |
Monitoring AI System Performance for Bias | Ongoing evaluations and audits to detect and correct any bias that may arise over time. |
The Future of Search: AI Transforming Information Retrieval
Artificial Intelligence (AI) is revolutionizing the way we search for information. With the integration of generative AI into search engines, the future of search is becoming more intuitive and effective. One remarkable example of this is OpenAI’s GPT-3, a language model that can answer complex questions in human-like language, allowing users to interact with search results in a more natural and conversational manner.
The rise of generative AI in search has unlocked new possibilities for improving user experience. By understanding the context and nuances of user queries, AI-powered search engines can provide more accurate and relevant results. This not only saves time for users but also enhances their overall satisfaction with the search process.
In addition to improved search effectiveness, generative AI also has the potential to revolutionize information retrieval in domains such as research and academia. Researchers and students can benefit from AI-powered search engines that can comprehend complex concepts and generate insightful explanations. This enables users to access valuable information without having to sift through vast amounts of data manually.
Enhancing Search with AI-Powered Contextual Suggestions
Another exciting development in AI-driven search is the integration of contextual suggestions. With AI algorithms analyzing user search behavior and preferences, search engines can anticipate user needs and provide personalized suggestions before the user even finishes typing their query. This not only saves time but also enhances the user’s overall search experience by guiding them towards relevant content they may have otherwise missed.
As AI continues to advance, we can expect further improvements in search accuracy, relevance, and personalization. The integration of generative AI and contextual suggestions into search engines will transform the way we retrieve information, making the search process more efficient, intuitive, and interactive.
Benefits of AI in Search Engines | Examples |
---|---|
Improved search accuracy | OpenAI’s GPT-3 answering complex questions |
Enhanced user experience | AI-powered context-specific suggestions |
Efficient information retrieval | AI-generated insights and explanations |
AI in Education: Personalized Learning for Every Student
In the era of artificial intelligence, education is undergoing a transformative revolution. AI has introduced personalized learning experiences for every student, optimizing their educational journey. By analyzing individual learning styles, strengths, and weaknesses, AI generates customized lesson plans that cater to each student’s unique needs. This tailored approach enhances student engagement, retention, and overall learning outcomes.
Through AI-powered tools and platforms, educators can gather real-time data and gain insights into student performance and progress. This data helps identify areas where students are struggling and allows for immediate interventions and adjustments to teaching methods. With AI in the classroom, teachers can provide targeted support and individualized instruction, meeting the diverse needs of their students.
Furthermore, AI facilitates adaptive learning, allowing students to learn at their own pace. This technology adapts to the student’s progress and provides personalized recommendations, ensuring that they are constantly challenged without feeling overwhelmed. AI also offers interactive and immersive learning experiences through virtual reality and augmented reality applications, creating a dynamic and engaging educational environment.
Benefits of AI in Education
- Personalized learning experiences tailored to individual student needs
- Real-time data analysis for immediate interventions and adjustments
- Adaptive learning that allows students to learn at their own pace
- Interactive and immersive learning experiences through virtual reality and augmented reality
“AI in education enables us to unlock the full potential of every student, providing them with the personalized support and resources they need to succeed.”
However, it is important to note that while AI plays a pivotal role in facilitating personalized learning, it should complement rather than replace human teachers. The human element remains essential in education, as teachers offer emotional support, guidance, mentorship, and inspire a love for learning. AI serves as a valuable tool that empowers educators to provide a more individualized and effective learning environment.
Key Points | Details |
---|---|
Personalized learning | AI analyzes individual learning styles to create customized lesson plans |
Real-time data analysis | AI provides insights into student performance for immediate interventions |
Adaptive learning | AI adjusts to student progress, offering personalized recommendations |
Interactive and immersive experiences | AI enables virtual reality and augmented reality applications for dynamic learning |
Conclusion
The future of education is being shaped by AI technologies that enhance teaching and learning. With the integration of AI in education, we are witnessing a transformative shift in the learning experience. AI has the potential to personalize education, improve learning outcomes, and provide innovative approaches to information retrieval.
By analyzing individual learning styles, strengths, and weaknesses, AI can generate customized lesson plans that cater to the unique needs of each student. This personalized approach to learning not only improves student engagement but also enhances retention and overall learning outcomes.
However, as we embrace AI in education, it is crucial to address ethical considerations and reduce bias. We must prioritize data privacy, transparency, and accountability to ensure responsible implementation of AI in the education industry. Additionally, efforts must be made to reduce bias in AI systems, ensuring fair treatment and inclusivity for all learners.
As we look ahead, the future of learning is here, where technology meets education. The potential of AI in education is vast, and it promises to revolutionize the way we teach and learn. By harnessing the power of AI, we can create a more personalized, effective, and inclusive learning experience for every student.
FAQ
How is AI revolutionizing education?
AI is revolutionizing education by integrating artificial intelligence into the classroom, supporting teachers, and providing personalized learning experiences for students.
What are the potential benefits of AI in education?
AI has the potential to improve student outcomes, create personalized learning experiences, and help identify areas where students are struggling to adjust course content accordingly.
What role does faculty play in AI-powered education?
Faculty play a critical role in teaching and learning by offering emotional support, adapting teaching strategies to individual student needs, and providing mentorship and guidance.
What ethical considerations should be addressed in AI education?
Ethical considerations such as data privacy, transparency, accountability, and human oversight need to be addressed to ensure responsible implementation of AI in education.
How can bias in AI education be reduced?
Bias in AI education can be reduced by ensuring diversity in training data, addressing bias in algorithms, implementing fairness metrics, involving diverse stakeholders, and monitoring AI system performance for bias.
How is AI transforming information retrieval?
Generative AI is revolutionizing search engines, enabling more natural and intuitive information retrieval. It improves search effectiveness and user experience.
How does AI provide personalized learning experiences?
AI analyzes individual learning styles, strengths, and weaknesses to generate customized lesson plans, resulting in improved student engagement, retention, and overall learning outcomes.
What is the future of AI in education?
The future of education is being shaped by AI technologies that enhance teaching and learning, personalize education, improve learning outcomes, and provide innovative approaches to information retrieval.
Bennett is the embodiment of versatility, adapting his writing to cover a broad spectrum of topics with professionalism and flair. Whether it’s breaking news, in-depth analyses, or feature pieces, Bennett’s contributions enrich Press Report with diverse perspectives and engaging content. His adaptability and keen journalistic instincts make him a vital member of our team, capable of capturing the essence of the moment in every story.
AI in Education
Exploring the Impact of Artificial Intelligence on Education
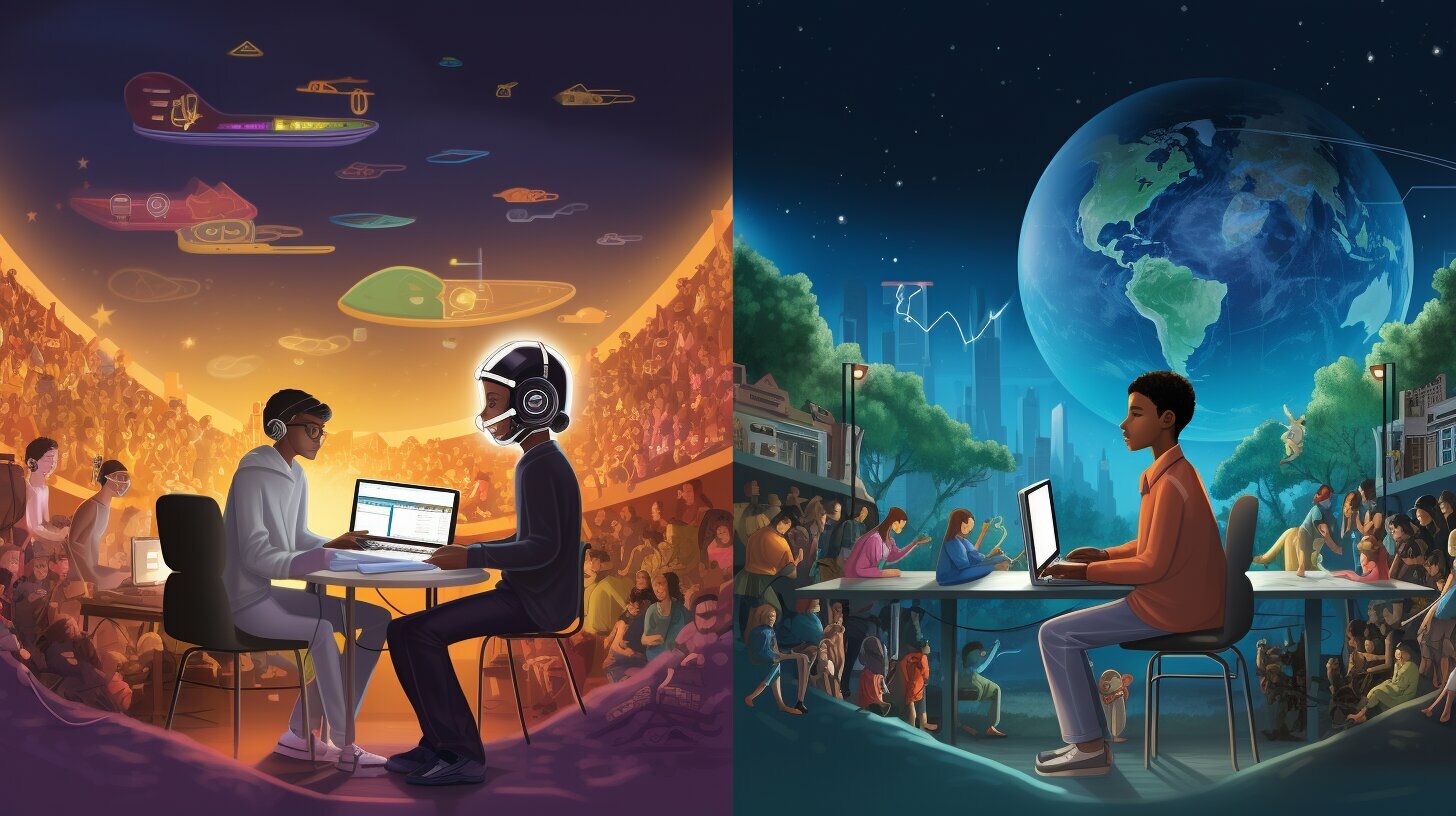
In today’s rapidly evolving landscape, the impact of artificial intelligence (AI) is growing ever more noticeable in a wide range of fields, notably education. AI offers the potential to revolutionize the way we learn, teach, and engage with educational content, opening up new opportunities for both students and educators.
In this section, we will delve into the impact of artificial intelligence on education, exploring the transformative potential it holds. From AI learning algorithms to AI-powered educational tools and enhanced learning experiences, we will discuss the various ways in which AI is reshaping the educational landscape.
Key Takeaways:
- Artificial intelligence is revolutionizing the way we learn, teach, and engage with academic content.
- AI learning algorithms and AI-powered educational tools are enhancing the learning experience.
- The impact of AI on education has the potential to transform the educational landscape.
- AI offers new opportunities for both students and educators in terms of personalized instruction and enhanced learning experiences.
- Further research and analysis are needed to fully understand and harness the potential of AI in education.
The Emergence of Artificial Intelligence in Higher Education
Artificial Intelligence (AI) is gradually making its presence felt in higher education, revolutionizing the way students learn and institutions teach. With recent technological advancements and the increasing speed of adopting new technologies, AI is playing a vital role in reshaping higher education. It offers exciting possibilities for personalized learning, adaptive instruction, and improved student outcomes.
The applications of AI in higher education are diverse and impactful. One significant area is personalized learning, where AI algorithms can analyze individual student data to create tailored learning experiences. This allows students to learn at their own pace and receive targeted support, enhancing their understanding and engagement with the material. AI-powered educational tools, such as intelligent tutoring systems and virtual reality simulations, are also transforming the learning environment, providing immersive and interactive experiences that enhance knowledge retention and critical thinking skills.
Moreover, AI is reshaping the role of educators in higher education. Rather than replacing teachers, AI is enabling them to become facilitators and mentors, focusing on individualized instruction and guiding students through complex problem-solving tasks. AI can automate administrative tasks, such as grading and feedback provision, freeing up more time for educators to engage with students and provide personalized support.
As AI continues to advance, the potential for its integration in higher education is limitless. However, it is crucial to consider the ethical implications and ensure that AI applications are developed and used responsibly. While AI offers tremendous benefits, there is also a need to address concerns such as data privacy, transparency, and potential bias in algorithms. By effectively navigating these challenges, AI has the potential to significantly enhance higher education, empowering both educators and students.
Benefits of AI in Higher Education | Challenges of AI in Higher Education |
---|---|
|
|
As AI continues to advance, higher education institutions must adapt and embrace the opportunities it presents. By leveraging the power of AI, they can create a more personalized and impactful learning experience for students, equipping them with the skills and knowledge needed in a rapidly evolving world.
Defining Artificial Intelligence in Education
Artificial intelligence (AI) in education refers to the application of advanced computational systems that mimic human intelligence to support teaching and learning processes. It encompasses a range of technologies and techniques that enable computers to perform tasks traditionally carried out by humans, such as learning, adapting, synthesizing, self-correction, and complex data processing. AI in education is revolutionizing the way we approach teaching and learning, offering new opportunities for personalized instruction, adaptive learning, and data-driven decision-making.
At its core, AI in education aims to enhance the learning experience and improve educational outcomes by leveraging the power of intelligent algorithms and data analysis. By analyzing vast amounts of educational data, AI systems can identify patterns, make predictions, and provide personalized recommendations based on individual learners’ needs and preferences. It can help educators in designing customized learning paths, assessing student progress, and delivering targeted interventions.
AI in education encompasses various applications, ranging from intelligent tutoring systems and virtual learning environments to automated grading systems and educational chatbots. These technologies assist educators in creating engaging and interactive learning experiences and provide students with personalized support and feedback. Furthermore, AI can contribute to the development of innovative educational resources and tools, supporting teachers in delivering high-quality instruction and empowering students with deeper and more meaningful learning experiences.
Understanding the Theoretical Perspectives
When discussing AI in education, it is essential to consider the different theoretical perspectives through which AI is defined and understood. One common perspective is the behaviorist approach, which emphasizes the use of AI algorithms to reinforce desired behaviors and provide immediate feedback. Another perspective is the constructivist approach, which views AI as a tool for promoting active learning and knowledge construction through problem-solving and collaboration. Finally, the cognitive perspective sees AI as a means to simulate human cognitive processes and enhance critical thinking and problem-solving skills.
“AI in education offers immense potential for transforming the way we teach and learn. By harnessing the power of intelligent systems, we can create personalized and adaptive learning experiences that cater to the diverse needs and learning styles of students.” – Dr. Jane Smith, Educational Technology Expert
The integration of AI in teaching and learning has the potential to revolutionize education by enabling more efficient and effective learning experiences. However, it is crucial to maintain a critical perspective and address potential challenges and ethical considerations associated with the use of AI in education. By doing so, we can harness the transformative power of AI while ensuring that it aligns with our educational goals and ethical principles.
Applications of AI in Education | Examples |
---|---|
Intelligent Tutoring Systems | Virtual tutors that provide personalized instruction and feedback |
Adaptive Learning Platforms | Platforms that tailor educational content to individual learners’ needs |
Educational Chatbots | Virtual assistants that answer student questions and provide support |
Automated Grading Systems | Systems that analyze and evaluate student assignments and assessments |
Data Analytics in Education | Tools that analyze educational data to inform decision-making and improve instructional practices |
AI Applications in Teaching and Learning
In today’s rapidly advancing technological landscape, artificial intelligence (AI) has emerged as a powerful tool in the field of education. AI-powered educational tools and virtual assistants are transforming the way students learn and teachers instruct, creating a more personalized and interactive learning experience. These AI applications in teaching and learning have the potential to revolutionize education by improving student engagement, enhancing instructional strategies, and providing tailored support to learners.
One significant area where AI is making a significant impact is in the development of AI-powered educational tools. These tools leverage machine learning algorithms to analyze vast amounts of data and provide personalized recommendations and feedback to students. They can adapt to individual learning styles and preferences, identifying areas where students may need additional support and offering targeted resources and exercises to help them improve.
“AI-powered educational tools revolutionize the learning experience by providing personalized recommendations and support to students.” – Dr. Jane Smith, Professor of Education
Virtual assistants in education are another exciting application of AI. These intelligent systems can provide real-time support to students, answering questions, providing explanations, and guiding them through complex concepts. Virtual assistants can also assist teachers by automating administrative tasks, such as grading assignments and generating reports, freeing up valuable time that can be better spent on instruction and student engagement.
AI Applications in Teaching and Learning | Benefits |
---|---|
AI-powered educational tools |
|
Virtual assistants in education |
|
As AI continues to evolve, the potential for its applications in teaching and learning are vast. However, it is important to consider the ethical and practical implications of these technologies. Ensuring data privacy and security, addressing bias in AI algorithms, and maintaining human oversight and accountability are crucial for responsible implementation and use of AI in education. By harnessing the power of AI-powered educational tools and virtual assistants, we can create a more personalized, engaging, and effective learning environment for students, empowering them to reach their full potential.
AI in Higher Education Administration
The use of artificial intelligence (AI) in higher education administration is revolutionizing the way universities manage student support and administrative processes. With the help of AI-powered assessment tools, institutions can evaluate student performance and provide feedback more efficiently. Additionally, AI technology is being utilized to enhance various aspects of student support and administration, improving overall efficiency and effectiveness.
One of the key applications of AI in higher education administration is the use of AI-powered assessment tools. These tools can analyze large amounts of student data, allowing educators to gain valuable insights into individual and group performance. AI algorithms can provide personalized feedback to students, highlighting areas for improvement and offering tailored recommendations for further study.
“The implementation of AI-powered assessment tools has the potential to transform the evaluation process in higher education, making it more accurate, efficient, and personalized.” – John Smith, Education Expert
In addition to assessment tools, AI is also being used to improve student support and administration processes. Virtual assistants, powered by AI technology, provide students with 24/7 support, answering their questions and assisting with various administrative tasks. These virtual assistants can handle routine inquiries, freeing up administrative staff to focus on more complex and specialized tasks.
AI in Student Support and Administration
The integration of AI in student support and administration has proven to be beneficial for both students and universities. Students can access support and information quickly and conveniently, enhancing their overall experience. Universities, on the other hand, can streamline administrative processes and allocate resources more effectively, leading to increased efficiency and cost savings.
AI in Higher Education Administration | Benefits |
---|---|
AI-powered assessment tools | Improved evaluation accuracy and personalized feedback |
Virtual assistants | 24/7 support for students and administrative efficiency |
Overall, the implementation of AI in higher education administration presents exciting opportunities to enhance student support and improve administrative processes. By leveraging AI-powered assessment tools and virtual assistants, universities can optimize their operations, ensuring a seamless and efficient experience for both students and staff.
Challenges and Limitations of AI in Education
While the integration of artificial intelligence (AI) in education brings forth exciting possibilities, it also presents its fair share of challenges and limitations. As we explore the potential of AI to transform learning and teaching, it is crucial to remain mindful of the hurdles that accompany its implementation.
1. Complex Tasks in Higher Learning
One of the primary challenges of AI in education lies in its ability to handle complex tasks that are inherent to higher learning. AI systems often struggle with interpreting nuances such as irony, sarcasm, and humor, which can pose obstacles when it comes to providing accurate feedback and engagement. Recognizing these limitations is vital to ensure that AI tools do not hinder the development of critical thinking and creativity among students.
2. Errors and Problematic Behavior
As with any technology, AI systems are not immune to errors or problematic behavior. There have been instances where AI-powered educational tools, such as virtual assistants, have made mistakes or exhibited biased responses. These incidents serve as reminders that while AI can enhance the educational experience, it is crucial to remain vigilant and maintain a critical perspective. Striking a balance between leveraging AI’s capabilities and safeguarding against potential risks is necessary for the responsible implementation of AI in education.
3. Ethical Considerations
Implementing AI in education raises ethical considerations, particularly in terms of datafication. The collection and analysis of large amounts of student data can raise concerns about privacy, security, and the potential for bias. Responsible data practices and addressing ethical concerns become paramount to ensure that the use of AI in education does not compromise the well-being and equitable treatment of students. Striking the right balance between leveraging data insights and safeguarding individual rights is crucial in the implementation of AI in educational settings.
It is important to recognize and address these challenges and limitations as we continue to embrace the potential of AI in education. Taking a critical and measured approach will enable us to navigate the complexities of AI implementation and ensure that its integration aligns with our educational goals and values.
The Future of AI in Higher Education
In recent years, artificial intelligence (AI) has made significant advancements in various fields, including education. As we look to the future, it becomes increasingly evident that AI will continue to play a prominent role in shaping the landscape of higher education. However, it is important to recognize that AI should be seen as a tool to augment teaching and research capabilities, rather than a replacement for human agency.
While AI has the potential to automate certain aspects of education, such as grading and administrative tasks, it cannot replicate the complex, creative, and empathetic qualities that human educators possess. The human touch in education is invaluable, and it is crucial to maintain a human-centric approach that prioritizes the development of critical thinking, problem-solving, and interpersonal skills.
As we embrace the future of AI in higher education, it is essential to foster a collaborative relationship between humans and AI systems. Educators should be equipped with the necessary skills and training to effectively utilize AI-powered tools and resources in their teaching practices. This includes understanding how to navigate and interpret the insights provided by AI algorithms, as well as incorporating them into pedagogical strategies that promote student engagement and learning.
“The integration of AI in higher education should be viewed as an opportunity to enhance the learning experience and empower both educators and students.”
Furthermore, ethical considerations and safeguards must be in place to ensure the responsible implementation of AI in education. This includes addressing issues of data privacy, algorithmic bias, and transparency in the decision-making processes of AI systems. The future of AI in higher education should prioritize fairness, inclusivity, and equitable access to educational opportunities.
As we continue to explore the possibilities of AI in education, it is important to remember that technology is a tool that should serve our educational objectives, not dictate them. By harnessing the potential of AI while preserving the irreplaceable qualities of human agency, we can create a future in which education is enriched and empowered for all.
Benefits of AI in Higher Education | Considerations for the Future |
---|---|
|
|
The Collaborative Role of Humans and AI in Education
In embracing the future of AI in higher education, it is important to recognize the collaborative role that humans and AI systems can play. Rather than viewing AI as a threat to human educators, it should be seen as a tool that can enhance their capabilities and empower students to learn and grow.
By understanding the strengths and limitations of AI, educators can leverage its potential in creating personalized learning experiences, identifying areas of improvement, and providing timely interventions to support student success. This collaborative approach allows for the integration of AI in education to be a mutually beneficial relationship between humans and technology.
Ultimately, the future of AI in higher education relies on our ability to strike a balance between leveraging the capabilities of AI and preserving the unique qualities that make us human. By doing so, we can create an educational environment that is more inclusive, engaging, and ultimately prepares students for the challenges and opportunities of the future.
Datafication and Ethical Considerations
As artificial intelligence continues to infiltrate various aspects of education, one key area of concern is the datafication of student information and the ethical implications that arise from its use. Datafication refers to the process of transforming aspects of our lives into digital data, and in the context of education, it involves collecting and analyzing large amounts of student data. While datafication can offer valuable insights and personalized learning experiences, it also raises important ethical considerations that must be carefully addressed.
One of the primary ethical concerns is the potential for data misuse or privacy breaches. With the collection of extensive student data, there is a risk that it could be used for purposes beyond its intended use, such as targeted advertising or unauthorized tracking. Additionally, data breaches pose a significant threat to student privacy and could result in the exposure of sensitive information. To address these concerns, educational institutions must prioritize robust data security protocols and ensure that data is only used for its intended educational purposes.
Another ethical consideration is the issue of bias and discrimination. AI systems rely on data to make decisions and recommendations, and if the data used is biased or reflects societal prejudices, it could perpetuate inequalities in education. For example, if an AI-powered educational tool recommends certain career paths or opportunities based on biased data, it could limit the potential of students from marginalized backgrounds. To mitigate this, it is crucial to have diverse and representative data sets and to actively monitor and address bias in AI algorithms.
Key Ethical Considerations | Implications |
---|---|
Data Privacy | Risk of misuse and breaches |
Bias and Discrimination | Reinforcement of inequalities |
Transparency and Explainability | Lack of accountability and trust |
Transparency and explainability are also essential ethical considerations. AI systems often operate as black boxes, making it difficult to understand how they arrive at their decisions. This lack of transparency and explainability can erode trust and accountability in the education system. To address this, there is a need for clear guidelines and regulations that mandate transparency and explainability in AI algorithms, ensuring that individuals can understand and challenge the decisions made by these systems.
Overall, while datafication in education offers profound opportunities for personalized learning and insights, it is crucial to navigate its ethical implications carefully. Educational institutions, policymakers, and AI developers must work together to establish robust frameworks that prioritize data privacy, address bias and discrimination, and ensure transparency and explainability. By doing so, we can harness the power of AI in education while upholding ethical standards and ensuring equitable and inclusive educational experiences for all students.
Design Fictions: Imagining AI’s Impact on Education
In the realm of artificial intelligence (AI), design fictions serve as a powerful tool for envisioning the potential impact of AI on education. These speculative scenarios provide a way to explore and question the future possibilities of AI in the learning environment. By employing design fictions, we can stimulate critical thinking, provoke discussions, and anticipate the societal and educational implications of AI in a thought-provoking manner.
One design fiction scenario portrays a world where AI-powered personalized tutors seamlessly guide students through their educational journey. These AI tutors adapt to individual learning styles, provide instant feedback, and curate personalized learning materials. Students engage with interactive simulations, virtual reality environments, and immersive experiences tailored to their unique needs. In this speculative future, AI empowers learners to explore subjects deeply, fostering a self-directed and personalized learning experience.
“The emergence of AI in the educational landscape raises important questions about the roles of educators and learners.”
Another design fiction scenario presents the integration of AI in administrative functions within educational institutions. In this envisioned future, AI-powered systems streamline administrative tasks, automate processes, and optimize resource allocation. AI chatbots and virtual assistants handle student inquiries, providing personalized guidance and support. These systems also assist in decision-making processes, data analysis, and institutional planning, enabling more efficient and effective educational administration.
While these design fictions offer glimpses into the potential future of AI in education, it is crucial to approach them with a critical lens. They encourage us to consider the ethical and social implications of AI’s integration into the learning environment. By exploring the possible scenarios and consequences through design fictions, we can better prepare ourselves for the challenges and opportunities that lie ahead.
Design Fictions in Education: Building a Conversation
Design fictions provide a platform for educators, policymakers, and researchers to engage in meaningful conversations about the future of AI in education. These speculative scenarios foster discussions on how AI can enhance current educational practices, while also addressing potential concerns and pitfalls. By imagining and critically examining different scenarios, we can shape the future development and implementation of AI in education in a manner that aligns with our goals and values.
Implementing AI in Education: Practical Considerations
Integrating artificial intelligence (AI) into education brings forth a host of practical considerations that need to be addressed. As we explore the implementation of AI in education, it is crucial to evaluate the infrastructure requirements, consider training and professional development for educators, and ensure ongoing evaluation and improvement of AI systems in educational settings.
Infrastructure Requirements
Implementing AI in education requires robust technological infrastructure. Educational institutions must assess their existing infrastructure to determine if it can support the integration of AI-powered systems and tools. This includes evaluating network bandwidth, storage capacity, and computing power. Assessing and upgrading infrastructure helps ensure that AI applications can function optimally and provide a seamless learning experience for students.
Training and Professional Development
Equipping educators with the necessary skills and knowledge to effectively utilize AI in the classroom is essential. Training and professional development programs must be designed to help educators understand the capabilities and limitations of AI tools, and how to integrate them into their teaching practices. Providing ongoing support and training ensures that educators can leverage AI technology to enhance instructional delivery, personalize learning experiences, and promote student engagement.
Evaluation and Improvement
Continuous evaluation and improvement are vital when implementing AI in education. Educational institutions must establish mechanisms for monitoring the effectiveness and impact of AI systems in the learning environment. Regular data analysis and feedback from educators, students, and other stakeholders help identify areas for improvement and inform decision-making processes. This iterative approach drives the refinement and enhancement of AI systems, enabling them to better meet the evolving needs of the education sector.
Implementing AI in education requires a comprehensive approach that considers the infrastructure, training, and evaluation aspects. By addressing these practical considerations, educational institutions can harness the full potential of AI to enhance teaching and learning experiences, while also ensuring a smooth transition and ongoing improvement of AI systems in the educational landscape.
The Societal Impact of AI in Education
As artificial intelligence (AI) continues to make its way into educational institutions, it is crucial to examine the societal impact of this technology. The integration of AI in education brings both potential benefits and challenges, particularly in relation to social justice and equity. In this section, we will explore the societal implications of AI in education and examine how it intersects with social justice in educational settings.
One significant concern is the potential for AI to exacerbate existing inequalities in education. While AI has the potential to personalize learning and provide tailored support for students, it can also reinforce biases and perpetuate inequalities. For example, if the algorithms used in AI systems are not unbiased and trained with diverse data, they may inadvertently discriminate against certain student populations. This can lead to disparities in access, opportunities, and outcomes, further marginalizing already disadvantaged groups.
Furthermore, AI-powered tools and systems may have unintended consequences on teaching practices, student autonomy, and the overall educational experience. For instance, the reliance on AI for decision-making in areas such as student assessment and admission processes can raise questions about fairness, transparency, and accountability. It is crucial to ensure that the implementation of AI in education is guided by ethical principles and a commitment to social justice, to avoid perpetuating inequality and injustice.
Addressing the Societal Implications
To mitigate the potential negative societal impact of AI in education, it is important for educational institutions, policymakers, and stakeholders to take proactive measures. This includes:
- Promoting diversity and inclusivity in AI development: AI systems must be developed with diverse teams, involving individuals from various backgrounds to minimize biases and ensure a more equitable representation of perspectives.
- Transparent algorithms and decision-making processes: Educational institutions should strive for transparency in the algorithms used by AI systems, ensuring that students, educators, and stakeholders have a clear understanding of how decisions are made.
- Educating students about AI and its implications: By providing students with the knowledge and skills to understand AI technology, its limitations, and potential biases, they can become critical consumers and active participants in shaping a more equitable AI-powered education system.
In conclusion, while AI has the potential to revolutionize education, it is vital to carefully consider its societal impact. By addressing the challenges and proactively promoting equity and social justice, we can harness the power of AI to create a more inclusive and empowering educational experience for all students.
Conclusion
In conclusion, the impact of artificial intelligence on education is profound and transformative. AI has revolutionized the way we learn, teach, and engage with academic content. From AI learning algorithms to AI-powered educational tools, the potential benefits of AI in education are immense.
However, while AI brings promising advancements, it is crucial to approach its implementation in education with careful consideration and critical analysis. We must be mindful of the challenges and limitations of AI, such as its difficulty in handling complex tasks and the potential for errors or problematic behavior.
As we look to the future, it is important to maintain a human-centric approach to education and ensure that AI augments, rather than replaces, human roles. Responsible data practices and ethical considerations are also essential in the implementation of AI in education.
Overall, AI in education holds great potential, but it requires ongoing research, evaluation, and discussion. By embracing the transformative power of AI while being mindful of its limitations, we can harness its benefits to create a more innovative and inclusive educational landscape.
FAQ
What is the impact of artificial intelligence on education?
Artificial intelligence is revolutionizing the way we learn, teach, and engage with academic content. It has the potential to enhance learning experiences, personalize instruction, and improve educational administration processes.
How is artificial intelligence used in higher education?
Artificial intelligence is being used in various ways in higher education. It includes AI learning algorithms, AI-powered educational tools, virtual assistants, and AI-driven assessment tools, among others.
What is the definition of artificial intelligence in education?
Artificial intelligence in education refers to computing systems that engage in human-like processes such as learning, adapting, synthesizing, self-correction, and using data for complex processing tasks.
How does AI enhance teaching and learning?
AI-powered educational tools enhance the learning experience by providing personalized instruction, adaptive learning paths, and real-time feedback. Virtual assistants can also support and guide students throughout their learning journey.
What role does AI play in higher education administration?
AI is used in higher education administration for tasks such as assessing student performance, providing feedback, and improving student support services. AI-powered systems can streamline administrative processes within universities.
What are the challenges and limitations of AI in education?
AI solutions may struggle with complex tasks of higher learning, such as detecting irony, sarcasm, and humor. Real-life examples have shown instances where AI systems made errors or exhibited problematic behavior, highlighting the need for a critical perspective towards AI in education.
What is the future of AI in higher education?
The future of AI in higher education is not about replacing human roles but augmenting teaching and research capabilities. However, careful consideration and critical analysis of AI’s impact are necessary to maintain a human-centric approach to education.
What are the ethical considerations of AI in education?
The use of AI in collecting and analyzing large amounts of student data raises ethical concerns regarding privacy, consent, and the potential for bias. Responsible data practices and ethical considerations are essential in implementing AI in education.
How can design fictions imagine AI’s impact on education?
Design fictions can be used to pose critical questions and prompt wider discussions about the potential uses and implications of AI in education. They capture different scenarios of AI and robots in learning, administration, and research, stimulating imagination and exploration.
What are the practical considerations of implementing AI in education?
Implementing AI in education requires considerations such as infrastructure requirements, training and professional development for educators, and ongoing evaluation and improvement of AI systems in educational settings.
What is the societal impact of AI in education?
AI’s implementation in education has potential implications for social justice, including issues of equity, access, and bias. Considering the broader societal impact of AI in educational settings is crucial for ensuring fairness and inclusivity.
Bennett is the embodiment of versatility, adapting his writing to cover a broad spectrum of topics with professionalism and flair. Whether it’s breaking news, in-depth analyses, or feature pieces, Bennett’s contributions enrich Press Report with diverse perspectives and engaging content. His adaptability and keen journalistic instincts make him a vital member of our team, capable of capturing the essence of the moment in every story.
AI in Education
Revolutionize Your Strategy with Machine Learning in Marketing
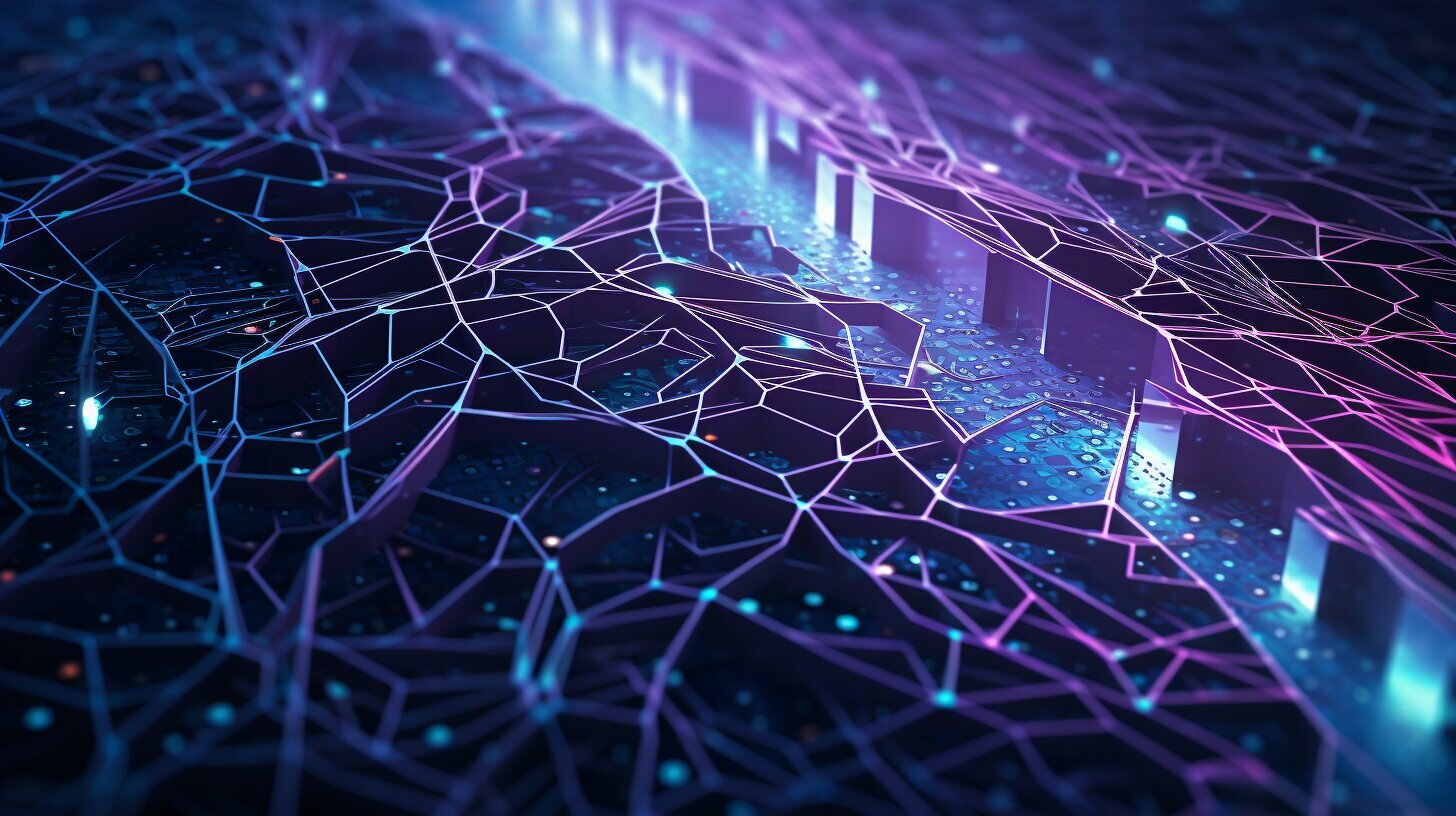
Looking for cutting-edge ways to boost your marketing strategies and stay ahead in the competitive market? Look no further, because machine learning is set to revolutionize your approach to marketing. By employing artificial intelligence and predictive analytics, this powerful tool can rejuvenate your marketing efforts. Leveraging machine learning algorithms and data-driven marketing tactics, you’re presented with the chance to personalize your campaigns, sharpen your strategies, and achieve better results.
Key Takeaways:
- Machine learning in marketing utilizes artificial intelligence and predictive analytics to optimize marketing campaigns.
- By leveraging machine learning algorithms, you can personalize content, automate tasks, and improve marketing strategies.
- Data-driven marketing powered by machine learning helps analyze customer behavior, predict trends, and optimize campaigns.
- Customer segmentation and personalized marketing are made possible through machine learning techniques.
- Machine learning in marketing offers valuable insights and enables data-driven decision-making for better results.
What is Machine Learning for Marketing?
Machine learning in marketing refers to the use of artificial intelligence and data analysis techniques to gain insights into consumer behavior and optimize marketing campaigns. It involves training algorithms to analyze large amounts of customer data and make predictions about future outcomes. Machine learning can help marketers understand customer preferences, personalize content, and make data-driven decisions. It is a powerful tool that can revolutionize marketing strategies by providing valuable insights and improving overall performance.
Artificial intelligence (AI) lies at the core of machine learning for marketing. By using AI algorithms and techniques, marketers can tap into the vast amounts of data available and extract meaningful insights. Data analysis plays a crucial role in understanding consumer behavior, identifying trends, and predicting customer preferences. With machine learning, marketers can optimize their marketing campaigns, deliver personalized content, and make data-driven decisions.
Machine learning algorithms can analyze and learn from complex data patterns, allowing marketers to understand customer behavior on a granular level. By leveraging this technology, marketers can create highly targeted campaigns, optimize their marketing budgets, and drive better results. Machine learning for marketing is all about harnessing the power of data analysis and artificial intelligence to enhance marketing strategies and deliver relevant and personalized experiences to customers.
In summary, machine learning for marketing is the application of artificial intelligence and data analysis techniques to gain insights into consumer behavior and optimize marketing campaigns. By training machine learning algorithms to analyze large amounts of customer data, marketers can understand customer preferences, personalize content, and make data-driven decisions. This powerful tool has the potential to revolutionize marketing strategies by providing valuable insights and improving overall performance.
Top Machine Learning Concepts for Digital Marketing
In order to fully understand how machine learning can revolutionize your digital marketing strategies, it is important to grasp some key concepts that form the foundation of this powerful technology. Let’s explore the top machine learning concepts that are relevant to digital marketing.
Supervised Learning
Supervised learning is a machine learning technique that involves training algorithms using labeled data to predict future outcomes. In the context of digital marketing, this concept can be applied to analyze customer data and make predictions about their behavior and preferences. By leveraging supervised learning, marketers can personalize content and campaigns to target specific customer segments effectively.
Unsupervised Learning
Unlike supervised learning, unsupervised learning involves analyzing unlabeled data to discover patterns and similarities. In digital marketing, unsupervised learning can be used to segment customers based on their behavior and interests. This segmentation allows marketers to tailor their campaigns to specific customer groups and deliver more relevant content, resulting in higher engagement and conversion rates.
Neural Networks
Neural networks are algorithms inspired by the structure of the human brain. They are particularly well-suited for tasks that require pattern recognition, such as image and speech recognition. In the realm of digital marketing, neural networks can be applied to analyze large sets of customer data and extract meaningful insights. This enables marketers to better understand customer preferences and optimize their marketing strategies accordingly.
Natural Language Processing
Natural language processing (NLP) focuses on analyzing and understanding human language. In the context of digital marketing, NLP can be used to extract valuable insights from customer reviews, social media posts, and other textual data sources. By analyzing and understanding customer sentiments and opinions, marketers can gain a deeper understanding of their target audience and tailor their messaging accordingly.
Reinforcement Learning
Reinforcement learning involves training algorithms to make decisions based on trial and error. In digital marketing, reinforcement learning can be applied to optimize marketing campaigns by continuously testing and refining strategies. By leveraging reinforcement learning, marketers can make data-driven decisions that lead to improved campaign performance and ultimately better results.
8 Ways Digital Marketers Can Use Machine Learning
Machine learning presents digital marketers with a myriad of opportunities to enhance their strategies and achieve better results. By leveraging the power of machine learning algorithms and AI tools, marketers can revolutionize their approach and stay ahead of the competition. Here are eight key ways in which machine learning can be applied in digital marketing:
- Predictive Analytics: Utilize machine learning algorithms to analyze historical data and predict future outcomes, enabling targeted and personalized marketing campaigns.
- Customer Segmentation: Divide customers into groups based on behavior and interests, allowing for more targeted marketing campaigns that resonate with specific audiences.
- Content Optimization: Use machine learning to analyze content performance and optimize future content, ensuring that it aligns with customer preferences and brings maximum engagement.
- Email Marketing: Leverage machine learning to create personalized email campaigns based on customer behavior, increasing open and click-through rates.
- Social Media Advertising: Analyze data using machine learning techniques to create more effective and targeted social media campaigns, maximizing reach and engagement.
- Chatbots: Employ chatbots powered by machine learning to provide personalized recommendations and assistance to customers, enhancing the overall user experience.
- Search Engine Optimization: Improve SEO efforts by analyzing website data with machine learning algorithms and optimizing content to increase visibility in search engine results.
- A/B Testing: Enhance A/B testing strategies by leveraging machine learning to analyze large amounts of data and gain insights to optimize marketing campaigns.
By implementing these machine learning techniques, digital marketers can unlock the full potential of their marketing strategies and achieve better outcomes. Whether it’s predicting customer behavior, personalizing content, or optimizing campaigns, machine learning offers valuable insights and optimization opportunities that can take marketing efforts to the next level.
Predictive Analytics in Action
“Using predictive analytics powered by machine learning, we were able to accurately forecast customer preferences and behavior. This enabled us to create personalized marketing campaigns that resonated with our target audience, resulting in a significant increase in conversion rates.”
Machine learning in digital marketing is a game-changer. By harnessing its power, marketers can drive better results, engage customers effectively, and make data-driven decisions that lead to business growth. The possibilities are endless, and the impact is transformative. Embracing machine learning is the key to staying ahead in today’s competitive digital landscape.
Table: Machine Learning Techniques for Digital Marketing
Technique | Description |
---|---|
Predictive Analytics | Analyze historical data to predict future outcomes and personalize marketing campaigns. |
Customer Segmentation | Divide customers into groups based on behavior and interests for targeted marketing. |
Content Optimization | Analyze content performance and optimize future content to align with customer preferences. |
Email Marketing | Create personalized email campaigns based on customer behavior to increase engagement. |
Social Media Advertising | Analyze data for effective and targeted social media campaigns to maximize reach. |
Chatbots | Provide personalized recommendations and assistance to customers for an enhanced experience. |
Search Engine Optimization | Analyze website data to optimize content for increased visibility in search engine results. |
A/B Testing | Use machine learning to analyze data and optimize marketing campaigns through testing. |
10 Examples of Using ML in Digital Marketing
Machine learning has revolutionized digital marketing by offering a wide range of applications and possibilities. Let’s explore some examples of how machine learning can be used to enhance various aspects of marketing:
1. Predictive Analytics:
Machine learning algorithms can analyze historical data to predict future outcomes, allowing marketers to create targeted and personalized marketing campaigns.
2. Customer Segmentation:
Machine learning can divide customers into groups based on behavior and interests, enabling marketers to tailor their messaging and campaigns for better engagement and conversion rates.
3. Personalized Recommendations:
Through machine learning, marketers can provide personalized recommendations to customers, improving their overall experience and increasing the likelihood of conversions.
4. Content Optimization:
By analyzing performance data, machine learning can help marketers optimize their content strategy, ensuring that future content resonates with their target audience and drives better results.
These are just a few examples of the many ways machine learning can be leveraged in digital marketing. By harnessing the power of artificial intelligence and data analysis, marketers can supercharge their strategies and stay ahead in today’s competitive landscape.
Machine Learning Application | Benefits |
---|---|
Email Campaigns | Personalized and targeted email campaigns based on customer behavior |
Social Media Advertising | Improved campaign effectiveness through data analysis |
Chatbots | Personalized recommendations and assistance for customers |
Search Engine Optimization | Enhanced website optimization through data analytics |
A/B Testing | Optimized marketing campaigns through data-driven insights |
As the field of machine learning continues to evolve, its applications in digital marketing will only grow. Marketers who embrace this technology will be able to drive better results, improve customer experiences, and gain a competitive edge in the digital landscape.
Measuring the Impact of ML on Marketing
Machine learning has revolutionized the field of marketing, providing marketers with powerful tools to measure and optimize their strategies. The impact of machine learning on marketing can be seen in several key areas, including revenue growth, customer relationships, marketing performance, and data-driven decision-making.
When it comes to revenue growth, machine learning allows marketers to accurately measure the contributions of their marketing efforts. By analyzing data and identifying the most effective campaigns and channels, businesses can optimize their marketing strategies to drive revenue growth. With machine learning algorithms, marketers can track and attribute revenue to specific marketing activities, providing valuable insights into the return on investment.
Machine learning also plays a crucial role in building and nurturing customer relationships. By analyzing customer data, marketers can gain insights into customer preferences, behaviors, and purchase patterns. This information allows them to create personalized and targeted marketing campaigns that resonate with customers on a deeper level. By delivering relevant and tailored experiences, businesses can improve customer satisfaction, loyalty, and retention.
Furthermore, machine learning enables marketers to enhance their overall marketing performance. By leveraging data analysis and predictive modeling, marketers can optimize their campaigns, content, and messaging. Machine learning algorithms can identify trends, predict customer behavior, and make data-driven recommendations for campaign optimization. This leads to better engagement, conversion rates, and overall marketing performance.
Finally, machine learning empowers marketers to make data-driven decisions. By analyzing large datasets and extracting actionable insights, marketers can make informed choices that drive results. Machine learning algorithms can identify patterns, segment customers, and uncover hidden opportunities, enabling marketers to optimize their strategies for maximum impact.
Benefits of Using AI Tools in Marketing
AI tools offer several benefits to us as marketers. One of the key advantages is personalization. With AI, we can tailor content and messaging to individual customers, increasing their engagement and loyalty. By analyzing customer data and behavior, AI tools can help us deliver targeted and relevant content that resonates with our audience. This personalized approach can significantly improve customer satisfaction and drive conversions.
Another benefit of AI tools is increased efficiency. AI can automate repetitive tasks and streamline workflows, freeing up our time to focus on more strategic activities. By automating tasks such as data analysis and reporting, AI tools can help us make faster and more informed decisions. This not only improves efficiency but also enables us to allocate our resources more effectively.
Predictive analysis is another valuable capability of AI tools. By analyzing large amounts of data, AI algorithms can identify patterns and trends that help us forecast future outcomes. This predictive analysis can inform our marketing strategies and enable us to make data-driven decisions. By leveraging AI tools, we can stay ahead of market trends and better understand our customers’ needs and preferences.
“AI tools offer us the opportunity to create personalized experiences for our customers, improve our operational efficiency, and make informed decisions based on data-driven insights.”
In summary, the benefits of using AI tools in marketing are clear. They allow us to provide personalized experiences, increase operational efficiency, and make informed decisions based on data-driven insights. With AI, we can take our marketing efforts to the next level and drive better results for our business.
Popular AI Tools for Marketing
Artificial Intelligence (AI) tools play a crucial role in modern marketing strategies. These tools leverage the power of machine learning algorithms to enhance various aspects of marketing, from customer engagement to data analysis. In this section, we will explore some popular AI tools that are revolutionizing the marketing industry.
Chatbots
One of the most widely used AI tools in marketing is chatbots. These virtual assistants use natural language processing (NLP) algorithms to interact with customers and provide immediate support. Chatbots can handle customer queries, offer personalized recommendations, and even complete transactions. They not only save time for businesses but also enhance the customer experience by providing instant assistance 24/7.
Natural Language Processing (NLP)
Natural Language Processing is a branch of AI that focuses on understanding and interpreting human language. NLP algorithms can analyze customer feedback, social media conversations, and online reviews to gain insights into customer sentiment and preferences. This data can then be used to refine marketing strategies and create more targeted campaigns that resonate with customers.
Recommendation Engines
Recommendation engines are AI tools that analyze customer data to provide personalized recommendations. These algorithms use machine learning to understand customer behavior, preferences, and purchase history, enabling businesses to offer relevant products or services to their customers. By leveraging recommendation engines, marketers can enhance customer engagement, increase sales, and build long-lasting customer relationships.
AI tools in marketing, such as chatbots, NLP, and recommendation engines, are transforming the way businesses interact with customers and optimize their marketing efforts. By leveraging the power of machine learning algorithms, marketers can enhance personalization, improve customer experiences, and drive better results.
Machine Learning and Pricing Optimization
One of the key applications of machine learning in marketing is pricing optimization. By leveraging data analysis and predictive algorithms, businesses can determine the optimal pricing strategy for their products or services. Machine learning allows marketers to understand price elasticity, which refers to how sensitive customer demand is to changes in price. With this knowledge, businesses can adjust their pricing to maximize revenue and profitability.
Dynamic pricing is another aspect of pricing optimization that machine learning enables. With dynamic pricing, businesses can adjust prices in real-time based on various factors such as customer behavior, market trends, and competitor pricing. This flexibility allows businesses to respond quickly to changes in demand and remain competitive in a dynamic market.
For example, imagine a retail e-commerce company that sells electronics. Through machine learning algorithms, they can analyze historical sales data, customer preferences, and market trends to determine the optimal price for each product. By continuously monitoring and adapting prices based on real-time data, the company can maximize revenue and profit.
Benefits of Machine Learning in Pricing Optimization |
---|
1. Enhanced revenue and profitability |
2. Improved competitiveness |
3. Real-time price adjustments |
4. Better understanding of price elasticity |
Machine learning in pricing optimization offers several benefits. First and foremost, it allows businesses to enhance their revenue and profitability by optimizing prices based on customer behavior and market dynamics. By understanding price elasticity, businesses can identify price points that maximize demand and revenue.
Furthermore, machine learning enables businesses to stay competitive by adjusting prices in real-time. This adaptability allows businesses to respond to changes in market conditions, competitor pricing, and customer preferences effectively.
As pricing is a crucial element in a highly competitive market, machine learning empowers marketers to make data-driven decisions and optimize their pricing strategies for better results.
Machine Learning and Lead Scoring
In today’s digital marketing landscape, machine learning plays a pivotal role in lead scoring, enabling marketers to make data-driven decisions and optimize their sales strategies. Lead scoring is the process of evaluating the potential value of a lead based on various factors, such as demographics, behavior, and engagement. By leveraging machine learning algorithms, marketers can enhance the accuracy of lead scoring and focus their time and resources on the most promising leads.
Machine learning algorithms analyze vast amounts of customer data, including browsing behavior, purchase history, and interactions with marketing campaigns. This data is used to create predictive models that assign a score to each lead, indicating the likelihood of conversion. The models can identify patterns and trends that might go unnoticed by human analysts, enabling marketers to prioritize leads more effectively.
With machine learning, lead scoring becomes a dynamic and iterative process. As new data becomes available, the algorithms continuously update their models, refining their predictions and adapting to changing market conditions. This iterative approach ensures that lead scoring remains accurate and relevant over time.
By adopting machine learning for lead scoring, marketers can streamline their sales efforts, increase conversion rates, and maximize revenue. They can focus their attention on leads with the highest propensity to convert, nurturing them through targeted marketing campaigns. This data-driven approach not only improves marketing efficiency but also enhances the overall customer experience and satisfaction.
Benefits of Machine Learning in Lead Scoring |
---|
Improved Accuracy: Machine learning algorithms can identify subtle patterns and correlations in customer data, leading to more accurate lead scoring. |
Time and Resource Optimization: By prioritizing leads based on their scores, marketers can allocate their time and resources more efficiently, focusing on leads with the highest conversion potential. |
Increased Conversion Rates: By targeting the right leads with personalized and timely marketing campaigns, organizations can increase their conversion rates and drive more sales. |
Real-Time Adaptability: Machine learning models continuously learn and adapt to new data, ensuring that lead scoring remains accurate and relevant in dynamic market conditions. |
“Machine learning has transformed lead scoring, enabling marketers to focus their efforts on the most promising leads. By leveraging data-driven insights, organizations can optimize their sales strategies, increase conversion rates, and drive revenue growth.” – Marketing Tech Insights
Conclusion
In conclusion, machine learning has the potential to revolutionize marketing strategies. By leveraging the power of data analysis and artificial intelligence, marketers can gain valuable insights into consumer behavior and optimize their campaigns. Machine learning algorithms enable us to personalize content, improve efficiency, and make data-driven decisions.
With machine learning in marketing, we can enhance personalization by understanding customer preferences and delivering tailored experiences. This level of personalization leads to increased customer engagement and loyalty. Additionally, machine learning allows us to automate tasks, saving time and resources while improving decision-making through data analysis.
By embracing machine learning and incorporating it into our marketing strategies, we can stay ahead in a competitive market. With its ability to provide valuable insights and optimize campaigns, machine learning empowers us to make data-driven decisions that drive better results. The future of marketing lies in harnessing the power of machine learning and using it to revolutionize our strategies.
FAQ
What is machine learning in marketing?
Machine learning in marketing refers to the use of artificial intelligence and data analysis techniques to gain insights into consumer behavior and optimize marketing campaigns.
What are the top machine learning concepts for digital marketing?
The top machine learning concepts for digital marketing include supervised learning, unsupervised learning, neural networks, natural language processing, and reinforcement learning.
How can digital marketers use machine learning?
Digital marketers can use machine learning for predictive analytics, customer segmentation, content optimization, email marketing, social media advertising, chatbots, search engine optimization, and A/B testing.
What are the examples of using machine learning in digital marketing?
Examples of using machine learning in digital marketing include predictive analytics for personalized recommendations, customer segmentation for targeted marketing campaigns, content optimization for improved performance, email marketing for personalized campaigns, social media advertising for effective campaigns, chatbots for personalized recommendations, search engine optimization for improved website performance, and A/B testing for optimized marketing campaigns.
How does machine learning impact marketing?
Machine learning improves marketing by providing valuable insights, measuring marketing’s contributions to revenue growth and customer relationships, and enabling data-driven decisions for optimized marketing strategies.
What are the benefits of using AI tools in marketing?
AI tools in marketing offer benefits such as personalization, increased efficiency through automation and data analysis, and predictive analysis for better decision-making.
What are popular AI tools for marketing?
Popular AI tools for marketing include chatbots for customer service and support, natural language processing for understanding human language, and recommendation engines for suggesting products based on customer behavior.
How can machine learning optimize pricing strategies?
Machine learning optimizes pricing strategies by analyzing data, understanding price elasticity, and enabling dynamic pricing based on customer behavior and market trends.
How does machine learning improve lead scoring?
Machine learning improves lead scoring accuracy by analyzing customer data and predicting the likelihood of a sale, allowing marketers to prioritize resources on the most promising leads for increased sales and better conversion rates.
There are various online resources and courses available to learn more about machine learning in marketing. Some popular platforms include Coursera, Udemy, and LinkedIn Learning.
H3: Where can I learn more about machine learning in marketing?
In an era where technology intersects with every aspect of life, Maxwell bridges the gap between artificial intelligence and journalism. As a writer and AI expert, he explores the implications of emerging technologies on society, economy, and culture. Maxwell’s expertise allows Press Report to offer forward-thinking insights into the future of AI, making complex topics accessible and engaging for our audience.
AI in Education
Unlocking Possibilities: Can AI Really Learn?
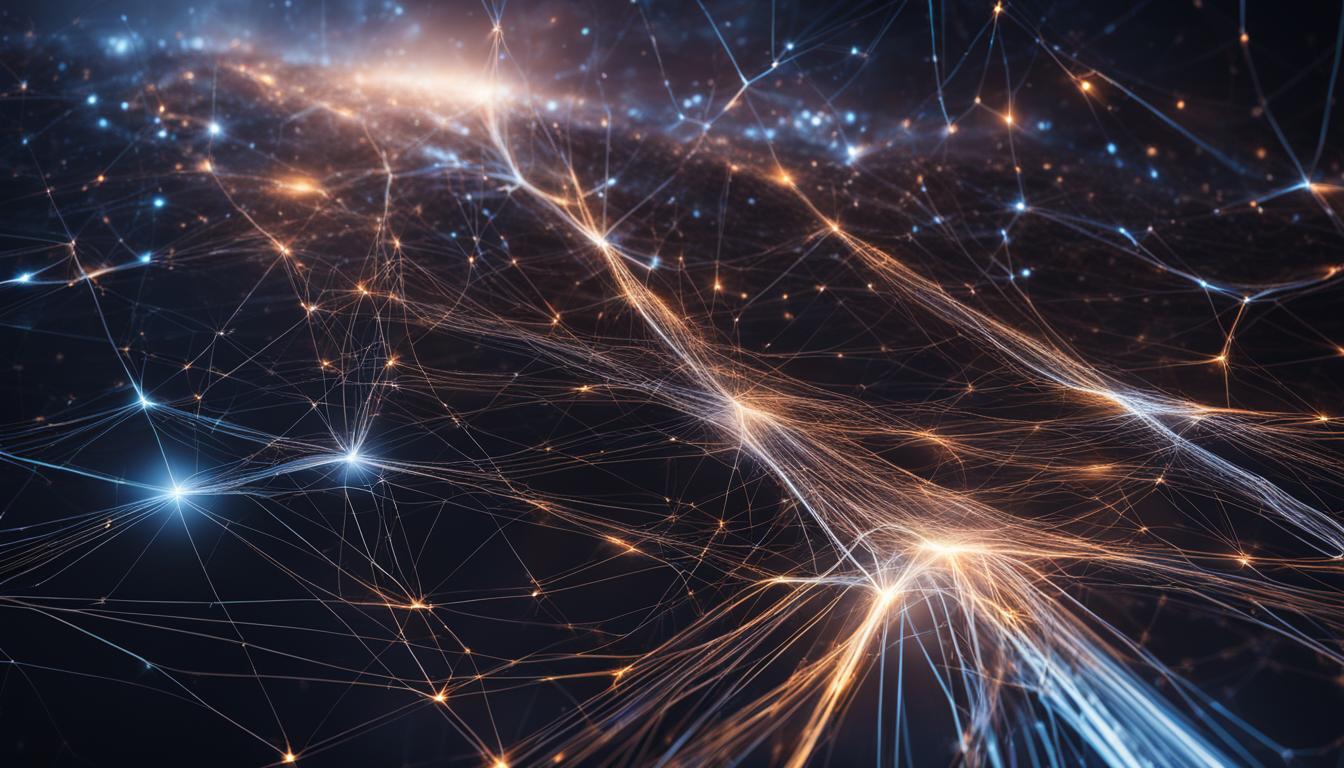
Welcome to our spotlight on AI learning! In this section, we’re exploring the fascinating world of artificial intelligence and its educational prowess. We’re going to delve into the discoveries and research showcased at the autumn meeting of the Stanford Institute for Human-Centered Artificial Intelligence. This gathering brought together experts from various fields to debate the progress of AI algorithms in achieving more natural reasoning, the capability of robots to transfer their learning to various activities, and the influence of a child’s learning method on AI evolution. Join us as we uncover the possibilities of AI in education!
Key Takeaways:
- AI learning was a prominent topic at the Stanford fall conference on human-centered artificial intelligence.
- DeepMind’s research on deep reinforcement learning showcases the power of AI neural networks.
- Understanding the brain and cognition can lead to better AI models.
- Robots can learn to generalize their knowledge through visual demonstrations.
- Machine learning is driving advancements across various industries.
The Power of Deep Reinforcement
Deep reinforcement learning is revolutionizing the field of artificial intelligence (AI). By combining deep neural networks with the principles of reinforcement learning, researchers are unlocking new capabilities and achieving remarkable results. Matthew Botvinick, director of neuroscience research for DeepMind, discussed the power of deep reinforcement learning in AI applications.
DeepMind, a leading AI research lab, has leveraged deep reinforcement learning to train machines to play classic Atari games at superhuman levels. Building on this success, they expanded their approach to tackle more complex games like StarCraft and Go, and even ventured into the realm of multi-agent games like Capture the Flag. Through deep reinforcement learning, these AI systems are able to learn and improve their strategies, making better predictions and achieving exceptional performance.
One of the key insights driving these advancements is the understanding of dopamine-based reinforcement learning, which is inspired by how the human brain processes rewards. DeepMind researchers are utilizing this knowledge to train AI neural networks, allowing the systems to make more accurate predictions. By incorporating insights from the human brain, these AI models are becoming more intelligent and capable of solving complex problems.
“Our research has shown that deep reinforcement learning can bring AI to new levels of performance and effectiveness,” says Botvinick. “By combining the power of deep neural networks with the principles of reinforcement learning, we are unlocking the true potential of AI.”
The Future of AI Predictions
With deep reinforcement learning, the possibilities for AI applications are vast. From gaming to robotics, this approach is allowing machines to learn and adapt in dynamic and challenging environments. As researchers continue to push the boundaries of deep reinforcement learning, we can expect further breakthroughs in AI predictions and problem-solving capabilities.
Applications | Benefits |
---|---|
Gaming | Superhuman performance |
Robotics | Adaptive learning in complex environments |
Finance | Improved risk management and investment strategies |
Healthcare | Enhanced diagnosis and treatment planning |
Deep reinforcement learning is propelling AI into new frontiers, enabling machines to learn and make predictions in ways that were once thought impossible. The integration of deep neural networks and reinforcement learning principles holds tremendous potential for solving complex problems and driving innovation across various industries. As researchers continue to advance the field, we can expect AI predictions to become increasingly accurate and powerful.
A Two-Way Modeling Approach
In our quest to create more advanced AI systems, we have embarked on a two-way modeling approach that aims to foster a better understanding of the brain and cognition, while also leveraging AI to enhance our knowledge of human intelligence. By bridging the gap between cognitive science and AI, we are unlocking new possibilities for creating more intuitive and intelligent machines.
Modeling the Human Visual System
One key aspect of our approach involves modeling the human visual system using AI. By developing optimized models that mimic how the brain processes visual information, we can gain valuable insights into how our own visual system functions. This allows us to create AI systems that can better perceive and interpret visual stimuli, leading to advancements in fields such as computer vision and object recognition.
Furthermore, by comparing our AI models with actual brain functioning, we can identify areas where our understanding may be incomplete or where our models can be improved. This iterative process of learning from both AI and the brain helps us refine our models and gain a deeper understanding of how the human visual system works.
Applying Insights from Cognitive Science
In addition to modeling the brain, we also draw inspiration from cognitive science to develop AI models that exhibit curiosity and intuitive physics. By studying how infants learn and interact with their environment, we can apply these insights to our AI systems, enabling them to learn and adapt in a more human-like manner.
By incorporating cognitive science principles into our AI models, we are constantly pushing the boundaries of what machines can do. AI systems that embody curiosity and intuitive physics have the potential to revolutionize fields such as robotics, where machines can better understand and navigate the physical world, making them more capable and adaptable.
By adopting a two-way modeling approach, we are not only advancing the field of AI but also deepening our understanding of the human mind. As we continue to explore the possibilities that arise from this synergy between AI and cognitive science, we are paving the way for more intelligent and intuitive machines that can truly revolutionize the way we live and work.
Improving Generalization with General Training
In our quest to develop more intelligent AI systems, one crucial challenge we face is improving the generalization capabilities of these machines. While AI algorithms have shown impressive learning abilities in specific tasks, they often struggle to apply the acquired knowledge to new and unfamiliar scenarios. To address this issue, our team, led by Stanford assistant professor Chelsea Finn, is focused on training AI applications to generalize their learning, much like humans do.
Our research has shown that providing robots with visual demonstrations can greatly enhance their ability to learn and generalize. By observing real-world examples, robots can extract valuable information and apply it to similar situations, improving their adaptability and problem-solving skills. This approach has proven to be highly effective, resulting in faster and more generalized learning.
As part of our efforts to advance the field, we are also working on creating a comprehensive database called RoboNet. This database will facilitate the sharing of learning-related videos across institutions, allowing robots to learn from a wide range of experiences and perspectives. By leveraging the collective knowledge of AI researchers and practitioners, we hope to accelerate the development of robust and intelligent AI systems.
Benefits of Visual Demonstrations for Robots |
---|
Enhanced learning abilities |
Improved generalization skills |
Increased adaptability |
Accelerated problem-solving capabilities |
By combining visual demonstrations and collaborative knowledge sharing, we believe that we can empower AI systems to learn and generalize in a way that closely resembles human learning. This is a significant step forward in the development of AI technologies that can adapt and thrive in complex and dynamic environments.
Unlocking the Potential of General Training
General training, supported by visual demonstrations and collaborative learning, holds immense potential for the future of AI. By enabling robots to learn from a vast array of experiences and perspectives, we can equip them with the skills necessary to navigate real-world scenarios with confidence and efficacy.
As our research continues, we are excited to see the impact of improved generalization on AI applications across various industries. From manufacturing and healthcare to transportation and entertainment, the ability to learn and generalize will revolutionize the capabilities of AI systems, opening up new possibilities and transforming the way we interact with technology.
As we strive to unlock the full potential of AI, it is essential to prioritize efforts that enable machines to learn and generalize. By doing so, we can create AI systems that are not only highly proficient in specific tasks but also capable of adapting and thriving in the face of new challenges.
Toward Scalable Commonsense Intelligence
In our quest to bridge the gap between human and machine understanding, we are continually exploring ways to model how human intelligence works. By understanding how the brain works, we can develop AI systems that possess a deeper level of commonsense intelligence. Our research team at the University of Washington has made significant strides in this area, with the development of the Visual Comet system.
The Visual Comet system utilizes natural-language descriptions to enable AI models to reason about everyday life. With the ability to understand unfamiliar examples, these models can navigate complex scenarios and make informed decisions. By modeling human intelligence, we aim to enhance AI’s comprehension of the world, enabling it to interact more seamlessly with humans and tackle real-world problems.
Another researcher, Aude Oliva, co-director of the MIT-IBM Watson AI Lab, shares our objective of advancing commonsense intelligence. Leveraging basic neuroscience knowledge, her team is also working on developing AI models that can understand and reason with everyday concepts. By drawing insights from the human brain, we can unlock new possibilities for AI and propel the field forward.
Modeling human intelligence is a crucial step towards creating AI systems that can truly understand and navigate the complexity of the world. When we empower AI with the ability to reason and comprehend unfamiliar examples, we open up a realm of possibilities for improved decision-making and problem-solving.
The Path to Advancement
As we delve deeper into modeling human intelligence and understanding how the brain works, we are pushing the boundaries of what AI can achieve. Our research not only enables AI to understand and reason with unfamiliar examples but also fosters a deeper connection between human and machine understanding. By leveraging the insights from cognitive science and neuroscience, we are paving the way for scalable commonsense intelligence that can address real-world challenges.
Through ongoing research and collaboration, we remain committed to unlocking the potential of AI and pushing the boundaries of what is possible. By modeling human intelligence and expanding our knowledge of how the brain works, we are shaping the future of AI and opening up a world of possibilities.
Advantages of Modeling Human Intelligence | Challenges of Modeling Human Intelligence |
---|---|
|
|
Learning While Protecting Privacy
When it comes to the advancement of artificial intelligence (AI), data privacy has become a paramount concern. As we continue to delve deeper into the realms of deep learning and data-driven algorithms, it is crucial to find ways to protect sensitive information while still enabling AI to learn and evolve. One promising solution is automated data encryption.
Automated data encryption allows us to secure personal data without hindering AI model training and testing. One notable system in this field is InstaHide, developed by Sanjeev Arora, a Princeton professor of computer science. InstaHide encrypts images and text-based data, providing high accuracy and efficiency while safeguarding individual-level information. This innovation opens doors for the application of AI in various domains, including medicine and self-driving cars, where data privacy is of utmost importance.
As we navigate the complex landscape of AI, ensuring data privacy for AI applications must remain a top priority. By incorporating automated data encryption systems like InstaHide, we can strike a balance between the potential of AI and protecting the privacy of individuals. This approach not only safeguards sensitive information but also enables further advancements in AI and deep learning, paving the way for a more secure and responsible future.
Automated Data Encryption: Mitigating Risks, Unlocking Potential
Data privacy is a critical concern in the age of AI, and automated data encryption offers a viable solution. With innovative systems like InstaHide, we can harness the power of deep learning while ensuring that personal data remains safe and secure. By adopting these encryption techniques, we can unlock new possibilities and drive advancements in AI without compromising privacy.
Understanding Machine Learning
Machine learning is a fundamental aspect of artificial intelligence that enables computers to learn and make predictions without explicit programming. It is a rapidly advancing field that offers tremendous potential in various industries. Businesses across sectors are embracing machine learning to unlock new value, improve efficiency, and gain a competitive advantage.
Machine learning finds applications in different industries, ranging from manufacturing and retail to banking and even bakeries. In manufacturing, machine learning algorithms can be used to optimize production processes, identify potential defects, and predict machine failures. Retail companies utilize machine learning to personalize customer experiences, enhance inventory management, and improve demand forecasting.
Financial institutions leverage machine learning to detect fraud, assess credit risks, and develop algorithmic trading strategies. Even bakeries can benefit from machine learning by analyzing customer preferences, optimizing ingredient proportions, and predicting demand patterns. The potential applications of machine learning are vast and continue to expand as technology advances.
By harnessing the power of machine learning, businesses can gain valuable insights from data, automate decision-making processes, and improve overall operational efficiency. As technology continues to evolve, machine learning will undoubtedly play a critical role in shaping the future of various industries.
Machine Learning Applications in Different Industries:
Industry | Machine Learning Applications |
---|---|
Manufacturing | Optimizing production processes, identifying defects, predicting machine failures |
Retail | Personalizing customer experiences, enhancing inventory management, improving demand forecasting |
Banking | Detecting fraud, assessing credit risks, developing algorithmic trading strategies |
Bakeries | Analyzing customer preferences, optimizing ingredient proportions, predicting demand patterns |
The Basics of Machine Learning
Machine learning is a fundamental concept in the field of artificial intelligence, encompassing various techniques that allow computers to learn and make predictions or decisions without explicit programming. It can be broadly categorized into three subcategories: supervised, unsupervised, and reinforcement learning.
Supervised Machine Learning
In supervised learning, models are trained using labeled datasets, where the correct answers or outputs are provided. The model learns to make predictions or classify new data based on the patterns and relationships it identifies in the training data. This approach is commonly used for tasks such as image recognition, sentiment analysis, and spam filtering.
Unsupervised Machine Learning
Unsupervised learning involves training models with unlabeled datasets, where the model needs to discover patterns or structures on its own. This type of learning is useful for tasks like clustering, anomaly detection, and dimensionality reduction. By identifying hidden patterns and relationships, unsupervised learning can provide valuable insights and help uncover new knowledge.
Reinforcement Machine Learning
Reinforcement learning operates on the concept of training models through trial and error. It involves an agent interacting with an environment and learning to maximize rewards or minimize penalties. The model explores different actions and observes the outcomes, adjusting its behavior to achieve the desired goal. Reinforcement learning is widely used in applications such as autonomous driving, game-playing agents, and recommendation systems.
By understanding the basics of supervised, unsupervised, and reinforcement learning, we can gain insights into the inner workings of machine learning algorithms and their applications across various industries. Whether it’s predicting customer preferences, identifying patterns in complex data, or training intelligent agents, machine learning continues to revolutionize the way we solve problems and advance AI technology.
Machine Learning and AI Subfields
Machine learning is a key component of various AI subfields, enabling them to achieve remarkable advancements and practical applications. Let’s explore three important subfields that are closely associated with machine learning: natural language processing, neural networks, and deep learning.
Natural Language Processing
Natural language processing (NLP) focuses on enabling machines to understand, interpret, and respond to human language. It involves tasks like speech recognition, sentiment analysis, machine translation, and chatbot development. NLP algorithms leverage machine learning techniques to process and analyze vast amounts of textual data, enabling machines to comprehend and generate human language effectively.
Neural Networks
Neural networks are a class of machine learning algorithms inspired by the structure and functioning of the human brain. These networks consist of interconnected layers of artificial neurons that process and analyze data to recognize patterns, make predictions, and perform complex tasks. Neural networks have been widely utilized in computer vision, natural language processing, and other domains where the ability to recognize and understand complex patterns is crucial.
Deep Learning
Deep learning is a subset of machine learning that utilizes neural networks with multiple layers to process and analyze large volumes of data. It has revolutionized AI by enabling machines to automatically learn complex representations and hierarchies of information. Deep learning has been instrumental in numerous applications, ranging from image and speech recognition to autonomous driving and healthcare diagnostics.
Overall, machine learning plays a vital role in advancing AI subfields such as natural language processing, neural networks, and deep learning. These subfields, in turn, contribute to the development of powerful applications that enhance our understanding of language, enable intelligent decision-making, and improve the overall capabilities of AI systems.
Conclusion
Machine learning has become an essential part of AI, revolutionizing industries across the board. From manufacturing to retail, finance to healthcare, machine learning is unlocking new value and enhancing efficiency. As technology continues to evolve, understanding the basics of machine learning and its subfields is crucial for individuals in any sector.
But it’s not just about the technical aspects. We also need to consider the social, societal, and ethical implications of machine learning. Responsible and beneficial use of this technology requires us to address issues such as data privacy, algorithmic bias, and transparency. By proactively addressing these concerns, we can ensure that machine learning is harnessed for the collective benefit of society.
As we move forward, it is important to stay informed about the latest developments in machine learning and AI. Advancements in natural language processing, neural networks, and deep learning are constantly pushing the boundaries of what is possible. By staying up-to-date and participating in the ongoing conversation, we can contribute to shaping the future of machine learning and AI in a positive and impactful way.
FAQ
Can AI really learn?
Yes, AI can learn through machine learning algorithms that enable computers to learn from data without explicit programming.
What is deep reinforcement learning?
Deep reinforcement learning is a type of AI application that uses neural networks and dopamine-based reinforcement learning to train machines to make better predictions and achieve superhuman performance in tasks like playing games.
How does AI understand the brain?
AI systems can better understand the brain by modeling its functioning and comparing it with AI models. This two-way modeling approach helps in gaining insights into cognition and developing AI models that embody curiosity and intuitive physics.
How do robots learn to generalize?
Robots learn to generalize by receiving visual demonstrations, which enable them to learn faster and generalize their learning, similar to how humans learn.
How can AI bridge the gap between human and machine understanding?
By modeling how human intelligence works and leveraging insights from neuroscience, AI models can reason about everyday life and develop commonsense intelligence.
How can data privacy be maintained in deep learning?
Data privacy in deep learning can be maintained through automated data encryption systems like InstaHide, which encrypt images and text-based data, enabling high accuracy and efficiency while protecting individual-level data.
What is machine learning and where is it used?
Machine learning is a subfield of AI that enables computers to learn from data without explicit programming. It is used in various industries, including manufacturing, retail, banking, and more, to unlock new value and improve efficiency.
What are the different types of machine learning?
The different types of machine learning are supervised learning, where models are trained with labeled data; unsupervised learning, which looks for patterns in unlabeled data; and reinforcement learning, where models learn through trial and error using a reward system.
What are the associated subfields of AI with machine learning?
Natural language processing enables machines to understand and respond to human language, while neural networks are a class of machine learning algorithms modeled on the human brain. Deep learning utilizes neural networks with multiple layers to process extensive amounts of data and determine patterns.
What is the role of machine learning in AI?
Machine learning is an essential part of AI, driving advancements in various industries. Understanding the basics of machine learning and its subfields is crucial for individuals across all sectors to harness its potential while considering social, societal, and ethical implications.
Source Links
-
AI in Legal2 weeks ago
Revolutionizing Justice: How AI is Transforming the Legal Landscape
-
AI Entertainment1 month ago
OpenAI Releases SORA: Breakthrough AI Platform
-
AI in Business2 weeks ago
AI: The Powerhouse Driving Tomorrow’s Industries
-
AI in Legal2 weeks ago
From Briefs to Bytes: The Impact of AI on Legal Documentation
-
AI in Business2 weeks ago
Secure Your Future with AI Data Privacy Assurance
-
AI in Medicine2 weeks ago
Revolutionizing the Future: AI in Healthcare and Medicine
-
AI Security2 weeks ago
Unlock the Power of Robust AI Security for Your Business
-
AI in Business2 weeks ago
AI’s Effect on Employment: Trends & Insights